Challenging Data Management Norms: A Conversation with Malcolm Hawker, Chief Data Officer at Profisee
Download MP3Challenging Data Management Norms: A Conversation with Malcolm Hawker, Chief Data Officer at Profisee
===
[00:00:00]
Chris Detzel: I'm Chris Detzel and.
Michael Burke: I'm Michael Burke. How are you doing, Chris?
Chris Detzel: Pretty good. How about you?
Michael Burke: Good. Good. I had an amazing little mini vacation the other week, so I feel totally recharged, focused, and excited to be on the podcast today. We have a special guest. Malcolm hawker the cdo of prophecy welcome malcolm.
Malcolm Hawker: Thank you for having me glad to be here
Chris Detzel: I think everybody knows who you are malcolm. So that's it's really exciting that you came on. So we're excited
Is that all the data people do? I
Michael Burke: had to double check and I was like i'm pretty sure we've met in person and then I realized we hadn't it's just been you're so active on linkedin.
I see your clips. I see your voice It's funny, you and Scott, I think are the two guys. You're always on my feed back to back. It's amazing, right?
Chris Detzel: He's a tough
Malcolm Hawker: act. He's a tough act. He is a tough act to follow, but I'm honored to do it. I learned everything that I know [00:01:00] about. LinkedIn about public speaking.
More importantly, I actually learned from him. Scott and I worked together for years at Donna Bradstreet. We used to do these road tours where we would go on the road, extolling the virtue of third party data, extolling the virtue of Donna Bradstreet and why I should be doing business with Donna Bradstreet.
And he was like the headliner. He was the opening act. And I was the guy that had to go on stage after the opening act, after he softened up the crowd and everybody's excited about, about, in this case data. And he was the why and I was the how. But it was an awesome experience.
And I love Scott to death. He's a wonderful human being there. There are other Malcolms in the space though. I just got off a conference call with Malcolm Chisholm. Who's another Malcolm. So there's oddly a couple of us. And sometimes we get confused. Malcolm's on
Michael Burke: my feed, but you really,
Chris Detzel: I got every day.
I, I awesome. Cool. I love the loyalty. No, I was looking at your LinkedIn the other day. I think it was yesterday or day before and a chief data officer promotion, [00:02:00] congratulations.
Malcolm Hawker: Thank you very much. Thank you very much. Yeah. We're excited about being in the MDM space.
I'm excited about doing what I do. I have great conversations with C level executives all the time. I had them every day for three years. State was three years straight while I was a Gartner analyst and I continue to do it with Prophecy. And my role is an externally facing CDO. It's all about sharing what I know with the market.
It's all about having one to one interactions with prospects, with clients, with anybody, frankly, who has questions about data management, data governance, data strategy. I'm here to share what I know. So that's really my role. It's a bit of a unique twist on CDO, but I do appreciate I do appreciate you saying that.
Chris Detzel: Yeah, of course. Go ahead, Michael.
Michael Burke: I was just going to say, It's been such an interesting period of my career engaging with you and some of these other thought leaders in the space Could you walk us through how you got to where you are today as a chief data officer?
Malcolm Hawker: Oh boy [00:03:00] Interesting.
So the first 15 years of my career I was on a product management track. I was Trying and ultimately became a chief product officer. And that, that taught me just so much about the world of software, about the world of software engineering, building software. Cause I was a CPO for a software based company.
And I loved that track. I loved managing a product team. I love the problem solving inherent to product management. I love the interaction with customers inherent to that. And I was given an opportunity. I stumbled into it and I stumbled into. More data centric goals. Although I would argue when I was leading a product team, data was critical, right?
We, we were we needed data to run our business. Who's buying things. Why are they buying things under what situations? What are they clicking on? Not clicking on. I could continue, but I worked my way into an it role and worked my way again, up the ladder where I got into a senior leadership role, running an it function, implementing data governance, implementing MDM.
And from [00:04:00] there, I just fell down this data rabbit hole. I really had a bit of a formulative moment when I got hired as a consultant to answer the question at the time that I thought was just the most simple question ever asked, which was, Hey, Malcolm, I need to hire you and I'm going to pay your consultant wage and I need to hire you to answer this question of how many customers do we have?
And I'm like, I know
Chris Detzel: where you're going.
Malcolm Hawker: I got the, I got this. We'll run, we'll set up some dashboards, right? We'll we'll run some reports. We'll maybe even set up some data marts or this is back in the day of data marts or, we'll do this. And this is a slam dunk, right? This is the easiest SOW I will ever sign.
No problem. And it turns out that simple question was like my gateway drug. To data and analytics and into MDM into data governance into some of the more complicated things that we live in and breathe with every day as data professionals. And from there, I just fell down this data rabbit [00:05:00] hole, and I really haven't come out, nor do I want to come out.
