Clean Data, Business Context, and the Future of Analytics - Featuring Noy Twerski, Sherloq Co-founder & CEO
Download MP3Noy Twerski - sherloq - Data Hurdles
===
Chris Detzel: [00:00:00] All right. Welcome to another Data Hurdles. I'm Chris Detzel, and
Michael Burke: Mike, we have you on. Yes. Yeah. It's been a while. Holy cow. Yeah, it's nice to be back. I think we took a good two month hiatus here for a little bit. Nice to see you. How have you been, Chris?
Chris Detzel: I've been good, man. It's February and it's hot in Dallas.
It's 80 degrees here. But it's good. How about you?
Michael Burke: I'm doing really well for those that don't follow me online. I just shifted careers again. So I joined Databricks as a solutions architect and I'm loving it. This is an amazing company. I've worked with the product for years. Probably do a whole nother episode on Databricks later on and bring some folks on, but yeah, amazing product, amazing culture, happy to be at the company.
Chris Detzel: Yeah, today we have a special guest Noy Twerski. Is that right,
Noy Twerski: Noy? That's perfect. You said it the best I've ever heard, I think. Amazing. Tough one. I practiced
Chris Detzel: it, actually, before this episode, I, welcome to the podcast. We're excited to have you on and we know, of course, we want to know about a little bit [00:01:00] about what you do, what company you work for.
I know you're a CEO and founder of it, and I'm going to let you tell us from where and everything else.
Noy Twerski: Amazing. So first of all, thanks so much for having me and super happy to be here and share our thoughts and what we're doing and a little bit more about what we're building at Sherloq. So very briefly I'll give a quick intro about Sherloq and about myself.
Let's start like from Sherloq. So Sherloq, I'm the co founder and CEO. Sherloq is a collaborative SQL repository. So one place for all your queries on top of your existing SQL editor. That means that we're a plugin that sits on top of. Databricks editor, for instance, on top of Snowflake, and pretty much any SQL editor that's out there.
And we also have a plugin that sits on like the JetBrains editors. So DataGrid, PyCharm, stuff like that. We'll get into the product in a second, but I'll introduce myself also. So yeah, I'm Noy, a co founder and CEO. I founded the company together with Nadev around two, two and a half years ago, [00:02:00] something like that.
I have a quick background. I was a PM, I come from like product management, so very much involved in the product, as you can guess, which is very like relevant for this type of product. And so I have over 10 years of experience in analytics and in product management. I've worked in various startups and enterprises.
And actually as a PM, I felt the pain of scattered SQL. I didn't have in one of the startups, in my most recent startup, I actually didn't have a data analyst to do the analysis for me. So I would write the SQL and then when people ask me, how did you get this number? I would send it over on Slack.
Oh, so that's what, yeah, but you might be familiar with this. You gotta love those
Michael Burke: questions, right? Who really understands the code to get to that number, right? You're like, here you go, here's the sequel, take
Noy Twerski: a look. Exactly. But the funny part is that if you don't know where you put the sequel, then you don't know how to explain it because you don't even remember how you wrote it yourself, so that's where, that's where it hit me. And then [00:03:00] Nadav, so he's a very good friend of mine from our computer science undergrad, a few years back, he was a data analyst and then a team lead of data analysts. And when we decided to found the company together, it was very clear to both of us that we're going to be doing something in data analytics.
Cause we're both like from that domain. And we were talking, we had this assumption of sQL being everywhere and SQL being scattered and we talked to our data analysts friends, and we were asking them what's your number one challenge when you're actually doing your day to day work, right?
And 80 percent of them told us that they're like, they don't know. Where their tables are, where their fields are, where their SQL is. And that's like where, how we started the company. So yeah. So that's where we began. And since then we've, we've been working on this for, again, for two or two and a half years, something like that.
Yeah.
Michael Burke: It's amazing. And as somebody who is a practitioner of SQL, I love SQL, by the way.
Noy Twerski: Yeah.
