Data Mesh in Action: Challenges, Opportunities, and Real-World Examples with Willem Koenders
Download MP3Beyond Buzzwords: Understanding Data Mesh and Its Business Impact
===
[00:00:00] Hello, data enthusiasts. This is Chris Detzel and I'm Michael Kern. Welcome to Data Hurdles. We are your gateway into the intricate world of data or AI, machine learning, big data, and social justice intercept, expect thought provoking discussions, captivating stories and insights from experts all across the industries as we explore the unexpected ways data impacts our lives.
So get ready to be informed. Inspired and excited about the future of data. Let's conquer these data hurdles together.
Chris Detzel: Welcome to another Data Hurdles. I'm Chris Detzel and
Mike: I'm Michael Burke. How you doing, Chris? I'm good. How about you? Good, it's been a while since we've done a podcast. I think we had a little bit of a hiatus there for two weeks or so. Happy to be back at it.
Chris Detzel: Yeah, it's exciting, and guess what? We have a guest today, Willem Koenders
willem, how are you?
Willem Koenders: Yes, sir, I'm doing very well. How about you guys? You
Chris Detzel: [00:01:00] know I'm in Dallas, and it's gonna be about 89 degrees today, so it's nice and cool here, so it's exciting.
Willem Koenders: It's dreadful where I'm in, in Washington. D. C. There's that. Are you getting hit by a lot of rain right now?
Is that right? We were. I don't know if it's related to what's happening more south of us, but it is positively dreadful. Anyway.
Chris Detzel: And Michael's close to, or he's in Massachusetts,
Mike: I used to run the golf before this, so I had a great day. I played nine holes, so I can't complain. Friday is a good day for me.
Chris Detzel: Tell us a little bit about yourself, what you do and those kinds of things, and then we'll kick it off with our topic today.
Willem Koenders: Yeah, sure. Thank you, Chris. Yeah, I've Chris, you and I talked before, but like I've worked for pretty much my entire career in data, we started out as a corporate strategist, but I very quickly realized the role that data plays for companies to sustain a competitive advantage.
Had the good fortune to work with a great series of chief data officers that kind of learned me the ropes of how this works and what matters and how to do it. Spend time in the U. S., Latin America, Africa, [00:02:00] Europe, Asia, actually, all either, permanently just, living there for a while or or projects.
And yeah, so now at ZS Associates, I lead, I'm a global data strategy leader, so I lead a lot of consulting engagements around data advisory on everything from like the highest level data strategies down to Rolled up sleeves implementation of different programs and projects.
But as you notice today, it's also just my passion. So I'll talk about it and I'll read about it in my free time as well and talk to guys like you. Yeah, good to be here. That's pretty awesome.
Chris Detzel: One of the things that we want to talk about And I've sometimes it gets controversial around this topic around data mesh and one of the cdos and michael can attest to this that we talked to a few weeks ago a month ago was like, you know He just didn't believe in data mesh at all.
And the reason is because he felt He doesn't know one person or one company that's using it doing it never seen some use cases and he goes he can need to Talk about this But so we'd love to get your take one and you know talk walk us [00:03:00] through what you know the concept of data mesh, how does it differ from like traditional data architectures and data lakes and data warehouses and things like that?
Willem Koenders: Yeah, for sure. For me, maybe just to start off with, what is it to begin with? For me, the data mesh to me, if I, I just see like a net, like an actual net with threads, like appear in my mind with like strategic data in there. And then we know How to make that strategic data available internally or externally, but in any case to those people that truly need it.
And at the end of the day, that's really what it's all about. It really a very strong concept. The concept that very strongly relates to is this idea of domain driven software engineering, domain driven governance of data, right? So that instead of you, having this, you just mentioned it yourself, right?
