Breaking Data Silos: AI-Ready Data Strategies with Nishith Trivedi, Enterprise Data Governance and Global MDM Lead at Pfizer
Download MP3Data Hurdles: Nishith from Pfizer
===
Chris Detzel: [00:00:00] ~Hello, Data Enthusiasts.~ This is Chris Detzel and I'm Michael Burke. Welcome to Data Hurdles.~ We are your gateway into the intricate world of data, or AI, machine learning, big data, and social justice intersect. Expect thought provoking discussions, captivating stories, and insights from experts all across the industries as we explore the unexpected ways data impacts our lives.~
~So get ready to be informed. Inspired and excited about the future of data.~ Let's conquer these~ data ~hurdles together.
Welcome to another data hurdles. I'm Chris Detzel and
Mike: I'm Michael Burke. How you doing, Chris?
Chris Detzel: Pretty good, man. How about you?
Mike: Good, good. It's beautiful day outside. It's Friday. I'm happy it's been an incredibly busy week for me, so I'm happy to have a nice weekend to unwind a little bit.
How about yourself? Do you have any running planned?
Chris Detzel: Yeah, probably run about 20 miles this weekend, one on Saturday, Sunday, and it's very nice here right now, but we are in the wind alert, like this is going to be potential fires here in Dallas or Texas, but we'll see, but lots of wind today, I think so.
Mike: Oh, that's really exciting.
Chris Detzel: Today we have special guest Nishit Trivedi. And Nishit is from Pfizer and he's the enterprise data governance and global MDM lead. How are you? Pretty good. How are you [00:01:00] guys doing? It's good. It's been a while. Yeah. Yeah. I was really excited about that. I think the last time
Mike: we saw each other was at the round tables, right?
The executive round tables at tio? Maybe? I think it's been a,
Nishith Trivedi: yeah, I think the one was it in Dallas or
Mike: Yeah. Yeah, the Dallas sync up. That's right. Chris Zel. You put that together,
Chris Detzel: yeah I did many of those things. You mean Danny.
Mike: Yeah.
Chris Detzel: Yeah. And I met Chris at the Gardner
Nishith Trivedi: DNA in Orlando as well.
Chris Detzel: That's right. That's right. And I've seen you several times last year at the data what is it? The data conference that Reltio puts on? Yes. Yeah. So it was fun. Yeah, it's been great working with you over the years and certainly, We're excited to have you on. So today we want to dive into who you are, what you're doing over at Pfizer and just get your journey.
So let's dive in and tell us a little bit about yourself, what you do at Pfizer, and we'll go from there, if that's fair.
Nishith Trivedi: Sure, happy to. So again, my name is Nishit Trivedi. As you mentioned, Enterprise Data Governance and Master [00:02:00] Data Management at Pfizer. ~ ~We are part of the ~ ~AI data and analytics practice as part of digital.
So we're basically a data horizontal. We cover all verticals. So I work with, not just commercial, but everything from, our supply chain, manufacturing, finance, legal, HR, R& D, et cetera.
Mike: That's a lot of verticals.
Nishith Trivedi: Yeah, it's it's fun. I came from a commercial world, so there's a lot of new challenging areas to work with.
And as part of, my journey, started out at Pfizer in a master data management. I came from consulting. My background has been traditional data management, so BI, data lakes, data warehouses, MDM. And so I joined as the MDM lead. And then, yeah. In 2023, March 2023, so essentially two years ago, I got a expanded opportunity to take also over data governance, enterprise data governance, and what that means, data governance means different things to different people.
But from our perspective, it's the Calibra Alation platform for data governance. It's ontologies, knowledge graphs, [00:03:00] reference data management, metadata management, data stewardship, and then data governance councils. So it's a lot of stuff and, considering various verticals, we have a lot of different initiatives right in the pipeline right now.
It's an exciting space.
Mike: And how did you get into the data space? I know it's such a different journey for everybody. Was it something that you had planned like since college or did you stumble upon it? No, I think
Nishith Trivedi: I joined college originally as a chemical engineer, but I realized very quickly that, I didn't want to spend my life in factories, like in chemical factories over.
So I did shift over to management information systems in college. And so I graduated with that. I went into consulting. And so essentially what I learned in college and what I learned, in consulting was really just data management, helping, in consulting, I worked across.
Various industries, right? So I was in D. C. Metro area for a while. So a lot of federal government, U. S. Postal, U. S. Navy, U. S. D. A. As well as large, fortune 100 clients parts of the [00:04:00] world of Verizon Wireless. So a lot of, data management and then got into B. I. Because once you get into data lakes, their warehouses, you get into B.
I. And once you get into that right, master data management, I started, I think, in Late 2014 or 2015 working with Reltio, a little bit with Informatica as well, but I have a very strong relation with Reltio, which is when I met both of you and then that translated to, jumping over to industry right in 2021 ~ ~when I got Opportunity Advisor.
Mike: I can't tell you how common it is that we see this life cycle of people that have been in the data space forever. They started off in another industry. They had this kind of golden view of seeing like the customer facing data sets in the space. And slowly they work through that pipeline until they end up in the depths of the serving layer.
I feel like that's where all the experts. reside in these systems as they end up owning large enterprise systems that feed the rest of the business. Huge amount of complexity and orchestration associated with those platforms. Really interesting story. And [00:05:00] do you think that if you looked back on today's self from your younger years, would you have seen yourself here ~ ~in this role, in the MDM space?