Michael Burke: I've used tools like this before, like things that save snippets, or things that track kind of the observability to some degree, more from a government's [00:04:00] perspective.
Chris Detzel: Yeah.
Michael Burke: How does Sherloq really differentiate In this space, it sounds like it's much more of a tool made for the engineer, is that right?
Versus the governance layer.
Noy Twerski: Exactly. So it's made specifically for like data people, for data teams, specifically for data analysts, data engineers, data scientists, and for engineers for like software developers. Those would be like our main users. So definitely very straightforward.
And I agree that like governance tools have all of these types of stuff, but this is more like a productivity tool and that's how we like to frame it so yeah.
Michael Burke: Really interesting. And how did you identify this pain point? I know you were working in the field. What was the spark that lit within you to start a company to do this?
Noy Twerski: Yeah. So we get asked this a lot and I think that what's like important to understand is finding a really good problem is apparently harder than it looks, right? Because problem that's actually worth solving and actually like building a startup out of it needs to be a very [00:05:00] hard problem to solve. And when we started Sherloq, we were looking at all types of different like data problems.
And what was really interesting to see is that when it came to as I said, when we asked people like, what's your number one challenge, a lot of problems already had some sort of solution. So data catalogs are out there, right? Like data governance tools, metadata platforms are out there which you probably are familiar with a lot of them.
And we were like, okay, they're a really good problem. Like solutions out there. And when we were like drilling down, we understood that SQL and scattered SQL specifically still doesn't have a good enough solution. It has all kinds of alternatives, which we can talk about, but for specific, specifically for ad hoc queries and those day to day snippet queries that you write most of the time, like 90 percent of the time as a data person, those actually don't have a good enough solution out there when it comes to creating like a collaborative workspace for all those queries.
So that's. That's when we understood that this is something that's worth like validating [00:06:00] and worth investigating. We actually, with this idea, plus one design partner, which we can talk about also, got into, accepted into Y Combinator after we raised our seed round. And then as part of Y Combinator, what we did most of our time was spend a lot of time talking to potential users and customers and getting and validating and building the product together with them.
And then it became very clear that this is like something that's worth worthwhile and worth our time. Until today, actually yesterday, I can send this to you later. We see so many like people posting on Reddit, like where do you guys save your SQL and stuff? And. As time goes by, we get more and more of our users to post them write Sherloq data IO.
So yeah, so it's really cool to see that this is like still a problem, but we're getting there. We're trying to spread the word as much as we can. Yeah.
Chris Detzel: That's really cool. And out of ignorance, probably when you look at the scattered SQL, is it that these people are building? These engineers are building in [00:07:00] data catalogs or in different areas.
And then, Hey, look let's attach this tool to this thing and we can hold our SQL so we know exactly where it's at. Is that kind of what it does or is that.
Noy Twerski: Yeah. So definitely you can think about it, like something between a notepad that you have on your Mac book probably and GitHub it's really in the middle.
Yeah, and it's goal is to be like a data catalog for queries, right? So that's how we also can frame it. But the best way that our users get it is something between Notepad and GitHub or OnePlace for your queries. So yeah, definitely. The
Michael Burke: thing that I think, and that's all just to describe this in a little bit more detail is when you start Like one snippet isn't going to hurt anybody, right?
One, maybe even ten, if you've got ten different scripts you run regularly, but when you have snippets that were run on snippets, and layers of things that compound into some business report at the end of the day, all the domain knowledge gets lost on how we got there. I think that's the scary part is, and all of this could be done in a series [00:08:00] of one week, right?
And then you go on to the next project and you don't touch it for a year. And so I know for me, I've definitely felt this pain point. I have found some kind of workarounds now with like pipelines are getting a little bit better and things like that. But still, I think that, even in a product like Databricks, you still experience that pain point and you create one off notebooks that sit somewhere and.
Just understanding the context of why you wrote that when you come back to it a year from now, it's really challenging. It's really painful.