I think. Found my way from a consulting engagement to to, to leading an IT function, to, to becoming what Donna Bradstreet called the distinguished architect, to becoming a Gartner analyst, to now being CDO at Prophecy. It's all been a bit of a natural progression, but at every step in the way I've been passionate about problem solving.
I think that's the through line of everything that I've done in my career. I've been truly and honestly passionate about problem solving, and I've been truly and honestly passionate about making things easy for my customers. And when you take that mindset, you can apply that into product management.
You could apply that into the sanitation arts, apply that into anything. And it's just that perspective and that mindset and attacking things with 110 percent of everything I've got, it's just really served me well. And I, looking back, I can't imagine how else or what else I would have done.[00:06:00]
Cause it's all been fun and it's all been a great ride. Oh, that's excellent. Go ahead, Chris.
Chris Detzel: No, and problem. The problem solving opportunity here is the when you start looking at all this stuff around A. I. Right. And so when you look at and more specifically, when you look at like A.
I. Governance as it's taking center stage right as a C. D. O. How do you look at when you look at the rapid proliferation of A. I. Especially like generative A. I. Impacted the approach to data. How do you look at How does that impact, the approach to data governance and organizations?
How do you, is it different than what you would think of normal data governance? Or is it just more intense now? It is.
Malcolm Hawker: Yeah, it is and it isn't. Bit of a consulting answer. It is and it isn't. And I think we need to be, and I think CDOs need to be extremely careful at separating Reality from platitudes.
There's a lot of platitudes out there, and that's mostly what I'm hearing. I'm hearing these useless [00:07:00] soundbites like, we need to focus on foundations, and we need to get our hands around AI ethics, and we need responsible AI, and we need AI governance. What does any of that actually even really mean? And I'm not trying to be rhetorical. I honestly mean that because I don't see very many people answering that question. I hear people talking about governance frameworks for AI, and that's fine. I hear people talking about regulatory impacts that are soon to be coming, if not already here. I hear People talking about ethics.
And these are important things. And I want our systems to be ethical. But what does that actually mean to a CDO? What do I need to do in order to enable more ethical AI? What's the difference between ethical management of data versus ethical behaviors of models, right? We need to go. We really desperately need to go from this world of platitudes and 80, 000 pithy soundbites into, okay, how do I put a shovel in the ground?
How do I alter my operating my data and [00:08:00] analytics operating model to integrate AI into the governance that I'm doing all day every day? What does it actually mean to be ethical? How do I quantify that? How do I measure that? These are all very difficult questions. I don't think we even have any solid grasp at answering that said, it.
There's a few things that I think that CDOs must be focusing on if they are not already focused on them. Generative AI based solutions are both built by And optimized by unstructured data text. These things are, they consume the Internet. Okay. Which is not necessarily known as a bastion of data quality, but it's text.
It's text based. So if you've got a significant chunk of your data estate that is in unstructured data, chances are pretty good. It's entirely completely unstructured. You need to get your hands around that quickly. Quickly, right? You need to start to understand things like rag patterns, vector databases, other things that you can use right now to try to [00:09:00] influence the behavior of these models that you're probably buying off the shelf and they're not building from the ground up.
Most people aren't building models because who has 12, 000 GPUs just picking around that they can use for the next 3 to 4 months, running 70 billion parameter models. Very few. And I would argue you don't even need to do that because they've become commoditized at this point. So separate the soundbites, separate the platitudes, start figuring out, like, how do I get my hands around unstructured data?
How do I even understand what a rag pattern is or vector databases? How do I start? What do I need to do in order to start using my data, my internal data that I have right now that is sitting in structured databases? How do I use that to start influencing? Generative AI models. What can I do to start deploying small scale POCs, internally focused POCs, so that I can check a box to my board that says I'm showing progress here, because if you're talking about platitudes, you're not making progress.
Michael Burke: I completely agree. And I think that, even as somebody who's worked in the AI space for a long [00:10:00] time, it is. Mind boggling how many platforms are that people are talking about and it's what are you using them for right? What is your real problem that you're solving? Yeah even if you have unstructured data and you need to make sense of it why these challenges and walking through the sequence of what needs to be done today Versus what do you need to be worrying about five to ten years from now?
And although ai ethics is incredibly important and you should discuss it of course the foundation of it early on You know Where we're getting in this space is becoming more and more complex. We're building off of more and more layers of other people's work. And until we get to that point where we say, okay, but why are we doing that?
What is the problem we're trying to solve and how is unstructured data or rag or fine tuning going to play into helping solve that problem? We're just, shouting from a soapbox and
Malcolm Hawker: you raise a really good point. And another advice I would give to CDOs out there is that there's really 2 swim lanes here.
There's companies with the [00:11:00] data science function. And there's companies without, if you have a data science function, if you're paying the expensive wages to have data scientists who are actually building and managing these models, some of those other conversations are going to be nearer and closer around ethics, responsible use of data and those things.