The data lake or data warehouse, like being very focused on having this big container or this big, [00:04:00] platform where you get all the data. It's really not about that. It's really about Yeah. Yeah. Connecting the dots, right? Creating that net across the organization. And for me, And maybe some listeners may take offense to this, it's a little bit less about the technology sometimes, like for me, it doesn't matter as much, what cloud native architecture, if any, it really is on for me, it's the part that I'm looking at more is is the governance around it is that kind of how do you mobilize it?
What are the concept, the roles, responsibilities? How do you maybe change Different people already have roles in organizations to be more aligned to this type of, data democratization. At least that's for me. And I think sometimes, like, where some of the challenges come from, and I don't know the respective CDO you spoke to, right?
But it's it sometimes over promises it, right? Here's the mesh, here's your data next quarter, it will all be there. It's not there, right? That's not how it works. It's a lot of hard work that will come with it, things like data ownership and et cetera. So [00:05:00] probably that may have something to do with it.
Yeah.
Chris Detzel: Why do you think it's gaining so much traction? And what are some of the challenges that are being addressed with it?
Willem Koenders: One of the main reasons is that the quality of data is still a problem. We've talked about this for decades and depending on the, the company in the sector in the industry, but like the quality and the availability of high of data remains a problem that has several challenges.
Yeah. Reasons. One again ties back to, these data lakes and, data warehouses where the technology at that point of time just seemed to advocate for a centralized kind of provisioning of that technology and like a lot of companies, even they know that they might create a data swamp.
It's just too tempting to pump it in there. And so I think that's just like a big part of the reason. Did
Chris Detzel: you just say data swamp? Yeah.
Willem Koenders: I may have. I know
Chris Detzel: so many different terms. Anyways, go ahead.
Willem Koenders: Yeah. And [00:06:00] so it's if that's the case, then then, carving some of that data up by domains and starting to drive ownership instead of indeed, like dumping everything in this swamp where there's nobody really.
Owning it like that's actually one of the key reasons. And so there's driving of ownership is almost one of the it's not even necessarily for me. The interoperability technology that most people think about of data match that is so foundationally potentially transformative. It's that ownership, right?
That you now have technology that matches that other governance. Prerogative that you have to this consideration to drive ownership on a domain basis that I think it's very important. And then, why, like, why does it gain traction even more? So maybe now while we've got yet another technology wave right now, it's Jenny.
I was something else before. We quickly want all of this data to be available. queried to help these use cases. But guess what? It's still not there and available. So that just keeps building.
Mike: Can you give me some examples? [00:07:00] Because, I actually think that to me, and I'm sure a lot of people will disagree.
I feel like this is the tabs versus spaces debate, programming, these cultural ways of setting up process are what are differentiating these frameworks to me. A lot of it is just about how you're thinking about process and control and communication. When you're implementing as a practitioner, Yeah.
a data mesh into a company that you're working with. If you have a dysfunctional company that's really struggling with some of these fundamental challenges, how do you go about Remediating that or what are your recommendations? Because, having gone through this a few times with different companies, right?
And these transformations sometimes, rarely, but sometimes they work really well and everybody takes to them. There's an alignment, but many times it falls apart. And I'm always curious for somebody on your side of the table who's coming into these environments and you need to come up with these plans for transformation.
What steps do you take? Okay.
Willem Koenders: Yeah, for sure. So [00:08:00] maybe a couple of things that come to mind for me is maybe the how and the where a little bit. And so maybe let's talk about the how a little bit first. So data mesh again, almost by definition is domain driven, right? You will not even the most leading companies will not ever You know, have a functional data mesh across each of the all of their domains at the same level that there's also no business case for that, right?
There's something about actually identifying the domain to figuring out what's the 123 key wants to start out with? Because, that other domains will need to consume the data from that is a very cliche. Cliche recommendation, but it's true, right? What? Start small, figure it out.
What are the two or three main ones? But then no matter which domain whether it's the first one or the seventh one to have some sort of a playbook. And I think with data mesh and just like other data governance concepts, it's very easy to. Make it [00:09:00] extraordinarily complicated to have a lot of different rules to make very a lot of tech speak in there, like what's actually the playbook here?