Chris Detzel: So what if you don't come back to it, but somebody else does within the organization? Do they have access to that code or the sequel?
Noy Twerski: So that's exactly, I was about to add on of Mike.
Yeah. No, exactly about that. That another really big issue here is like the collaboration part, right? It's exactly what you said, Chris. If someone else needs to actually access that notebook, and you haven't actually saved it anywhere, that snippet of code that you use so often They won't have access to it, or even worse, they would write a totally different part, like SQL [00:09:00] code.
And then you would get two different answers, and that's what usually happens. Two people would ask the same business question. There's no there's no ability to actually reproduce that SQL. And then you find out that one person says you have 300 daily active users, and the other one would say that you have 500 daily active users.
And what's the right answer? And that's like a huge problem.
Michael Burke: So how does, this is a, I love that example and I think we hear that a lot in the data space, right? Of these mismatch on key KPIs or performance metrics, in my perspective, at least how much of this, how much of Sherloq enforces cultural change versus being a tool to help organize and implement these kinds of snippets, right?
Because to me, so much of this is cultural and I've gone through many tools that try to solve this problem in different ways. How does Sherloq address those issues,
Noy Twerski: so that's a really good question. There are two ways to go, right? The first way is definitely that culture is a huge issue at Sherloq.
And we really believe that [00:10:00] people need to understand that they have the problem and want to fix this problem to use our tool. And that's why we made it self served. We do mostly, most of our leads are inbound. Like people that understand that they want to fix this problem. So culture is definitely.
One way to go, the other way to go, and this is also super important, is that the product needs to be really amazing. And when I say really amazing, it really needs to give the people like a good feeling and want them, want, get people to want to come back to the product. So we do it in all kinds of ways, right?
We actually, a few months ago, finished a total redesign of the product because we felt it wasn't good enough. And we really took two to three months to redesign and build everything from scratch pretty much. And since then we've been getting amazing feedback, but the number one goal of that was to help people actually change their habits, right?
So we wanted the product to look really good. We wanted the UI to look good. We wanted the UX to be like super intuitive and we wanted. to be able to catch people where they [00:11:00] are and create like new habits and new triggers for them to actually be able to change their existing workflow, which I definitely agree is very hard.
So there's definitely UX play here for sure. Like it's super important, this type of product, by the way, that's why it's also a plugin, right? Because we want to catch users where they are. We don't want the context, which we don't want people. And that's like a headache when it comes to developing plugins.
You can only imagine. Wow, it gives us so many bugs, but it's super important. It's like a core value at Sherloq differently.
Chris Detzel: The one thing that sorry, Mike, but no, go for it. The one thing that I like what you said is right now you get inbound leads to, people using it. And I think that's key when you think of how developers work and people like engineers or whatever, the community around that stuff is big, building that community, they stick together and they'll tell everybody about their.
But I think that the opportunity over time with big organizations is that they can require their [00:12:00] teams to use a tool like this, right? Not to say right now you have the word spreading and that's the goal. But when you get into a larger organization, I think that, from a cultural standpoint is,
Noy Twerski: Hey,
Chris Detzel: this is the tool we use to save the code so that everybody knows what's being done and all of these things.
I think that's an opportunity to think about. As you continue to grow into these bigger organizations, right? Cause right now it's just like spots, people are usually you're in there. They hear from their friends or they look on Reddit or whatever, and that's fantastic. I love it, but to even just go to the big enterprise and have those big deals.
Noy Twerski: So I definitely agree on that. I think it's super important, by the way, 90 percent of our revenue essentially is only from big enterprise deals. Our revenue is from, yeah, most startups. So revenue is like one, one way and growth is from the, from like the inbounds, from the self serve motion, so definitely, I will say that in enterprises, because there are [00:13:00] more data people, the problem, and you can imagine the problem is even more painful.