Chris Detzel: No, he's a chemical engineer. No way.
Nishith Trivedi: I don't know exactly, but I think, definitely like it's a very exciting space to be. I, I'm a data nerd. I do enjoy just solving problems right coming from consulting is all about solving problems, giving tangible results.
I never wanted to be just pure. Strategy consultant where I'm giving decks and recommendations walking away. So always implementing and making sure we see through to the business outcome. So I do appreciate that. And then I had to say moving from just at Pfizer, only the MDM to now owning various components.
And, when I joined, to be honest like the team, the catalog team the elation team and the Caliber team are two different teams at the point in time. Now we combine them. And then the The, the reference management and the MDM team did work together. So there was some synergy, but for the most part, like the ontology team work separately, knowledge graph team work separately the catalog team and MDM team, like they probably don't even know each other.
[00:06:00] So now my challenge has been, Hey, how do I build that team culture? And then more importantly, how do I make sure that these capabilities, right? MDM reference data, metadata, ontology, knowledge, graph, catalog They're basically coming together and the whole is better than the sum of the parts, right?
So that's been one of our, challenges, but it's also the exciting part. I had to say right now, right? Like now that, I've gone deeper into each of these areas, we do see many different patterns, right? You can see, for example, now specifically the gen AI and the agentic AI stuff, right?
Like you really need aI ready data. And what does AI ready data mean, right? Obviously from MDM the interconnectivity, right? Like all these different data sets and different data lakes need to be interconnected for structured data. Now for unstructured data,
Chris Detzel: you don't
Nishith Trivedi: have MDM, right? That's where knowledge graphs come in.
That's where ontology is coming out. We have scientific articles. We have lab notes from bench scientists. We have clinical trial documents, right? Coming out of, principal investigators or their, handwritten stuff or, lab written stuff. We have lab nodes, we have lab EMR, EHR kind of data, and being able to [00:07:00] stitch together multiple unstructured data together using, ontologies.
We basically have various kind of semantic technologies, like named entity recognition, optical character recognition, voice audio. ~ ~Translation to kind of text and applying metadata tags to that and then applying taking that metadata tags putting into knowledge graph and then being able to do graph rag and then your LLM can call that right that you really need to combine a whole bunch of different technologies together and that's the challenge and that's the exciting part right now is, when I was just doing MDM, it was great because we supported, enterprise MDM, marketing medical, but now we're truly saying, hey, it's not just for Structured, transaction data, but now it's unstructured data.
We're helping with content generation. We helping with knowledge mining. So it's really opened up into the opportunities.
Mike: I don't know if I gave you this update before the call, but I've actually switched to Databricks if you know the company. Of course. Yeah. We're,
Nishith Trivedi: yeah we're one of our r and d and clinical is a Databricks shop, so we work closely with that.
And we're right now doing a POC to connect the Databricks Unity catalog to our [00:08:00] Collibra, so that way we have one amazing, one catalog that rules 'em all from a marketplace perspective. And I'm at Zoom Info,
Chris Detzel: so I don't know if you guys think about third party data in that way, you work with sales folks?
I'm just, I wouldn't think that you would a ton, right? With whom? Like sales folks, marketing folks, and all that kind of stuff? No, that, that's like
Nishith Trivedi: bread and butter. I came from ZS, from consulting, which is a sales and marketing consulting company, that's my expertise is like sales and marketing, commercial and MDM, of course, supports, right?
Like from a customer perspective, really supported, everything from sales reporting to IC to targeting alignment, ERM, medical CRM, sales CRM, marketing messaging. So we do that currently at Pfizer, right? There's a big push to modernize our marketing technology, Martec stacks that we're bringing in.
CDP technology, so we can get better at, like when we have website visitors, right? Like not everybody in fact I think like it's a, in a single digits, like the number of known people who go to a Pfizer website, right? Whether it's a Pfizer. com, Pfizer. com. [00:09:00] Or it's a brand website or even a therapeutic area website, like most people could go in there, whether you're a patient, caregiver, or it's a HCP or, some healthcare professional, you go there, you look at data, you download it, only in certain, exceptions, I would say, or certain like things like sample ordering, you actually need to register to be able to run a transaction, but there's a lot of stuff you can go there without registering.
So really, I think it's single digits where we have known visitors to the websites. And not using CDP, they want to basically increase that 3x, 5x, whatever the percentage is to have greater help. And so from an MDM perspective, we're partnering with the CDP team and the marketing team of how do we help them go from the anonymous to partially identified to fully identified.
And then from fully identified to that customer 360 view marketing campaigns.
Mike: Yeah, it's so interesting to see companies, especially like Pfizer, who is been traditionally more of a provider of things than a consumer or customer facing [00:10:00] technology starting to pull back and realize that value, right?
Of if they have this customer 360 view, what can they do? What can they learn? How can they make the experience better? Talking about large language models. I know you mentioned rag and some of the development you're doing. How are you? How are you thinking about rag in the long term across Pfizer?
And especially from a servability perspective, the architecture is so much different than what you would see in your traditional structured data systems. How are you guys planning the long term? And again, I don't want to ask you too many questions about specifics, but really interested to understand your vision and your take on the future.
Nishith Trivedi: Sure. Of course, it's evolving technology, right? And as at Gen AI, every two weeks, there's a deep seek and then, et cetera, et cetera. It's fast. I would say that probably the earlier iterations of RAG at Pfizer have been more vector RAG. And I would say that of course, when the LLM came out with chat, GBD, copilot, et cetera, like probably Q4, 2023 and last [00:11:00] year.