What would you do? What is, definition around what a product or an asset is? What is the data owner? What do they do? And then a role and responsibilities in three or four bullets, not 25, right? What are the key things that you really need to think about? And how do you enable them? I think those are two of these kind of if I know where I'm going to do it and I'm going to how I'm going to do it that already gives me two tools and now across this have some sort of portfolio view of progress like can I see how many nodes I have in this data mesh, how many like functional certified data assets and products I have.
Where I'm very strict in the beginning around the business case, like, how do I calculate the ROI, very tough thing to do for data, like, how do you drive the revenue uplift or the cost decrease and risk mitigation. But if you have a view of a portfolio of [00:10:00] 3, 4, 5, very well functioning debt assets or products, you'll notice that the rest of the company wants to have part of that too.
That's at least the pattern that we've seen. Some of the companies that are able to have a good start and then take it from there.
Mike: That's great. Yeah, I've noticed, too, that like in larger organizations, it's so interesting because the bigger the organization, as you try to make some of these transformations they take a lot of time and buy in from a lot of different departments, right?
And even when you start small and implement some of these use cases, it can be really challenging to toe the line. Even if you do have success to pull the rest of the organization along, how do you see the long term, more proactive steps of implementing a data mesh, right? Because I'm assuming a lot of your customers are fairly big customers, right?
So this is this could be a 5 to 10 year transformation, for some of these larger organizations if they're really working towards a centralized kind of unified system.
Willem Koenders: Yeah, I think so. I think they're just having A view or a backlog of [00:11:00] what these products or assets are really helps and to be very deliberate about early on what are these kind of logical clusters of data that can eventually be turned into an asset Or a product and to have a deliberate effort to schedule them out and to deliberately say yes or no To them that really helps.
At least from what i've seen to so that you have this portfolio that I mentioned earlier not just across the Data assets and products that are live and generating value right now, but to also have it about like up and coming here's what's coming for this year. Here's what's coming next year.
You can compare it to, some people use a supermarket example. Others use different ones. Like some of the shelves are still empty, but there's a little sign up there and say this is coming this year. That, that for me at least helps to be proactive about it, to help the resourcing and as long as you're like very early on, very deliberate about both ownership, like who actually owns this, someone's job, it's someone's job to represent this product or asset once it's prioritized, and then two, for that specific [00:12:00] person to be very deliberately articulating the business case.
It's where in the longer term, that should eventually find its way to the shelves may have some reprioritization as we go. But that's at least where you can go. And just now we went through it last week in the workshop with let's say globally leading technology company. And, we had a list of probably 30 40 critical data sources that eventually they would need.
And what we eventually did is we created this Heat map of source, of sorts, what are these critical assets and products? What are the use cases across them? And then we pick five eventually that would be able to drive a little over 20 of these use cases, almost just these five.
And so that's a way to be very powerfully saying this will have to be done in the next year. If you truly want to be serious about those things that you actually already say in your business strategy,
Chris Detzel: I have a question real quick. Sorry, Mike,
Willem Koenders: but
Chris Detzel: I'm trying to envision this, right? As it's just a [00:13:00] practical person.
That's not like your hardcore data person that talks about it. Meshes and swabs and things. So is that kind of Okay, what does this mean in real life as a business owner? So for example, and i'm just making this up, but let's say, you know Your data teams have all this data in a centralized way But then we're you know using the data in our own ways as a business.
So marketing has some stuff, this team over here has some stuff and can you talk about that and like real life how somebody like me would understand that because I'm trying to understand it
Willem Koenders: better. Yeah, for sure. So if I take a real example that maybe probably existed 90 percent plus more of industries is if you take a domain like customers, right?