And that's where people are actually willing to pay a lot more to get this up and running. So today when we work with like enterprise customers, I definitely agree that usually we would try to talk to the director, the data director, or at least the team lead to get them on board with us. And then once they have, they are on board and want the solution, then they help us like spread it out to they have like dozens or hundreds of data analysts.
So that's usually how I go. So I definitely, it's a really good point for sure.
Michael Burke: It's an interesting phenomenon. I feel like in startups, you've got a small team of really talented people, right? And as they grow, they add more people on person that's been there the longest, who's the most technical, can pave the way for a long time doing like 80 percent of the work, they're developing everything that domain knowledge only has to transfer one to two layers.
For a long period of time, when you get to three or four layers of hierarchy, you've got people that are onboarding, they're two months old and [00:14:00] people that have been working in the product for six years as principal engineers. And it just, that, that information never transfers down. And so you end up having this huge upramp and struggle for the newer engineers who are trying to get on and understand the process and the people.
And, I think tools like this are super valuable because they help track a little bit of that lineage and help you understand the context of what you're doing faster, right?
Noy Twerski: Yeah. So definitely. And one of our main use cases is like onboarding new users and new employees. And it's like a super interesting thing that we've noticed.
Is that those new employees, we were just yesterday on a call with one of like a user interview with one of the new employees and one of our enterprise deals. And what she told us is she's becoming like this champion internally inside her org because she got to know and it made her onboarding so much like quicker and easier just for her to be able to see like how other people do their work.
So yeah, so definitely it's one of our. Most favorite, use cases, for sure. [00:15:00]
Michael Burke: So pivoting a little bit away from the product, right? I think in this space, there is so much change right now. I think that, there's people on one side that are saying, why are we even writing SQL? AI is going to change everything.
I think that is an extreme and there's other people who are very much in this traditional space of data warehousing and like writing standard SQL queries that migrate into reports on a batch process. Where do you see the whole landscape shifting to with the boom of AI?
Noy Twerski: So the first thing that I always like to say when it comes to this to talking about AI and SQL and where it's headed is that something I think we cannot ignore is that AI is like crazy, we're just in the beginning of this crazy era, right?
And we can't ignore it. And whoever will ignore it will be left behind. So that's for sure. And going on with that, I'm sure that SQL and analytics will be at some point. Self served and democratized. [00:16:00] This will definitely happen. The question is how long will this take and how much organizations would need to switch switch and adopt new tools and new stuff to get this actually up and running.
So yeah, so I think that. How we look at it is that, and we can go a bit deeper into that, but how we look at like the self serve motion when it comes to analytics is that we're having people to actually be able to generate SQL and to be able to generate analytics. In a way that makes sense, you would need to have this business context and that's what makes it super hard.
So Chris, we talked about this like a bit before, but we're getting into the spoiler right now. That when it comes to, when it comes, it's very different than any type of code out there. In my opinion, I'm a very big SQL fan for sure, but it's different in the sense that it, SQL comes to explain business, right?
When you look at a SQL query, it actually is some person. [00:17:00] Explaining the story behind the business through code, and that's how I like to look at it. And, yeah, and that makes it much harder for anyone that doesn't have the business context to actually be able to generate it. Even if you do have the business context, you would need to know how it is shown in the data, right?
So if you, for instance, have a metric that's called, going back to the active user metric, right? So you need to know how each org actually defines that metric. This active user, and not only, even if you know how they specifically define active user, a user that signs up to the platform in the past three months and uses the core feature one time a week, for instance.
Then you would need to take this and translate it into how it is shown in the data. So what tables define this core value? What fields do I need to query from to get to this core value? So getting all of this inside an LLM and making it generate the SQL correctly [00:18:00] is very hard. It will get there for sure.
It's a matter of time, but it will take time. And it, and organizations need to actually build. Very massive governance and data management infrastructure to get this up and running.
Chris Detzel: I'm sorry, but I have to jump in here, but I think that you hit on something very important is, like today, and I mentioned this on the pre show, is that I can now go in there and to cloud 3.