From the executive level, right? The CEO basically told all the C level people to, have, goals, right? And making sure like Jenny is front and center, for their goals and departments, right? And finding use cases. So at the highest level that Pfizer, right? That's been a priority of, how do we make AI their my, my leadership team, right?
Which used to be senior VP of Data analytics is now a chief AI officer, right? So it's a named chief officer. Yeah, an analytics officer. So even from a rule perspective, right? Like they brought in people who have that. And I think specifically to your question, right? The first generation was, hey, let's use LLM and LLM, not that it's a silver bullet, but hey let LLM do a lot of the rag stuff for you.
And when we got to, Yeah. Complex, clinical trial data, research data, omics, proteomics, genomics, stuff of that. You can't really just, have your graph, or your, yeah. The LLM expect that it knows all the scientific stuff. It has all the knowledge about, various genomes and gene sequences and gene assays and stuff of that.
So now we're [00:12:00] saying, Hey we really need to bring more context to LLM so that, hey, you don't want to hallucinate and be right. Like you want, to be, better integrated with your terminology, right? Pfizer terminology or industry terminology. My team owns the ontology and the platform called Sentry.
And so we have, all sorts of public plus internal Pfizer or, public with the extended curated and extended curation of the, things like gene ontology, protein ontology, things of that Pfizer product and Pfizer departments, et cetera. We have a technology called for named entity recognition and essentially think of it as let's say you have a document and I'll use a very generic term, right?
Let's say the document has the word bat, and that could mean a baseball bat or a sports or that could mean, an animal, right? Like the bird bat, right? Like when you read a document, how does your, how does your, Jenny, I know what context is word bad was. You can say I have this metadata tackle bad in this document, but what is the context, right?
So this is where the vocabulary and the name [00:13:00] entity recognition will have rules on top of that. And the rules are, things like, hey, the word bad is there. And then to the left and right of that, if you have the word baseball sports, like field pitching, right? Like then, okay, this is related to sports and you basically go down this semantic path of sports ontology.
If you have things like, nighttime and, cave and echo and stuff like that, okay, that's the bat and there's animal, bird ontology, right? That kind of stuff, right? Like that context behind looking at unstructured data, applying the right metadata tags, and then ability to then take that into a knowledge graph, right?
Where you say, okay, using, we have these documents, but, we basically. generating these specific ontology specific nodes. And then you can now, by looking at the different metadata tags, you can say, here's the inferred relationship between these nodes. Now you're building a much more richer graph, which will help you with your graph rack.
So I think the evolution to your question is, Hey, we went from vector graph to now going towards more graph rank.
Mike: That's amazing. Yeah. And it's so interesting in these areas of technology [00:14:00] and for folks that aren't as technical on the call, your LLMs and your chat GPTs, they have generalized context that works pretty well and answering a large array of questions.
But once you get to these edges these edges of research and science, ~ ~they do okay, but they won't understand the specifics of the questions that you're asking. And if they return any answers at all, they'll be pretty generic. And so what Nishet is talking about is. By loading all of this additional research that they've structured in a way that's meaningful and contextualized, the machine learning model can give responses that cite specific research related to that problem.
And this is where I think we're seeing a lot of evolution in the model of experts stage of LLM, where we have specialized models that are able to answer these questions better than any generalized model like a chat GPT. ~ ~How are you thinking about, and this is something I'm curious about we'll pull back a little bit on the technical, even though I could probably talk to you for hours about this
Chris Detzel: quick question because I'm a little [00:15:00] curious around, you mentioned, we got to try to stop the hallucinations.
I feel like every LLM model hallucinates. Is there. Something that you could put in to say, don't speculate, don't do any of these things, like just use what the data shows or what we put into this.
Nishith Trivedi: And to be fully honest like we, I work on the foundational data, right?
So we're supporting our DNA COE, which is actually, and we have, our, generalized LLM platforms. We use all of them Claude? Bar, et cetera.
Chris Detzel: Yeah.
Nishith Trivedi: There's obviously the prompt engineering, there's, ability to put parameters of how much to hallucinate. And, if you're creating marketing content and you say, Hey I want some more flowery language, right? That's hallucinate a little bit more, be more creative if it's Yeah. This document generation as for FDA, documentation like FDA filings for yeah. new drug, like you don't want any hallucination at all, right?
So you can put some parameters there. But I think, as Mike was saying ability to have context using like better metadata and better able to say if you ask a question of your data, you say, Hey can you tell me me like the various scientific journals, as well as clinical [00:16:00] studies, which have, Gene ontology for X, Y, Z gene, for this indication, then like ability to say, Hey, like this indication, right?
Probably includes other synonyms, right? Or like this protein, there's a different synonym of that, right? If you just have your pure like rag images or pure vector, you may just only know that term. But now with ontologies, we're saying, Hey, this term is associated with this term is associated with this term, right?
And it just spreads out. And then also when it gives you a return, it'll give you the link saying, Hey, these are the 10 documents, which we looked through. You can click on it and you can verify yourself that, okay. Got it. Just anything context specific.
Mike: Yeah. Also, these new kind of multi model approaches, they do things that are really interesting.
There's reinforcement learning in them. So if 10 other people have been searching in a similar area and returned results that they liked and didn't mark those results as poor, then the model will get better and it will start recommending more in that area of research, right? When you ask a similar question.