The customer or patients or accounts or whoever you're serving. Like it tends to be the case that type of data is required throughout the organization, because, for example, you're if you have a call center or some sort of communicational team they need to be able to call them up and have accurate information to talk to [00:14:00] them about whatever products or services they have in the past or still.
Buying from you. Like you need to have that available somewhere as an example. So what you'll get to is like in a domain, like customer organ patient or whatever, it ends up, whatever your equivalent to that is, is that becomes one or several assets. They tend to be tied to an operational platform, like a CRM or an MDM or something like that, that you think about that as a product is now, if I'm.
In these in the operational processes are part of the business or function in the organization that creates or changes this data. How do I now do this with keeping in mind the actual use cases that are using this? So I have a quantified understanding of. Hey, if the marketing team doesn't have an email address, they can't actually run this campaign.
And so that campaign is set to drive half a billion dollars of possible revenues. So they don't have to be precise. Actually, as long as the magnitude is roughly okay then you'll be fine in terms of driving it as a product. [00:15:00] That's one example of. Of the most, probably the most common or the clearest example of starting as a product.
Now, the nice part is that all the domains depend about on each other. It's not the case that, you have two or three domains who are serving all the others. There's a reciprocal nature to this. And that's, I think like where you, the same thing as everybody in a stadium keeps sitting, everybody has a great view.
Same thing here is everybody lives up to their own responsibility. You got in a very nice environment of being able to truly exchange data. Now, it sounds easier saying this on a Podcasts like this, like we just talked about it, that, that, that's rough. You have ownership, people are busy systems, maybe, old and all of these things, but that's at least the thinking behind it.
And so that you truly own that now we can take any sort of analogy, right? You can do the kitchen is used a lot, right? So that you're a chef, you're trying to build stuff of, you're trying to create dishes and to create the food. Somebody is in charge of giving you the carrots or the onions, right?
That, that's effectively how it's all about, right? It's everybody is. You're creating something for someone else. You want to do that up to a set [00:16:00] of standards that you have very clear, like in a real world example, like that's what it's about. You give me the sector or I'll give you some thoughts on you know what, how it could be, but that's effectively how how it would work.
Mike: I think the really interesting thing, Chris, is that, it's not just getting all the data together for customer, right? It's what is the customer count like aligning on the kpi's what meal tastes good for the kitchen analogy, right? Like the whole business has to align behind these definitions Which almost always there's ambiguity and people are reporting out those numbers differently it's always happening right like you'd think there would be one customer account or one revenue number, but there's not
Chris Detzel: I remember this guy joe dos santos.
He was at the time the cdo at Qlik, but then now he's moved to workday but he said When I was at Qlik, there was like five different departments that thought about customer and we all thought, Oh, one person was like on the account level and one team was on the contact level, and he goes, we had five different [00:17:00] views of what a customer was.
So we had to define that first. Nobody knew or they knew what they thought the customer was, but none of us had the same definition. So it's a good point.
Willem Koenders: I love, Michael, what you said about like the analogy here about with the kitchen value may pull that a little bit further is it's not just about knowing the dishes that they want to make.
There's also realization. And this is very core to thinking about it as a product is like the products of this year may not be the products of next year, right? Like cars and it, whatever clothing, it all changes. And so it does data. And so in this kitchen analogy, maybe it's vegetarian dishes that this particular company needs to start serving up, or maybe, they have a particular ingredient that no longer works for them, or there's the carbohydrates need to be taken out, whatever it is like that is very relevant to data as well, especially also customers, right?
Their demands are changing absolutely rapidly. Also privacy concerns, how they want to be addressed. It's like that, like being able to have an active like product oriented process around this is critical. It is, I would say, no, it's no [00:18:00] longer enough to say, I'm going to invest five or 10 million this year and platform and I'm done.
That is just not how it works. There's just, what data are you actually interested in? What's the data model behind it? What are the business requirements on that? And making sure that you keep track of them as well as as time passes.