5 or chat, GBT or whatever. And I can start building my own website through code. When I say code, I just mean like JavaScript, HTML, CSS stuff that I know nothing about, but if I can give it the right prompts and if I can tell it what to do, it will do it most of the time. Not perfect, but it will do it right.
But this is building something from scratch, right? And so. The beauty is that I've done it. So if you go to my website, it's called DFW Running Group. I built this whole training plan for a [00:19:00] marathon, for a half marathon, and it gives you a day by day training plan and all these things, right?
Through code. This isn't, this isn't a person that can't code, but I know we're talking about something a little bit more enterprise wide, right? You can build or before you can start doing that, it has to understand the business context, but I agree. It's going to get that.
And I think you're right. Is you got to figure out how that's going to get that when it gets there. Who knows? Because you're right. The governance, the. The things that companies will or won't allow the large language models to do and things like that. So I think it's intriguing and interesting and it's not far because now I can code.
I'm going to disagree with you a
Michael Burke: hundred percent. And the reason is, and I'm glad that we're having this conversation. Like what? So you're looking at a set of data and you're trying to get a KPI. Yeah. Let's say you're looking at, high quality users, right? How do you define high quality?
What information do you need? Take your own website to define what a high quality user [00:20:00] is, right? What attributes, if you see an increase month over month in these users, are you going to take a business action on it? What about seasonality? What about the geography changes in your landscape?
There's all this expertise that I don't believe we're close to having an AI be able to advise on yet to the degree that a business decision could be made effectively. I think that a data store could ask a large language model, technical questions and get technical responses back, but the business context of being able to build that story of this is how we got to the metrics that we're driving our business off of, and this is why we're going to go raise money, or this is why we're going to go double down in these areas.
That takes a huge amount of context that I don't think that we've been able to even figure out how to capture that information correctly. And I totally agree on that, Mike. And I feel that a year ago, people were more hyped about like text to SQL and SQL chatbots and people really wanted these types of solutions.[00:21:00]
Noy Twerski: And I feel like in the past year, people are starting to understand that this is so much more complicated than just like cool tech. I think we're getting like Again, I think we will get there, but it will take a lot of time. We're not close to it. And probably the way to go would be to start as you said, in tiny steps.
So first of all, getting data stewards to get correct answers, correct technical answers on their data schemes. Then the next step might be, once we have those technical answers, are we able to create like query or a basic query based on those answers? Then taking it a step after, breaking this down into like steps again, this is, these are processes that are going to take years.
None of us know how much it will take, but it will take years and enterprises would need to very well manage their data in order to create those those con that context and that those business metrics, like in a way that is well defined. Defined and connected to the data, right? So not only that way, by the [00:22:00] way, I will say like when it comes to Sherloq, we see Sherloq as as the first maybe like infrastructure layer to creating the connection between the SQL and the business context to a certain extent, right?
Because we have the SQL, the snippets of code, the very, like the most tiny snippets of code until like very large SQL queries. And then we have the title of the query. Which is very much defined and we have the description of the query, which people, which our users literally write there, essentially what they are creating, which is amazing for the organization, right?
Is this like a semantic layer, like a semantic layer on the go and they're creating it for themselves as they go and continue to use Sherloq and when it comes to these like text to SQL, which we all know need this business context, we are creating those connections, tagging it for the LLM to a certain extent.
So I think this might be an interesting way to think of it, but again, it will take a lot of time and we're not even close. I definitely agree on that.
Michael Burke: [00:23:00] I was thinking about this the other day, that a lot of these things and I'm, by the way, I think text to SQL is a dangerous path to take for many organizations because it will create siloed biased assumptions and answers.
Based off of people that don't know how to ask the right question. Not for everybody. Some people will do a great job, but a lot of people will ask the wrong question.
Noy Twerski: And not even the wrong question, I would even add on top of that, they would ask maybe the right question, but they wouldn't know how to break it down into smaller questions.
You know what I mean? To get the right answer. So that's like how I see it. Yes, for sure.