So I'm not trying to say those things aren't important, but I will say that the majority of companies out there will be operationally operationalizing AI through commercially available models that are mostly. Not exclusively pre trained stop talking about training models. If you're in that swim lane, if you don't have a data science team, chances are you're not training.
You may be fine tuning. There's a difference between those two things. Again, fine tuning. You're using text to do it. You're not using rows and columns to do it. So these are two very distinct worlds. Now, if you do have a data science function, one of the things you need to be focused on is finding a way to bring them together.
With what I would call traditional data management. Cause one of the elephants in the room when it comes to AI is that data scientists are [00:12:00] not using the output of traditional data management approaches. They're not using the output of MDM systems, data quality systems. They're not using data integration software from Boomi or from TIBCO or from whoever they're writing custom scripts to do everything.
They're going straight to the source. They're getting data straight from the source, right? And they're using custom Python, custom scripts, custom, everything to do what they need to do. Which means if you are doubling down on foundations, if you're, if you were investing in your legacy approach to data governance, if you're investing in your legacy approach to data management, data quality, data integration, it's not even moving the needle.
With your data science function, because they're doing what they've always done. So how do you bring these worlds together? I would argue the way that you build them to bring them together. As you start focusing on unstructured data, you start talking about the use of graph as in some sort of rag pattern to start providing context on structured data that can be fed into some of these models.
But you need to find a way to bring these worlds together because Yeah. Honestly, I think that there's [00:13:00] going to be economies of scale there that companies that do have data science functions that are paying for a custom development need to start to find ways to leverage.
Michael Burke: Absolutely. And I also wonder, I'd love your perspective on this data science when it started off was.
A couple of guys in the company in a room together building out Python docs and many companies that's still the same way that people operate, right? They have scripts. They've moved to AWS. This idea of a curated data set for AI and for machine learning. Yeah. Who owns that? Who should own that within the organization?
Malcolm Hawker: That's a good question. There's a lot of people out there talking about data products and that you expose these curated managed certified master data actually happens to align nicely to that, right? So if you have a gold master customer record, you can say it's a data product and you say, Hey, data science, data scientists go use this.
Like you could expose it in a catalog. Okay, great. But if I'm a data scientist and I'm looking at this and I was like, and you don't [00:14:00] know the business rules that were used to create it. You don't know its source. You don't know its lineage. You don't know any of the rules that were used to create it.
Are you going to use it confidently? No. Because you need to be, you need to be able to explain how your model is behaving, why it's behaving. And if you've got data that is basically come out of a black box that you can't explain, you're not going to use it in your model. So there are things I think we need to do to provide confidence to say, to, to data scientists to say, Hey, you know what?
Yes. You are inherently a different discipline because you are experimental, you are iterative. We don't need you to be 100 percent all the time, right? We honor the fact that you know what? The fact that 80 percent of models don't go to production is a feature, not a bug, right? Data scientists here.
That stuff is of course, only 20 percent because I'm an experimenter. I have to keep throwing pieces of spaghetti at the fridge to see which one sticks [00:15:00] yet. On the other side of our house, I'm hearing that's a bad thing, right? Because we have these different standards. The standards we have for data management are very different than the standards we have for data science.
One of the things that is that I think we need to start to understand here is we're going to bring these 2 worlds together. We have to honor the artistic endeavor. In many ways, it's a scientific endeavor, but in many ways, it's an artistic endeavor, but find ways to bring these things together.
And if we're talking about data products that data scientists can use make them your customer start having requirements, gathering sessions with them, understand what they need in order to do their jobs, because trust me they're doing the best that they can do. It's not oh you need to improve your hit rate with those models that are going to production.
I'm not entirely sure we do, but there's a lot of finger waving around that right now. I just don't understand it.
Michael Burke: That's really interesting. Completely agree. I think that the, depending on where you are in your A. I. N. M. L. Journey, you have a lot of people that are used to doing experimentation at the tip of the [00:16:00] spear.
And then you've got everybody else underneath it who's production izing. And molding and conforming to standards, right? But those guys at the front, by the time they finish that experiment, they're not the ones that are going to necessarily run and own that throughout the entire ecosystem. And they probably shouldn't be, just based off of the value that they provide by creating the next thing.
But I think it's a really interesting shift and definitely something that I've seen from an opposite angle in the data science space of, needing to take on more responsibility and saying, Hey, you can't just jump to the next. Thing you have to build out a data structure and productionize your models and you have to manage them.
And that's where this MLOps and these other kind of subsets of large organizations are really starting to emerge.
Malcolm Hawker: Yeah, don't get me wrong. Everything you just said is absolutely true. And just because I can assert confidently that data science is more than iterative experimental. Endeavor than traditional data management.