Mike: So another really interesting question I have, and I love the kitchen analogies, but when we go into some of the more complicated pieces of data mesh like governance, as an example, from at least my perspective, and I haven't done a ton of data mesh, but I have done some work governance becomes something that's really challenging, right?
Because you essentially are giving ownership of data to the organizations or the subsets of organizations within your business. But then you have to You're also trying to bake in things like self service or governance and how does that work like when you go into a large organization how would you implement governance that was done so in a meaningful way where there is still some layer of control over who has access to what and how that's [00:19:00] being audited.
Willem Koenders: Yeah, for sure. Now, maybe first recognizing that there's different types of governance even, right? So there's governance around, the security of the data, which is an extreme, an example of where you actually want to continue to be pretty strict, like you do not want to, because a lot of domain driven teams will not have definitely not initially the same maturity across the entire stack.
So that's going to be one that you are going to want to have a common kind of, process instead of protocols around that. However, like giving access would be something you would have the domain team do, for example, right? So that is an example of where you can that one's actually fairly standard and probably people won't fight over as much like the governance around the quality of data is much harder.
And so that and that's where like a cultural. Change may be required as well. There may be a case where a central team, maybe finally, had some standards in place, some control, and they're now almost told to let go. That's no longer your problem. That's now going to these domain driven [00:20:00] teams.
That's something you need to address and talk about, like, how it works, why it works like that. But on the receiving end, it's actually important. It's almost the inverse image of a similar kind of culture change that requires like a lot of people might be longing for it because the data has been not up to what they've been wanting for.
But more commonly, once you actually try to find owners, like a lot of Business teams, they just, I've heard it so often. Just give me access to the data. It's that's not really how it works. That's one part of the reciprocal relationship, like who's on the other end, like actually owning it.
And so when you're trying to find an actual individual who signs up for, I own it, that is hard. And so that is something where. A lot of things in talk, fill the remainder of the hour with this, right? Like how you can work on that type of transformation. But a lot of things come down to helping them understand that it's actually not a new responsibility, right?
It's maybe you didn't know that you were already in charge of making sure that the data was protected and you didn't do [00:21:00] anything strange or unlawful with it. That was already a core responsibility of yours all along. It's we're just going to ask you to do it a little bit more formally.
And then the other bit is it's enablement. You cannot just, I've seen that too. Hey, here's a new set of policies and and standards and good luck. And that doesn't work. You have to help them. Like after that playbook I mentioned earlier, what are those key two, three things?
And really two or three things, not 10 or 15. What are those key few things? And ideally, how can we tech enable do this? Like you, Please do not get into any manual lineage efforts anymore. Manual efforts to write data quality rules. There's tooling who can do this now. So help them do that.
Help give them the tools to quickly take the first strides.
Chris Detzel: I feel like it's just so complicated. And like, when you think of, so as let's say a business if I own some of that data or whatever, but all these if I'm new to an organization and I come in, there's all these things that I'm gonna have to do to try to get data.
Like I, I'm at an organization today and I'm building something brand new, and need all this [00:22:00] data first. I got to figure out where to go, who does what. And there's five people that do these things, and I'm like, I don't like, I need to do start getting, I just need a list of these kinds of customers.
Where's that at? It's hard to get, I was like, why is that hard to get? I should be able to know, and maybe that helps, once you start having this data meshes, you have the centralized area that has the data, but then there's these different owners, like for me, I have to create my own stuff, and hire out a company to do this.
It's just, it feels complicated to go into these somewhat big organizations. I can't imagine a 50 billion organization or something massive, to do some of this stuff. Setting that up, it seems very difficult, in the At first, it was at a massive organization, then two is, if you're new, maybe that actually helps.
I don't know.
Willem Koenders: And as a part of like your I guess the experience you're describing, it sounds like you have a fun time or have had a fun time in the, in while you enter. But there's a part in here that we haven't really mentioned yet [00:23:00] that if you do it right, a data mesh can really it comes hand in hand, namely some sort of marketplace or catalog of the data, right?