Michael Burke: So I'm finding this incredibly interesting right now. And I'm just going to bring this up totally off topic from Sherloq, but that. Claude Anthropic recently banned people from using AI to add any kind of context into their resumes or into their cover letter.
And I think this is where we're going to go because everybody can ask for a marginalized, generalized statement from an LLM. But what you're, what you really need and [00:24:00] what companies need to drive value is something that's slightly better than that. That's innovative. That's that takes in the context of working in that business for years to be able to find a KPI that's meant for that business, right?
And not just meant for that business, but meant for your specific customer base. Exactly. And I think that For your
Noy Twerski: specific product.
Michael Burke: What we're going to see in the future and what we're seeing already is that everybody is using these AI. Because it sounds a little bit better or it makes them move a little bit faster.
But in terms, we're getting very generic answers back
Noy Twerski: for a
Michael Burke: lot of the things that we do. And especially if you're not an expert in SQL or in building these kinds of business use cases, you're going to say that's good enough. Everyone's happy. But it's not taking into account all of the context and underlying knowledge that You know, experts used to come up with a solution for these problems.
Chris Detzel: Maybe that's what they should do is how do you solve these business problems of that, are so intertwined when [00:25:00] you look at all the SQL and all the code, it's all built by humans, right? But as time goes on. It needs to understand, like, where the problems are, how to write the code, it needs, I don't know I think you're right, that's going to be a very hard problem to solve.
Very hard problem. Yep.
Michael Burke: A lot more input, right? A lot more input needs to be gathered to get that right.
Noy Twerski: Exactly. Exactly. And a lot more training, probably, based on wrong and right answers. And again, starting from small steps, that's, I think, if I would need to guess, that's how I think. Wouldn't be the right way to break this down.
Michael Burke: All right, so three to five years out. I'll say three to five because I know that you guys are still early. Where do you see Sherloq, right? I think that there's a lot that can happen. We won't hold you to it. One year out, let's do one year. Yeah. We'll see you guys in 2026.
Noy Twerski: Startup life is definitely the, you have the most highs and lows, and one day you can feel like you're at the top of the world. And then the next hour you're like, Oh my God, this isn't going to go the right way. So that's my life. Yeah, for sure. No, [00:26:00] but as we continue, actually it does stabilize. At the beginning it's the worst and then it gets a bit better, but not that much.
Yeah, so going forward, what we're planning is really like diving deep into this like collaborative SQL repository motion. That's what we've actually been trying in the past, like year, all kinds of other features. We even had. To a certain extent, like we tried out like this SQL chatbot with one of our design partners and stuff like that.
And what, it always came back to our users telling us that the bread and butter and what they come back to every day and what they love the most is the repository and the SQL repository. And that's what actually makes their day much easier their work much faster and then more productive. So we're definitely doubling down on that and taking into account and taking into context.
that this again is like an infrastructure, a really good infrastructure for those AI capabilities. So this is how we're going. So we're planning in the future to have this massive, for each organization to have this semantic layer, right? The [00:27:00] repository, which is essentially like a semantic layer, again, a data catalog for queries and to give them the ability to use.
internally, of course, these queries on all kinds of applications that they would like when it comes to their LLMs and to their like AI motion. And that's how we're thinking of this because for the organization, these queries are priceless, right? They have like suddenly so much data that they can actually use to even create.
an internal data catalog, right? To create like this first version of like self serve motion. Again, it doesn't have to be text to SQL, but just the ability to give, for instance, product managers that do know SQL to a certain extent, the ability to search and to find their most relevant SQL. It can go a lot of ways.
So that's how we're thinking about this, taking this like a collaborative approach and taking like these saved queries and giving. Then all kinds of integrations and applications into the AI experience and models. Yeah.
Chris Detzel: It's like a tagline. You could say the data [00:28:00] catalog for your for your queries or for all your SQL queries.