That doesn't mean we need to be chasing unicorns, right? It doesn't mean we need to be trying to build models to [00:17:00] explain things that the business doesn't care about. We learned that the hard way in the big data days when people were deploying Hadoop clusters and coming up with answers to questions that nobody was asking.
So can we do a better job of pulling data scientists closer to the business? Can we do a better job of making sure that they move faster so that by the time something does get to production, it's still relevant? Yes. Yes. Yes. This is the reason why I'm really optimistic about what I would not data products, but data product.
Management product management integrated into data and analytics, applying more product management disciplines and product management rigor to everything we do in the data and analytics world, whether that's building data science models or whether that is building traditional data products, like a gold master record or a pipeline.
So more product management, get us closer to the business. Those things are all very good because doing just useless science experiments, isn't helping anybody.
Michael Burke: Totally agree. I agree a hundred percent. So [00:18:00] let's another question. I think that Chris and I have both been pondering a little bit offline.
We talked about this a bit is where does the data mesh architecture Fit into all of this, right? And how are chief data officers? Before you get
Chris Detzel: into that, I do know Malcolm is a big data fabric guy. And knew this would have some, so let's get into that.
Malcolm Hawker: Oh, you oh, okay. So I'm a data mesh contrarian.
And just saying that will probably cause some of the people listening to this to, to clutch virtual pearls. And let me tell you why, let me tell you why I'm a data mesh contrarian, the underlying assumption, the underlying driving assumption sitting underneath the data mesh. And what, and everything I'm about to say is through the lens of what I believe the holy book of the data mesh movement to be, which is data mesh by Zamarkand.
If you have other perspectives, and there are many other perspectives out there because they've adapted as [00:19:00] meshes have continued to fail and not get deployed and not get deployed. People have changed the definition and move the goal posts of the data mesh. We have separate discussions about that, but I look at the data mesh through.
Data mesh buys a mock that I've read three times because I had, cause I had to read it three times. It's that dense. I am not a believer in the mesh because the underlying business assumption and the underlying assumption that is basically fuels it is that centralized data management architectures are inherently bad, do not meet customer needs, are too slow to adapt and too slow to move and are ultimately unproductive for the business.
That's the underlying premise. Like the centralized data management architectures are no bueno. So what the data mesh does is it swings everything to the other extreme without fully abandoning centralization of some things. And this is where things get a little bit murky in the book is the acknowledges that there's a place for shared data management and for the management of, I think she calls them [00:20:00] poly seams, the containers of data that would be moving between business functions and between domains.
What I would call master data. So there seems to be a recognition that there is some role for centralized data management to play, but we've gone all in on decentralized data management. And I have yet to see a compelling business case. Like a legit business case that says going to a complete spiderweb architecture is more cost efficient for the business.
It may be better for individual autonomy within domains. Don't argue that, can't argue that, it's impossible to argue that. But overall, from the business perspective as a whole, is there a net positive ROI of implementing a data mesh insofar as ZAMAC would define a data mesh? I have yet to see it. What I what I see is data product owners, domain authority, domain centrist centricity without data sharing without any sort of centralized data management patterns without any sort of approach to data governance [00:21:00] where that's problematic because I
Michael Burke: was just gonna say the funny thing is we centralized data before.
As things grew within our ecosystems because we couldn't maintain governance right and definitions fell apart So so your pendulum, right? That's what it is
Malcolm Hawker: Yes, and it doesn't recognize the reality that everybody has always known but seems unable to fundamentally acknowledge, which is that requirements Governance.
I could use a lot of different words here, but let's, for now let's use Governance in essence existed three layers within organizations. A enterprise wide level, a cross functional level and a functional level, and you can give autonomy over the control of data. The governance data at the functional level.
But that how does that support a cross functional enterprise wide use case? If your CEO asks you, how [00:22:00] many customers do we have? There is only 1 answer. Hard stop. And you don't want to be the guy or gal that saying, Oh, depends through what lens who's asking. I've tried that. It doesn't work. It doesn't work.
So you need to be able to support all three. You absolutely positively need to be able to support all three. And there is a role for cross functional data management and data governance. There is absolutely a role for that because you need to find a way to be able to support all three. Another way to look at this is the idea or the concept of truth, right?
Truth is contextually bound and there's three contexts in any business. So the idea of there being a single version of truth to rule them all is simply untrue. There are multiple versions of the truth. Now, one may matter more than another. The CEO's view may matter more than the director of marketing, but there are multiple versions of the truth that we need to be able to support.
And that's something that the data mesh just seems to have a hard time [00:23:00] With and really, nobody has much of an answer when I start asking questions around. Okay that's fine. Give domains complete and total control over their analytics. But what does that mean from a cross functional perspective?
And how do we and how do we support those cross functional use cases? There's not a lot of guidance there with within the data mesh. And I think that is highly problematic.
Michael Burke: Yeah, and I think the other thing that we always consolidate when we talk about this at conferences and even in reality is You know, this completely varies depending on what industry you're in.