And then in addition to that, [00:17:00] in the multi model approaches, there's now models that do things like fact checking of the responses from the specific expert that returned that information. So it will, need a citation, and it will check that the citation is relevant with the synonyms and the reference data.
So there's these really cool intricate ways that You know, models will always hallucinate to some degree, but that you can significantly limit those hallucinations and returning poor data. Got it. And it's becoming so powerful. It's becoming more of a network of model of experts. Like you would see in a company when you try to solve a problem, you don't just ask one person, you ask 10 people to collaborate and get back to you with a real answer.
Nishith Trivedi: And now it's basically a network of agents, right? With different models. That's yeah, you can stumble upon a every second linkedin article about agent decay. So that's the new buzzword this year.
Mike: Let's go ahead. Just one more thing because I think this is interesting. That's all we can probably do a podcast just on this at some point.
But in the agentic AI space, one of the [00:18:00] newest thing that's coming out is this ability to test feedback from models. So like you have 100, 000 people using this expert model. And all of a sudden you're getting feedback of what works and what doesn't. And you might need to change models. You might need to swap chat GPT for cloud under the scenes as like your main system.
How do you measure all the accuracy that's shifted in your environment? What happens when the models update? This whole framework almost becomes a more complex data problem. If you think of like your model, it's just another giant database. You're shifting systems. And so part of this agentic multi model shift in the landscape right now is.
Is really focused around how can we create a better platform that controls and monitors and measures the accuracy and reliability of these systems. And that is so important. I think that is like coming. It's net new. Only a few companies are really adopting it, but it is something that in the next few years you're going to see every company working on one of these [00:19:00] platforms that helps measure data and accuracy of models this way.
Chris Detzel: So interesting. How do you think about it? You mentioned that it's the big buzzword is AI agents. What is that? Is that kind of these? very kind of what Michael is talking about, expert type of models that are just specific experts in certain things. Or how do you see
Nishith Trivedi: that today?
I think, there's obviously there's going to be all flavors of them, right? I don't think there's, limited. So I think There's going to be each of our software vendors, right? So for example, Excel data, right? Like I mentioned, I spoke to Ramon and they announced a couple of weeks ago that Excel data has, this autonomous kind of agent kind of architecture coming.
I'm sure Relative has it. I'm sure like ServiceNow is big into it. Salesforce really pushed like in agents, right? And these are like, we are ServiceNow shop, we're Salesforce shop, right? So we'll have individual ad, our SI vendors, right? The Accenture's and, yes, the world like they have their own kind of frameworks.
And our job really is to say, Hey, how do we bring all of this together? And how do we [00:20:00] build, cohesive agent framework, which will help us in multiple areas, right? So obviously, from a data quality perspective we can have Excel data agent, and it could be looking at various pipelines.
Versus it could be a data stewardship agent for MDM data quality, or versus we could have a, right now we're trying to build, automated metadata for Colibra and Elation and, data catalogs Unity catalogs. You you can have 10, 000 tables in your Databricks or Snowflake.
You want to bring that into your catalog and, you can get a technical metadata, but what are you really interesting is the business metadata and how do you get the business terms and definitions and how do you get more context. So when a business user is like looking at that for self service BI, they understand what the data is, right?
And nobody I know I don't want to type in handwritten, like for 10, 000 tables, right? And all the problems, like what the actual metadata is. So how do you pass it like various documents, right? Like your design documents, your technical specs, your, whatever it is, right? Like various documents.
And then, you could have gen AI generate. Recommendations for metadata tags and, potentially [00:21:00] over time as you build new enhancements, your data lakes, data breaks, et cetera, you can have agents say, Hey, any new data comes in, right? Feed it, like some documentation. I'll at least recommend you some metadata tags, but you can add to your unity catalog or Calibra, et cetera.
So various kind of very specific usage type agents will be there, but Ideally we have one holistic framework, which will, you know, guideline and version control and all of that versus, 20 different places where we have been managing is difficult. So it's really right now we're trying to, work towards right.
Like that, what that general framework is. And, I think there's some, it's a new space. So I think everybody's experimenting.
Mike: It's just another layer in the database model. I think that people think of it as something much more complex, but you think about it, like if Salesforce and ServiceNow and everybody else is running their own agent and they're not connected to the same source of truth, you're going to have vastly different answers and you've created agent silos, right?
Which is going to be just as big of a problem as [00:22:00] data silos. It is a data silo, right? And I think that's where. The platform and the orchestration is becoming so important is that we need to bring everything back to a centralized set of reference data and truth and ground truth. It's been validated.
And then at the end, we need to figure out. Okay, all the inputs and outputs going between these agents. We need to track that and measure the value and accuracy and reliability of that system.
Nishith Trivedi: Yeah. And we've been doing like things like robotic process automation, right? The last five, 10 years, right?
So it's not it's just basically the iteration of that, right? It's adding gen AI and other AI kind of elements to it. And, but it's really automating things which you could do, either through rules or, road automate, like automating, like manual steps, which are repeatable, essentially.
Chris Detzel: So my assumption is governance is pretty hardcore for, Pfizer. You have, you're in over 150 countries with different, requirements and all of those things with the AI stuff going on with a lot of other things going on. How do you [00:23:00] think about that? Managing and how to get people to adhere to these things.