Again, not all data everywhere, but across that net that we now start to build across the company. What are the, what are those key nodes? What are the key cities? Let's say on this neighborhood map. And what are the directions to get there? At the end of the day, That's where you want to get.
But like before, if you go to any of the vendors and maybe sometimes we do this ourselves to like in a demo that looks very easy, right? Here's the assets and the products, double Qlik order added to my cart and boom, here it is right behind the scenes. All these things we just talked about do need to come together, right?
So what data is it? Do you have an owner in place that has made sure that it's fit for purpose? Have you, do you have a data access process in place to check that the respective parties should have access to it? Does that access process itself not take three months to get through?
Because a lot of companies, counterintuitively, who've got, had a lot of, Success on the technical [00:24:00] side of enabling this data democratization now are getting a push from their privacy and legal organizations saying ho ho, right? That's we now have PHI, PII, a lot of privacy concerns, and it may be a breach or two.
And you actually have to go back and figure out how do I factor all that in? So
Chris Detzel: yeah, so what do you think, as you look at, you mentioned some case studies and if you want to mention another one or two, it'd be really good. But how do you know? And how do you measure like positive business impact?
And what are
Willem Koenders: the outcomes? Like, how do you do that?
Chris Detzel: Yeah, for me,
Willem Koenders: that's cool. Yeah, for me, that's just for me, the most dominant way to go about it is that portfolio of assets. It has to be that. So it's you may have seen that's like online. I've written about and talked about this and another analogy.
Here we go. But now it's like real estate folks. So if you want to. Contrast and compare how you can drive value off of kind of data assets. It's maybe something a little bit vague, right? But if you think about real estate, like a company's new office buildings, train stations, it's much easier, like to think about what do you need to [00:25:00] do for them to, so for them to be fit for purpose and to drive value, namely they need to have an owner because if not, they're going to be dilapidated.
Like no one's going to care if you can't get the profits out of them. You need to have people who are in charge of the quality, roughly not like you don't have to like. Clean every hallway every day, but you need to do enough, et cetera, et cetera. So if you were to compare something like that to a data mesh type of organization where you're going to start seeing these nodes in the net, it's those nodes that drive the value, right?
So those nodes, by definition, should be managed and designed as a product. They have value statements and hypotheses against them. And that's the story that, at least in the examples that I've seen, you can most powerfully. Tell so, so compare that to, I've tried to do this too in, in past years, I invested 2 million in said data data catalog tool, or, a million and a half in this data quality tool.
What actually was the ROI very tough to do? It is a totally different story [00:26:00] to say now this kind of landscape to that together, we have 17. I'm just making it up, right? Data assets or products, which are tied to 32 use cases. And each of these have an owner, right? So the assets and products do, and the use cases do.
And so these use cases have value statements that, that should be cumulative, where you can say, hey, these 17 assets in these 32 use cases jointly drive over a billion dollars of revenue. They have helped to decrease 300 million Of a 300 million in cost, they've removed, over 100, 000 manual steps.
That's the type of story you want to tell. You do not want to talk about the net or the nodes. You want to talk about the strategic data and the use cases. That's also the most fun, right? Because that's when you actually get to the app, the business side or the functional side of your organization.
You're going to have very, Kind of engaging conversations about tell me truly, what's going to change if we do this data better. And that, that's the, I think it's the fun part actually as well. You're not, I think maybe there's an [00:27:00] opportunity for a lot of very savvy people to get out of that buried back office kind of operations or data team, and like truly get out there in the wild with the people who actually, sell the products and services and interact with the customers.
Mike: Chris, I'll give you a fun story too. When I was working a lot with sales reps, right? This was one of the hardest problems. Sales reps, they have the opportunity to provide you some of the best quality data ever, but they don't normally do it because they're not incentivized to do it. And I worked really hard to build a strategy because all of my work that I was doing for this one marketing and sales campaign using AI required high quality sales data required, BDs to put information into sales force.