What are your priorities? Are you prioritizing speed or are you prioritizing structure? Yeah. Are you prioritizing governance? I'm in a startup now, right? We have one database and half the people have root access, and I'm like, Hey, let's do a little bit better of a job with governance here.
People are like, what is governance? And so just changes depending on where you are. And, at a 5, 000 person company, things would move so slow with getting access to data sets. We couldn't innovate. So I think that the same thing with a data mesh or data fabric, or even in my case at a [00:24:00] startup, one database or a table the operation needs to be defined based off the outcome.
And in a complicated ecosystem, it becomes harder and harder to understand what is your top priority. How are you going to mold a strategy around that?
Malcolm Hawker: Yeah. The complexities. This is one area where I love talking about AI for governance because I honestly think that AI will save us because we have a hard enough time coming up with business rules, like one set of business rules to rule them all right.
The top level of that pyramid that I was talking about, we have a hard enough time like coming up with one set of business rules that can be deployed. Now the right model, the model that I'm talking about is N Sets and the three is a conceptual model. There's probably more layers than that, but you get my point.
There's, and you could even have business rules at a domain level. I don't argue that point of the mesh, by the way, I that, but it's not an either or it's an end. So you're [00:25:00] talking about N sets of business rules, right? N sets of quality standards, N sets of definitions, N numbers of models, N numbers of hierarchies.
And for the average governance maturity of most organizations out there that's forget about it, right? It's a non starter. This is where the fabric comes in, which is, I believe is, A great conceptual model to help us start talking about how to automate the management and governance of data because we desperately need it.
And I will argue until I'm out of breath that AI is not a viable that AI somebody who says that AI isn't a viable option here because I think it absolutely positively is AI seems to be really good at languages. And that's exactly what data is. It's an abstraction of a language.
It is an abstraction of a language, the language of business, quote to cash, procure to pay. We stick things into rows and columns because [00:26:00] that's how we've always done it. But the irony to me is that what we're struggling with now from a Gen AI perspective to take things out of rows and columns where there's no context at all.
And find ways to overlay context which is narrative, which is a story that the AI can actually consume and make meaningful. So I asked the question to a friend of mine last week, Juan Cicada. I said, Hey, we seem to be moving heaven and earth here to try to bring these 2 worlds together.
Unstructured data, structured data, adding context to rows and columns and wouldn't it be, wouldn't it be cool if our business applications just tracked data in this like ongoing narrative, right? Instead of dropping things in as rows and columns and joins and maybe we just start storing things like an ongoing narrative, like Malcolm click button, right?
Michael bought product x at date at y time, right? And just start storing things in stories instead of rows and columns. I think it's a bit of a mind [00:27:00] blower, but I don't know. I'm optimistic that, AI enabled data fabrics will get us to the point in the future of automating the classification management of data because we desperately need it.
Michael Burke: I totally agree. I think that, there are so many use cases where that holds true. I still get hesitant about some areas like health data and places where, you're going to have the cost outweighs the reward quite a bit. And that manual painstaking review has merit. But in 90 percent of these cases.
What are you really doing with the data? You're putting into some kind of report or spreadsheet, and you're making a business decision based off of it, and you don't need it to be 100 percent accurate. You don't even need it to be 99. 9 percent accurate. You need it to be above 80 percent accuracy or 90 percent accuracy.
And that's where I think Classifications with AI can get us there, I'm already seeing that it's doing better. Then some data stores, and in a lot of organizations it's [00:28:00] doing better. It's amazing.
Malcolm Hawker: I don't, I, I hear still a lot of yelling at clouds up there around AI like it's never going to automate dah and.
Don't get me wrong, folks. When I'm talking about automation, I'm talking about a future state between now and the future state, of course there's going to be human oversight, right? And nobody's going to turn over, making signing their name to some sort of, regulatory sign off and turn it over to robots.
It's just not, it's just not going to happen in the short term, but I think we're going to ultimately we'll get there. So we've got a long way to go between where we are and where we're going to be. So don't get me wrong. I'm not talking about like this automation happening tomorrow, but. What I see in a future state data fabric is this world where we finally and once and forever bring together the world of analytics and operations.
And if we paid attention to metadata, if we paid attention to transactional data, and we did it in a way that understands. When transactions are successful [00:29:00] and understands when transactions fail and under what conditions are they successful? Under what conditions do they fail? What data led to successful transactions or fast transactions?
There's data everywhere that tells us. When data quality is sufficient and when data quality is insufficient based on the speed of transactions as they execute within our systems based on this, I could go on, but we have systems that can tell us when things are working or when they're not working. A little tiny microcosm of this today is something called data observability where we're.
Somehow we've been able during in one little use case in one little swim lane to say, okay, here are the conditions that mean things are going to work. And here are the conditions that suggest things are going to break. This is exactly what I'm talking about. But instead of looking at a pipeline, look at a business process.