Nishith Trivedi: So first of all I think from day one, I realized, like that it is an impossible task, right? Like Pfizer, we have in our, what we call CMDB, the Configurable Management Database. We have 3, 000 applications, right? Which are registered technology applications. Platform, CRM, databases, data lakes, reports.
3, 000. There's no way as an enterprise data governance I can govern 3, 000, right? It's we already saying, hey, we're not going to Do everything, right? So how to be strategic? Now our group as a idea to analytics, right? Like we obviously own all the data lakes, right? And the analytics tool.
So that obviously would be a key area for me. And then, as from a as a chief data officer hat, right? What do you focus on? You focus on where the business value is. So you basically focus on where's the biggest bang for the buck, right? What is business priority? So if the business right now is prioritizing on, let's say, Better supply chain, right?
We want to make our supply chain more efficient at the, right now, obviously we Pfizer purchase a C gen right for oncology drugs, right? So there's a lot of focus and a lot of, investment in [00:24:00] oncology. And, there's an initiative called digital first oncology.
So anything around oncology and better data management calls, that other, vaccines is also important, right? Of course, but sure, quality they're doubling down there. There's things like in the clinical trial space, right? They want to basically have better like submission, right?
Like how do we do document generation for better regulatory submissions and all that, right? So the document generation, and knowledge mining of, documents is very important. So focus on that unstructured stuff, which we're talking about, right? So we do have to pick our places, but we pick places where the business is betting on.
And we basically say our job as a data team is to enable the foundational data and, linking of that data together to make that data AI ready so they can Work together. Help their processes or help their requirements. Sorry go ahead. I was going to reply to the other part, which is 100.
Mike: Go ahead. No, go ahead. Yeah,
Nishith Trivedi: you met. You mentioned the 1 50.~ I'm sorry. There's like a mosquito. ~The 1 50 countries. So there's obviously as a life science kind of it's a regulated industry. There's things where [00:25:00] like Pfizer is really good at because we've just been doing it forever, right?
Things like GXP, good manufacturing practices, HIPAA for clinical trial data, like patient data, or, the clinical trial participants that data is like blinded, right? Like very few people right outside of the global clinical supply can see who it is, right? So HIPAA compliance for like patient services and making sure the firewall between the Patient services data can't go to patient marketing.
So that stuff is like bread and butter because we've been doing it forever. And then there's all this reaction to new stuff, right? China PIPL, GDPR, of course, not new, 10 years old. China PIPL, for example, we had to figure out how do we react to that. And, what happened was we said, hey, we're going to have a China team for China data.
And so I had a person in my team who was my China MDM lead. And he actually got transferred over in our China data lake team, or they got transferred over to the China data analytics team. So We are not only SME and we support them from here's the type of platforms, here's, data migration, etc, but we don't have access to China data anymore.
So China data, specifically HCP data, PI [00:26:00] data, is only in mainland China. We don't have access to it at Waldorf for PRP purposes. We don't even have cross border transfer ability to do that anymore. In that case, it's like a separate team. And, we're not even governing them. We'll support them from a platform perspective if they need a catalog or if they need, tools and technologies, right? But they're managing governing their data locally. In other cases like GDPR and then there's, obviously government stuff like GDPR or the California Privacy Act, or there's things which are contractual, right? We buy especially pharma data, especially distributed specific contracts.
It's a Hey, here's how you can use it. Here's you can. And then obviously, as Michael you did earlier, right? Like now we're more patient centric. Like previously for pharma customers is really doctors and hospitals. And now for pharma, we're also consumer centric, right? So patients, consumers, caretakers, right?
We have various digital labs. There's a, there's a Super Bowl ad for like the Pfizer for All is a website where you can go to and ask questions, almost like a WebMD where you can ask questions, it gives [00:27:00] answers oncology specific, like Living for Cancer is a digital app for cancer patients.
So there's obviously, because of consumer technology and even for doctors like there's a lot of consent management and, preferences, which they put in. And so being compliant to, their data privacy, their consent we will use. These emails only for these purposes, right?
If it's a doctor, like we will email them, like if it's oncologist, only email them about cancer drugs, or don't send them emails about Paxloid or, COVID vaccines, something like that. So it's just being, respectful, of the consumer experience, and, making sure that we're complying to contracts as well as laws, as well as, consent.
Chris Detzel: Michael.
Mike: Yeah. Yeah. I was just going to say it's an amazing space to be in. And I think that. From the outside, everyone kind of looks in and, even going into a lot of these new industries that I'm in, you're like, how hard can this really be? And you get under the hood and you're like, Oh, there's 10, 000 regulations that we have to follow for each data set.
And no wonder the lift and shifts and migrations and transformations to some of these [00:28:00] AI tools is so challenging because your contracts might all be different on which ones can actually leverage an LLM or which ones can interact with a rag ecosystem. So it is an exciting space to be. How do you upskill your team and keep everybody learning and continuously accelerating with all of the changes, especially with LLM, where I feel like the entire architecture, at least even from our perspective, is Databricks has completely changed year over year.
Like it's evolving that quickly.
Nishith Trivedi: Yeah, I want to just quickly react to the pharma industry and how unique it is really. I think like when I first came into the pharma life science consulting, I Very early on, I realized that, hey, like the uniqueness is that, compared to e commerce where like the person who, makes the purchasing decision is a person who pays for it, is a person who gets the thing.
Over here, like the patient is getting the drug. It's really the doctor who makes a decision on what to prescribe. In some cases, the doctor can't make the decision is a provider who will say, Hey, like for this type of drug, this is the standard of care. Give [00:29:00] this the provider will make the decision based on sometimes the tiers of the, or the PBMs or the pharmacy benefit measures.