I don't know, whatever. You said it. It depends,
Noy Twerski: exactly, it really depends. Taglines, what I've learned, is, are really dependent on who you're talking to, right? Yes, good point. So when I talk to users, The number one thing that they like to hear is like one place for your queries. That makes most sense to them.
When I talk to investors, I would say GitHub for a SQL query. That's what they like to hear. And when I talk to like data directors, so I would usually say data catalog for your queries. So it really depends on who's listening. Who's your audience.
Chris Detzel: Yeah. One
Michael Burke: more question for you and this one's a tough one, I think we said we wouldn't go too hard, but
Noy Twerski: let's go for it.
Michael Burke: What do you think is the, is like the next unsolvable question in the enterprise data space? I think that this is one I love to ask and get people's responses and perspectives on what's one thing that we are still going to struggle with for the next five years. Yeah. So in my mind, I think the space that we're working in not only SQL, but the [00:29:00] space that we are working in, it's not random that it's, there are so many tools and so many companies that are trying to save to help like organizations and this space.
Noy Twerski: And when this space, I'm talking about clean data. Like how do I organize my data? How do I govern my data in a way that makes sense? And why I think this is like a, such a tough problem is because this is a problem that nobody loves. Except for me but like my customers, this is a problem.
That's a real headache for them. It's not cool. It's not hyped in a good way, but it's just like a really hard problem to get people to actually figure out and solve. And you need really good tools that change people's habits to get people to actually get, to get people on board. To want to make this change.
Data and having clean data. I'm sure I'm not the first person saying this on this podcast, but having clean data is essential to actually creating these AI motions and these AIs and like using LLMs in a proper way. And it will only get worse and worse because people are actually creating more SQL, more data as we go.
And I. I really do believe that this is a [00:30:00] problem, of course, that's worth solving and worth building startups on, but definitely a problem that's going to be sticking around here for a while and will only get worse and worse once people actually need their data to be super clean and super tight to use it.
So definitely. I love that. Data quality is always the hardest problem to solve.
Hardest. It's the hardest.
Chris Detzel: You hear every data leader to every data person. That's it. Clean, crisp, quality data.
Noy Twerski: Exactly.
Michael Burke: Oh, and to add on to that too, and really extend what you were saying, for years, data has been thought of as a service based organization, right?
That we serve the business stakeholders. And I think that more and more you're hearing like data is a product, right? What does that mean internally? I think that means that as data practitioners, we really need to think about how do we measure success? How do we measure value? And these are things that we have not had to focus on for the last decade, right?
And as data becomes more integrated into the product ecosystem, into the [00:31:00] customer ecosystem, where do we invest our time? And how do we ensure that those pipelines and the culture around those pipelines and servability is really being attended to with a product mindset, product led growth mindset.
And we don't have that today in many organizations.
Chris Detzel: It's hard because think about this is that a person that used to work at company A now moved to company B. So their email address, maybe their phone number has changed, or maybe they move from, you know what I mean? If you're just looking at like contact data or whatever.
I mean, the data just was always having to be updated and how do you do that? And how do you keep it clean? How do organizations like this person used to be at this address and they get an insurance company and they're calling in from a call center. And they used to say they used to be at 1, 2, 3, whatever drive, and now they're at 4, 5, 6, whatever drive.
You know what I mean? Like, how do you keep that updated and clean, and how do organizations do that? How do you get that, it's always going to be a hard problem to solve.
Noy Twerski: Definitely, even exactly like that, connecting to, [00:32:00] to what Mike said, how do you even how are you even sure that you're doing it well enough, right?
How do you like define the metrics that make this successful, right? How like customers always ask us, like how, help us define what's the ROI here, right? What's the like return on investment here? Like, why should I invest in data tools? When I'm not sure what this gives to the business, right?
So these are questions that are going to take time and it's really dependent definitely on each business and each organization, but for sure it's a hard problem to solve and for sure it's a problem worth solving. And I love this problem because of the, because it's super challenging and I'm sure people like our audience here would, might agree with us.