Quote to cash, procure to pay. What are the conditions that lead [00:30:00] to successful transactions or valuable transactions or repeat customers, right? How do we bring all these worlds together so that we get to a point where, Oh, okay. I don't have to have squabbles around. How do I define data quality as a part of my data governance committee?
I can actually look at the data and it'll tell me when data is fit for purpose and when it's not fit for purpose. I love that. I know it sounds fairly conceptual, but that's what I view a future state. Okay. Data fabric to be future, future, future state. Today. It's just basically this hyper virtualization layer.
That's cool. That allows you to run, SQL queries against graph databases and allows you to virtualize and, basically remove the differences in essence between a lake and a warehouse. And that's cool. But I see things going far, farther future down the road in the future that becomes something completely different.
Chris Detzel: Yeah, totally in the future real quick, but before we do the future stuff, I knew that, once we started talking about data mesh, you'd probably [00:31:00] start talking about data fabric. So I knew your research,
I used to work at Forrester and for many years. And the one thing that they really started writing about was the data mesh piece years ago And you guys start or gardener started writing more about you know The data fabric piece and one went big in one area and the other went in the other and then Yeah, i've listened to some of your stuff.
So I knew
Malcolm Hawker: I'm not opposed to domain autonomy, right? I just I just know that Incentive structures are such in the corporation that sharing across domains is just not going to organically spring from the earth It's just not Anyway,
Chris Detzel: completely agree. So I had a bunch of other questions, but we're going to skip a lot of those.
And and get to future state as we think about cdos and you know What are some of the trends over the next few years that cdo should be aware of in your mind in your thinking? Yeah
Malcolm Hawker: Boy we've been talking a [00:32:00] lot about one of them and it's not going anywhere. So check on the AI box.
That's certainly one. I'm optimistic that the seed of product management will slowly take root. Because I wanted to, and I think we could desperately benefit from having more product management integrated to the data analytics function. There is, I think there is more that we could be doing and more that we could be talking about from the perspective of Perspective of sustainability.
I was a guest on NPR in San Francisco Bay Area last week in a radio show talking about this very thing. I published an article in Forbes two years ago now about our problem with data hoarding. And it's a huge problem and the environmental impacts that has because this data needs to live somewhere.
That's another area where I think we could be certainly doing a better job. We absolutely positively need to figure out once and forever and for [00:33:00] all how to quantify the value that we are delivering to our business partners. We need to get over this hump that.
Quantifying the value of data and analytics is like some sort of combination of world peace and cold fusion and like this impossible Gordian knot that cannot be unraveled because it's simply untrue. The idea we can't quantify the value we deliver to organizations is a pure myth.
And I would argue if we're out there saying that it can't be done we are the data and analytics people, right? We're the people, the pithy joke I say is that we're in the modeling business. I'm not talking about blue steel. I'm talking about building models that actually help you to understand, correlations between things and potentially even causal relationships between things that are not directly related.
And so my message here is that data leaders absolutely need to be focused on. Quantifying the value that they deliver, right? We need to be closer to our business partners. We need to put [00:34:00] the customer. And I use that word in purposefully. We need to put the customer at the center of everything we do.
We need to stop moving away from the use excessive use of platitudes. We need to stop talking about the thing that gives us jobs. The thing that gives us jobs as garbage. It's not. It's unrefined gold. Can you imagine if the COO of Frito Lay kept referring to their corn as garbage, or if the COO of Anheuser Busch kept referring to their water as garbage, but we do it every day.
We need to, we need to stop with that stuff. We need to recognize data quality as an opportunity. And not a burden we need to reckon data quality gives us jobs. And by the way, we need to start seeing the positive intention of everybody in our organizations because data quality problems exist.
Not because people are trying to make our lives hard. But because our business functions operate differently, and that's by design. So there's a whole bunch of things we [00:35:00] need to do as data leaders to look at our data, our positions, our roles, our jobs, our value differently than what we do today, because the data suggests that we've got a little bit of an uphill battle.
The last CDO survey from Gardner said that only 44 percent of CDOs believe they're delivering tangible value to their organizations. And that's a self, and that's a self assessment, that's a self assessment, right? So how do we do better? I just gave some perspectives that I think are going to be important to help us improve our mindset, to help us improve our data culture.
Oh, by the way, data culture is not a dependency for CDO success. It's an outcome of CDO success. So if you're a CDO out there saying, I can't do anything because our data culture sucks that's that is you're disempowering yourself for one. And for two, you're putting things in the wrong order, find a way to deliver value, build a customer [00:36:00] 360 build, do some dashboard find a way to solve a customer problem, find a way to deploy some gen AI solution for an internal use case.