Say, Hey, this drug is in this tier, so will pay for it. And then the, obviously the distributors are the ones who take the data from take the actual physical pills. From Pharma, McKesson, ABC Cardinal will take the data, they will send it to a CVS, and then the patient will go to CVS or Walgreens and pick it up, right?
So it's the distribution is different, the payment is different, the decision of who buys it, who's going to pay for it, how much is going to be paid by insurance versus out of pocket, like it's all different, right? So compared to e commerce where you can go to Amazon and you say, I want this and you buy it and it'll ship it to you and it'll come to your house, right?
It's totally different. Very data rich, but very complex. A lot of middlemen and a lot of, the gross to net kind of you can see in the politics of the day, right? Like the gross to net how much, is pharma gouging people as a, it's really middlemen, right? There's all sorts of untranscripted, they're the, margin.
Mike: Even I didn't know this until recently, and I was talking to a consultant who specializes in [00:30:00] just this is medical coding, like how complex, even from the doctor's perspective, there could be 100 different ways to code it one procedure, and depending on which codes you select is how you're built.
And if your insurance covers it and what kind of medications are allowed for you. And that in itself is a whole ecosystem right in the variability of how you're going to get charged and what you're going to get provided.
Nishith Trivedi: Going back to your original question of how do we upscale the team and, myself, I think, definitely I think, when I started out, as I mentioned we had, Two teams and technically the one team was also siloed.
So we had just the catalog people and MDM people and then, one ontologist and, knowledge graph, et cetera. And, they were this is my capability here. I'm the expert in that. And now I've tried to say, Hey guys, for your own career growth, and also for us to be able to more cohesive, let's figure out how the platforms work together. And then also let's try to get some cross training. Yeah. In terms of, we have people from my MDM team right now help on reference data management. I have my MDM team members helping on the Colibra catalog.
We're, we're doing some migrations of, [00:31:00] various, teams from one catalog to another, things of that. I have people from the MDM team helping on Excel data and data quality, data observability. So there's, specific things where, you know, I'm actually helping as part of their goals I'm helping them Get exposure to new technologies, new platforms, even new, in some cases like people who work in commercial networking, R& D, etc.
So new domains. So that's one thing. Obviously with the new AI technology like we're still a foundational data team. So my goal is really getting data AI ready as opposed to doing the AI COE and, we have partners that we work with very closely who are experts in that. But I think everybody like I think at the Pfizer AI, like we're part of AI analysts and data practice, right?
Like from the, leadership down they're basically pushing for, various education. So there's, obviously all sorts of online classes or, online, opportunities. But really on the job training to me is the best one, right? Like I can, I'll get bored, like looking at, Coursera after a while, but.
It's Hey, let's try to find these AI projects and let's try to like make a [00:32:00] dent in it, right? Let's try to help them be a little bit better, right? Like their contact, their recommendations have a little bit more context or let their data be a little bit richer. I think that's where I enjoyed it.
The practical stuff we're doing a lot of POCs and I think in the AI world, that's how we learn, right? Is by doing POCs, you can't go straight to production. You have to iterate them. So just on the job learning, I would say more and then cross, at least for the team, more cross training ability to just see new areas, and I think most people like for their own career they are excited to try new things.
Chris Detzel: Especially the AI stuff, they have to, and so it's really good that you,
Nishith Trivedi: yeah. And I think everybody's worried, right? That Hey, if you're going to get left behind, if you don't know it. So I think, yeah, everybody has motivation, but I think it's also exciting. It's not just a negative part. It's also, Oh, for sure.
The cool, cool, shiny object. I didn't want to play with them. Go ahead, Mike.
Mike: I was just going to say the other thing is you have these groups, like the data scientists who were [00:33:00] doing traditional ML. Now you have these ML architects that have moved into the space specifically for large language models, and there's this kind of merge over now where it's less about the models that you're creating, which was more of a classic ML approach, still very relevant and certainly a model of experts.
It's going to be a mixture of experts. It's going to become even more relevant, but The LLMs is really more of a data problem right now, right? Because you've got this product that you're just handed, and you need to extend it with all of these intricate graph databases and vector databases and, traditional data sets and unstructured data.
And really, it's interesting that the data stewards and the, heads of data and the MDM experts are now becoming the experts and helping accelerate the movement of these large language models. And I think it's primarily because it is a data structure problem more than it is a AI and ML problem for 95 percent of the people out there.
Right.
Nishith Trivedi: The accelerate is key, right? So I think Pfizer did a great job, obviously, during the pandemic to say, hey a vaccine, which typically it takes five to 10 years to come out, they were able to [00:34:00] launch it in 10 months, right? It was like ridiculous, right? And now they're like, hey, why can't we do it for every drug, right?
The next oncology cancer drug, why can't the next set of vaccines, why can't we speed up drug dev, right? So really now looking at every part of your value chain, whether it's clinical trial, whether it's, the early discovery, whether it's regulatory filing, whether it's supply chain manufacturing, whether it's, the commercial process where there's, our medical team giving information to people, right?
It's like they're looking at every part of the value chain and saying, hey, where can we speed this up? Where can AI come and help make it better, make the process better and make the process faster? So I think really that acceleration is where the, value is, right? It's, you know, I think I'll give another example from the marketing world, right?