Michael Burke: I hope so. I hope so. If you don't agree with data as a product, we need to talk. Come on the show. Exactly. All right. Anything you want to wrap up with Noi? Thank you so much for coming on the show. This was great.
Noy Twerski: Yeah, I had the best of time. Love talking to you. And I love kind of hearing your thoughts and hearing where you [00:33:00] think we're headed and also sharing what we think at Sherloq.
So super fun time. And thank you so much for having me here.
Chris Detzel: The last thing I would say is there anything that I, that we should have asked that you're just dying to get out? Was there anything there? Not really.
Noy Twerski: Not really, but self promotion, you don't have to show this with a bit of self promotion.
If anyone here wants to try out your luck, you can just hop on our website and try it out for free. So it doesn't cost anything and just give it a try. That's it. We'll show it. We've
Michael Burke: got a soft spot for startups. Absolutely. Thank you so much. Alright.
Chris Detzel: That makes sense. Thank you, everyone, for coming on to another Data Hurdles.
Please don't forget to rate and review. It's so important. I'm Chris Detzel and
Michael Burke: I'm Michael Burke. Thanks for tuning
Chris Detzel: in. Thank you, everyone.
Creators and Guests
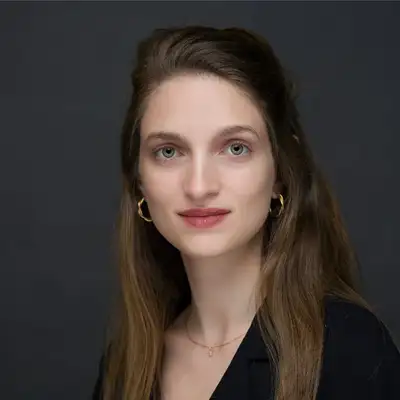
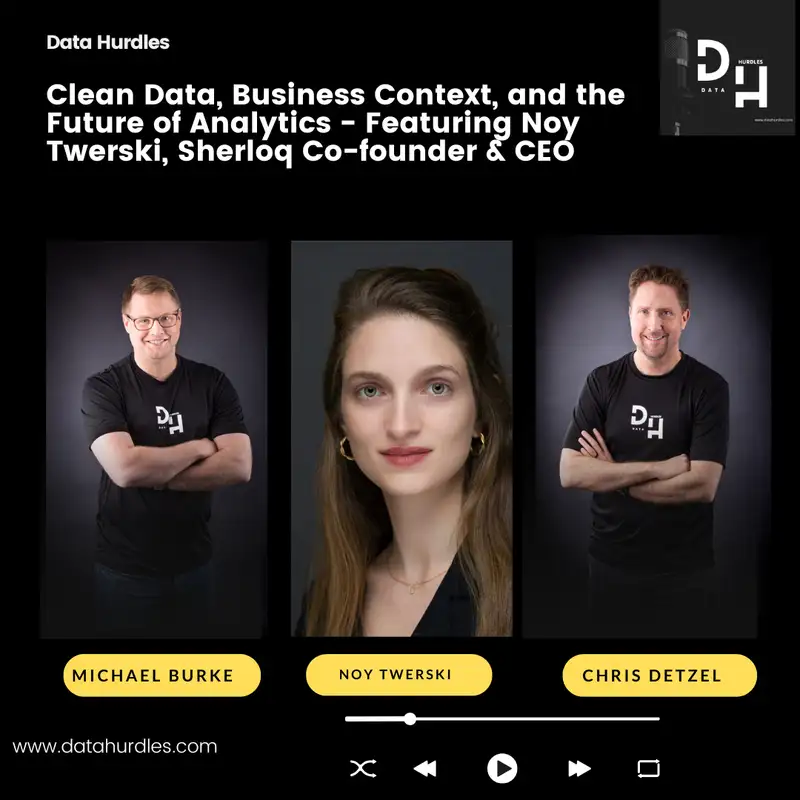