That's relatively low risk. That can still allow you to show progress when it comes to AI, deliver value to the organization and do that and do it again and do it again and do it again, and over time you will be seen as a trusted business partner that is delivering value. And when you do that, people will say, wow, how did we ever operate without that CDO?
Then all of a sudden, miraculously, you'll have a data culture, but data cultures don't happen overnight and they don't happen as a deliverable. They happen as a result of you providing value.
Michael Burke: It's so interesting you brought that up too, because I saw that time and time again, when I was at all of these different conferences with Reltio and others working in the data space, this self victimization of your inability to push forward.
And at the end of the day, when you look at the people [00:37:00] that you dive into what really are their problems? They're smart people, right? They're motivated people. It's, they can't communicate what's at stake effectively to their leadership and they have trouble sequencing their work. And I think that like to what you're saying.
What is the smallest increment of value that you can deliver in your organization to move you forward in a direction that's meaningful? And, I think that a lot of people who work in this space a long time, they see that, right? They see that but there's just especially people that kind of had this linear progression of being a follower, doing a task, cleaning and munging data.
And then they get to this position of leadership and all of a sudden they have to sell themselves. Like they have to sell their department to create value. And, I think that it's one of these things where. A lot of the customers I even see that are solving and investing in solutions to help with that, to help get more organized, some of them are really big and they need it, but others just need some handholding.
They need to say how do I do this? How do I sequence the work to get to a place where I can enable the rest of the business? [00:38:00]
Malcolm Hawker: Yeah. And when they get, and when they go to get that handholding. They approach analysts, they approach consultants, they approach some thought leaders, or they approach thought leaders in the space.
And what do they hear? They hear, hey, you're going to need to embark on a 6 to 9 month maturity assessment, followed by the current state assessment, followed by the gap analysis, followed by the implementation of our 12 step data governance framework. I used to hear this over and over and over again.
So first of all, forget the current state assessment. Forget the maturity assessment. It's not moving. They're not moving the needle. By the way, if you wanna know what your data and analyst governance maturity is, or any data management maturity, it's 2.2 to 2.7 on a five point scale. I can already tell you what it is because every time you do it, the answer is always the same , and it's not solving any problems for your customers.
In the short term, it's not driving any value. It's putting money in the consultant's pocket. Yep, [00:39:00] there's nothing to stop you from sitting down and having lunch with your chief revenue officer, your chief procurement officer, talking about the 3 things that could be different in order to make their lives easier.
And you don't need Deloitte to tell you how to do that and to have that and to have that conversation. Yeah, you're exactly right. Michael, people are looking for help around. How do I get started? I want to put a shovel in the ground. I want to deliver value. I want to get going. But when they go to the.
The thought leaders, the people that are supposed to advise the people that are supposed to guide, whether it's any of those groups that I mentioned, what they typically hear is the same old that will lead to a lot of money being spent, very little value being delivered and a lot of frustration along the way, which a big part of why CDO tenure is only 18 months by compared to double and triple that for CTOs.
That was great.
Chris Detzel: That's true. I remember when years ago, the CIO role was 18 to 24 months, right? And so that's getting better. So I think over time as the CDO kind of continues to [00:40:00] drive the value drives those outcomes that, the business needs just like CIOs do today. And as important as it becomes, people start seeing the more important the importance of, and people just get smarter as they go.
Some of these CDOs are just like, Okay. We've never done this before, so here's how we're going to go do it and they're making shit up, and Saying that
Malcolm Hawker: So well, yeah, but michael to your point we do suck at marketing. So so you know this is why another reason why i'm optimistic about the benefits of product management because all product managers Will tell you need marketing as a part of a go to market effort.
They'll tell you that they you need exceptional product design You and I could go on and on. But imagine a world where you were tasked to build a product, right? Like maybe it was a phone or maybe it was something. It doesn't matter. And you launched the product and nobody used it.
Or the people are using or complaining about it, or they even went so far as to go buy it [00:41:00] from somebody else. And not you, the internal provider of that product. If you were the product manager of that product, what would you think? What would you assume? You'd probably say, man, what did we miss? We didn't solve the customer's problem, right?
We didn't we didn't deliver value to the customer. What do we need to do better? Differently. Instead, a lot of it, a lot of what we do, frankly. Is blame the customer. This is this is data literacy, right? And don't get me wrong. Some training will probably always be required. And I prefer the word training over data literacy because it's an it speaks to enablement.
It speaks to education. But the idea that a lack of customer skill. Is the reason why you're failing to deliver a problem, deliver value again is disempowers you, right? Because it puts the hands in there. It puts the power in their hands. It says, okay my success is a function of their knowledge level, which probably isn't even true, by the way, [00:42:00] right?
Because if you build a very customer centric, design centric, easy to use, easy to understand product, I would argue people will use it. If it's solving a problem, they're going to use it. And if people are complaining about it, it's not because they lack literacy. Okay. It's because you probably built a crappy product.