We had Then globally, something like 75 marketing agencies, which took, the global marketing content and said, Hey, we're going to convert this into 100 different languages, right? The same marketing content, we can now localize it for China, Brazil, etc.
Brazilian Portuguese versus Mandarin versus, I know exactly
Chris Detzel: [00:35:00] where this is going, but keep going.
Nishith Trivedi: Now, as you can imagine, right? Own the product master, right? We have the globally local language, and this is the brand name. Now we can just point to LLN to that and say, Hey, let's, here's a template.
And then now LNL, they create like 75 versions of the same content and different languages, local, we can say, Hey, like The local person, right? You still need to look at it. We don't want to just send it out, right? But there's all this review, but like now, so much faster. And so that's a new case, right?
Of so
Chris Detzel: much faster that it takes five minutes rather than months.
Nishith Trivedi: And like cheaper. You're not paying like 75 local agencies to like localize conduct. You can basically say, hey, the local person, marketing person in the country, just review the document, right? And because LLM can convert it for you, right?
So I think, and. My part is not the LLM part. My part is the value add of, hey, saying, I know that in this country this drug is this local label, right? So my part is really just the foundational data saying, here's the metadata or the data which you can [00:36:00] use to enable that.
Mike: I've been using localized, specialized large language models to help me get better at my own job, and it has been incredible.
I think I want to write a blog post about it at some point, but you can load in all these research papers of things that would take you days or weeks to comprehend and understand, and you can give the context of who you are as an individual, what your goals are. And start to ask based on the research, which one of these are important and how can that technology accelerate your own objectives.
And it is like such a different way of looking at research and how you can accelerate moving through content that, a lot of these research papers are really dense. They take you a ton of time to fully comprehend. And, you don't necessarily need to do that to the same degree anymore. If you don't have the time, you can really isolate and.
Create more value out of that same time by telling an LLM to help you align which things are more important for you to investigate. I love the
Nishith Trivedi: way that you wrote both, so please do work on that.
Mike: Yeah, absolutely. There's just so many ways like that, but they're not [00:37:00] huge efficiency gains. It's not transforming the way I learned or anything like that, but it is helping really accelerate, like I had an expert working next to me saying, no, you don't want to look at that.
Look at this, right? Look at this form of research. It'll be better for you. Spend your time there. So it's just incredible. I think that we're gonna see so much more coming. Even at Databricks internally, we have LLMs that we use to help us learn about our products. There's just so many products and so much complexity to each product that you can use LLMs that have essentially scraped every bit of JIRA and GitHub and documentation to help synthesize when you're looking for an answer that a customer like Pfizer comes to you and says, Hey, I'm having trouble with this.
Instead of having to spend three days researching it, you can go back and maybe cut that down to half a day. To come back with a solution. It's pretty cool. It's amazing how this is going to change the world, especially in something as complex as drug discovery, where you've got such a, a complex ecosystem to get something from, an idea or proof point to a finalized [00:38:00] product.
Chris Detzel: Absolutely. So just to sum all this up, or at least, parting thoughts, anything. What are you looking at for the future and the ship, at Pfizer and what your team is doing? What are you thinking about?
Nishith Trivedi: So I would say at least, as a data horizontal, right?
And as a foundational kind of data element, a partner to business and partner to AI and analytics team, right? It's really all about our vision is, hey, how do we make data AI ready? How do we make data fair? I don't know if you've heard fair, but it's a pharma r and d concept of fair is findable, accessible, interoperable, and reusable.
And then we have fair plus, right? So there's trust and data quality and all the other part of it, right? Really findable, right? So it's really how do we make sure. Hey, all the data in the data breaks is in the unity catalog. And we linked that to Colibra and there's one UI where it's a one global marketplace and the snowflake data is there and the redshift data is there and the, all the other Oracle or whatever an unstructured data and all of that we can find in one place.
Accessible. Obviously, it's like now that once [00:39:00] you find it, how do you get access to it? How do you have the right, privacy policies and encryption and, the right people can get right access to the right data. So all the standards policies around that interoperable. You guys come from the MDM world, right?
That's super important, right? Probably the most important part of all of this is you can have all the data silos and if it doesn't talk to each other, it doesn't help. So structured data, MDM, RDM, yes, harmonizing and unstructured data. Tagging it, interlinking it, creating a graph which can be read, and then reusable, right?
Obviously it would be okay, like now we build a data product or analytics product. How can we basically make it available to everybody. They can use it with creating the data mesh or data fabric or whatever, whatever term of the day, but essentially like creating something which you have trusted data with, APIs or, specific, things which, you have there.
It's governed. It's Essentially managed by somebody and there's owner and the ownership is important is documented and then people can now use it. And that's our goal is how do you make [00:40:00] it ready? How do you make it fair? How do you bring all these different groups together?
And I think previously at Pfizer, The clinical group worked with clinical data and the sales marketing group with the marketing data and the medical group with medical data. And now you can see there's many use cases coming together. Some of it over there, but now a lot more, right? And we have a lot of, hey, like the just now, right?
We're trying to get the clinical trial data in our data breaks to merge with the commercial data and snowflake. To help support our chief medical office, and chief medical affairs office and medical analytics team. And they're, they have their own use cases and we need all this data to come together.
Chris Detzel: Yeah,
Nishith Trivedi: It's lake to lake integration. It's you know, it's cataloged here. We have linears, right? Like now we can show that we can show the power of it. It's easy. It's trusted. So yeah. Speeding up kind of ability to do new analytics, which previously like the team and chief medical would have never ever spoken with the team and, clinical.