Michael Burke: It's true. It's true. And we don't, we as data professionals do not dive into problem spaces the same way that product people do. And to your point earlier, that emergence of either data leaders going and getting that training or experience to understand how to operate and build a product or bringing in product people who can help with that, and saying that the CDO isn't just a technical leader, they are a product leader. And the same way that the chief product officer and even the chief technology officer sell their services, data has to be in the same sphere. Love it. Awesome.
Chris Detzel: Interesting. And last question I'll ask how do you see the role of the CDO evolving from these [00:43:00] trends?
It's always got to change, no?
Malcolm Hawker: Yeah I'm bullish because I'm an optimist by nature. And I tend to see things positively. I think we're going to continue to have a few bumps along the road. Particularly for the next few years, but I am CDO role in the long term because I cannot imagine a future where data isn't where data isn't the primary fuel of the business.
I just cannot see a future where that is not the case. So we need people who are experts. We need people who understand data. We need people who understand data governance and data management. We need to understand. We need people who understand how AI systems are built. And optimized and governed.
So I'm really optimistic for the role in the longer term, in the shorter term, I think we're going to continue to have some bumps, but I'll tell you, I spent all the last week in Boston and there, there are rays of hope, I think on the horizon, I think we have [00:44:00] some younger generations of people that look at problems slightly differently.
A newer generation of CDOs that see. The same old that we've been doing over and over again. There's probably a better way. I see a younger generation of CEOs who are willing to question the status quo, who are willing to take some risks, who are willing to try some new things and are willing to say that the DM Bach isn't the answer to everything.
And don't get me wrong. I own one. It's sitting over there and it's got some great answers in it, but it's not the answer to everything. So there's a new generation. I think of people that are asking all the right questions that are willing to and able to take the reins. I think we just need to equipment with equip them.
And that's a big part of what I see my role to be here is to be provocative and to get people thinking a different way, because I'm honestly, I'm truly dedicated to changing that 44 percent number to something closer to 70 to 80%. Because I know. I know [00:45:00] data can transform organizations. I know it can, cause I've seen it happen and I could list the number of companies here that are doing it right now.
We don't talk enough about successes, but I'm bullish about the future. I'm bullish about the role. I'm bullish about data. It's why I choose to do what I do.
Chris Detzel: So passionate, man. I love it. That's awesome.
Malcolm Hawker: We need more of that too.
Chris Detzel: Yeah, I mean if people could be as passionate as you are about data, man. Wow
Michael Burke: Love it no, malcolm. Thank you so much for your time. This was incredibly helpful and informative. I'm sure viewers are going to love it don't forget to rate and review us if you're listening and have a great day. Thank you. Thanks guys. Enjoy the chat
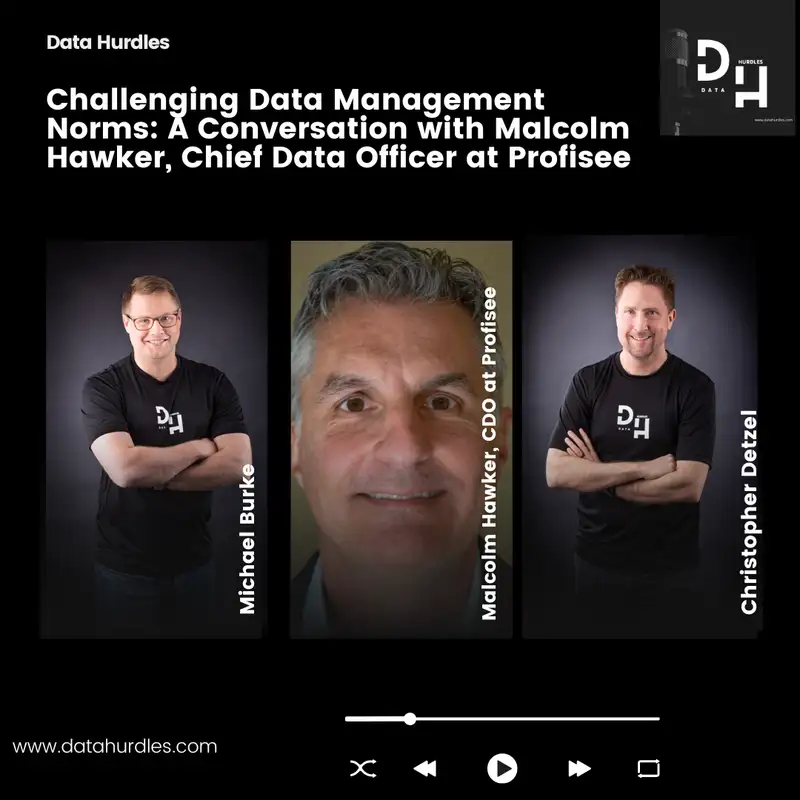