They don't even know what data is available now. So you can see what data is there immediately.
Mike: That [00:41:00] federation is key. And I think that's the that is the future of all large enterprises. The catalog has become much more than just a structured data set that a few backhouse people use. Everybody in the organization is using.
Everyone's talking about it.
Nishith Trivedi: And then the federated like just using the word, right? Like it's we can't even have one big data governance council. It's just too much data. We have federated governance. We're like, Hey, like there's a governance, the council, but there's multiple like siloed, right?
I don't want to say siloed, but multiple governance councils that maybe we have a federated way where Hey, if, let's say commercial medical need to talk together, right? Like we're going to have that small subcommittee or, clinical research and patient service need to talk, right?
For real world data. We can have a Spin up like a, working group, right? But the federated governance is important. Federated knowledge graphs, the other thing which we're trying to do right now is not only like we obviously have federate MDM, right? There's a clinical MDM, there's a contracting MDM, I have my enterprise MDM, and we have SAP, which is a supply chain manufacturing SAP MDM.
And those are like four big MDMs we have, [00:42:00] and they are connected together from a federated perspective. That's just MDM. But now we have A knowledge graph for ontology. I'm sorry, oncology and knowledge graph for clinical trial and knowledge graph for like internal medicine research and all that.
And we're like, Hey, all of these genes, they have studies, they have products, we have common data elements. We now creating a semantic layer on top of that and have a federated graph and, being able to ask questions to that federated graph. And, yeah. He'll create the Sparkle or GraphQL or whatever it is, Cypher for the different graphs and distribute that data and pull it back together.
But it's very difficult to get 10 different teams to work together. But if you have the semantic layer on top that's powerful.
Mike: And it's what's going to differentiate, it's what's going to differentiate companies like yours in the future, I think, is having that unified view. Everything from this point moving forward, you're going to build off of it, right?
And thank you so much for sharing your insights today. Really appreciate your time. This was [00:43:00] a great talk. I think we'll definitely ask you back at some point if you're interested. Of course. It's been a pleasure. Yeah, it's been a pleasure.
Nishith Trivedi: We're going to see you guys. We can have some beers sometimes. For sure.
We love beers. Let me know if there's a Databricks conference coming and if you're going to be there.
Mike: Oh, yeah, there is a data and AI summit. Would love to have you and if you send me your rep We'll see what we can do. All
Chris Detzel: right All right. Thank you everyone for tuning in to another data hurdles I'm, chris stutzel and don't forget to write and review us and
Mike: I'm, michael burke.
Thanks for tuning in.
Chris Detzel: Thanks everyone. Take care. Thank you
Creators and Guests
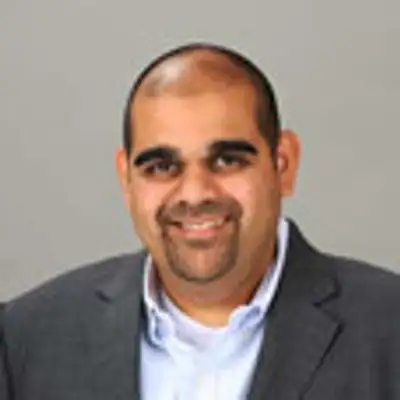
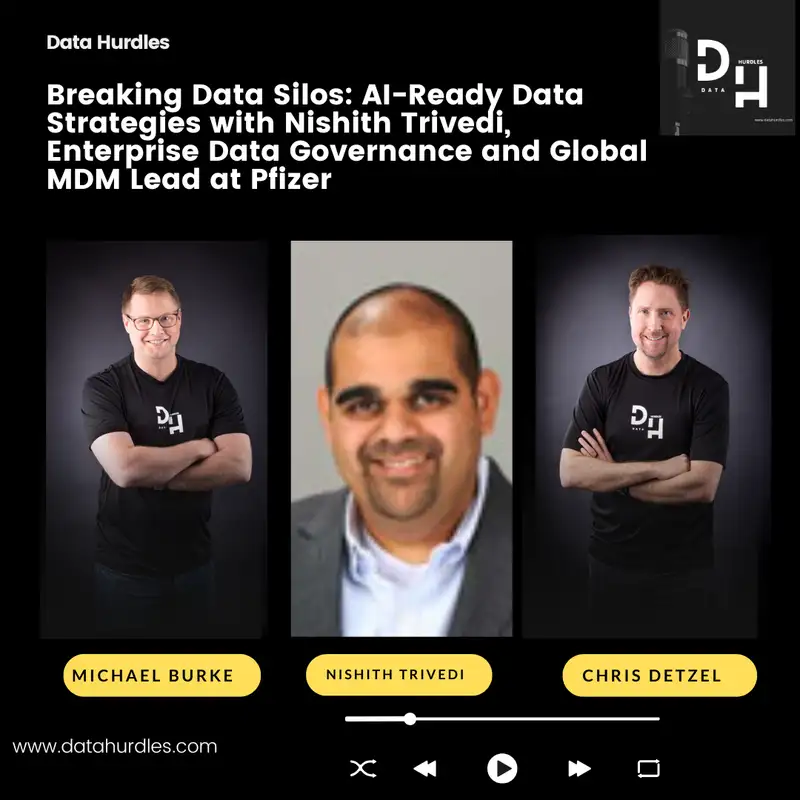