So we started actually coming up with an accreditation program where we went to the top of the funnel and said, okay, if you get high quality data and we're able to make a conversion based off of that data using a I will accredit some of that to you. And that was one way that then everybody at the front line was like, Oh, okay.
We're going to actually get something out of doing all of [00:28:00] this. But it's it is really hard to weave those lines between different organizations that are incentivized by completely different things. Most people don't really care about that quality of data. They should, but it's not their priority.
They're not getting compensated on it. And so they're not going to, why would
Willem Koenders: they love the example? I think yet another thing that two things that come to mind is I just having me. A passionate kind of positive mentality about like, how am I trying to drive this? Change that. I don't know how you did it, but that's like, how can you be creative and try to make a change?
That's so that's almost like secret, not so secret, ingredient behind a lot of this data management work that is otherwise so dull because just, it just happens to be in the second part is process discipline as well, right? You can not ever. You cannot solve for that.
AI can't either yet. And so I love your example as well, because like you just have found a very original way to fix the process discipline, right? Like where it's actually being created because like garbage in, garbage out.
Chris Detzel: So if I'm starting out, I'm going to a big organization and I want to start thinking [00:29:00] about implementing a data mesh concept and actually do it.
What are, help me understand where should we start? Where do we go? What are we doing?
Willem Koenders: A couple of things. Like first, I would try to figure out, are people already bought into it or not? So like literally the highest level of what is it or not? If they are great, you can go to step two and be a little bit more tactically, around the next steps.
But if they are not like, let's just make sure that we established a business case here, like a little bit, the chat we've had here, have that one with the right people in your organization. And this is not yet another, governance heavy type of, you Program with like uncertain benefits, like there's something that should truly help you.
And if it doesn't, then almost like just talk about why not what are the things that are not working? And how could that help you that may be 1st 2nd, you get a little bit more into the logistics, the tactical steps around this depends, obviously, enormously sector, do you have certain technology regionally spread those types of things?
But I would come back to some on [00:30:00] Relatively simple depiction of what does this mean for us? Like, when we say data mesh what are the components that are in here? And how does that translate to some sort of reference architecture of sorts? These, because the whole point of, at least in my maybe simplified dataset.
Interpretation is like how can we make sure we almost start speaking some of the same language everywhere, right? And I have so what are those languages? And if even if you pick 2 or 3 languages what are the different translators between them? Having a single page that kind of is able to summarize.
Hey, we store data in. Each of these five or six types of buckets or containers, these are the three or four key interoperability or pipelines, that really helps. It is, a menu of sorts. If anyone now wants to start building something new, take a look at the menu. And if there's something not on there that should be, okay, we'll have a conversation.
But typically it tends to be there.
Mike: Chris, I think also you should think Really long and hard about what value [00:31:00] are you going to be providing all these other people that you're coming to ask for data for, right? Like the best data people that I've ever seen are incredible salesmen salespeople, right? They can they know how to trade priorities to get Things done because at the end of the day, everybody has their own initiatives.
I think that's why, yeah, exactly. Report exactly. And that's why I honestly, I believe that companies like ZS come in and they do such a fantastic job is that they bring in. An authority figure to come work with multiple stakeholders and find alignment in the sequence of work that makes people happy and gets things done.
And that is really hard. That's a full time job. Most people don't realize how much negotiation just for you to get marketing data or sales data into your platform or whatever you're building. That's really challenging, right? And that's a lot of work on both sides to set something like that up.
And they have two years of road map, right? Every one of those departments has two years of road map that you're competing against. To get that data [00:32:00]
Chris Detzel: are there specific industries willem that you look at and say these are the ones that are Doing more of the data mesh stuff than another or is it just all across the board?
Willem Koenders: I think it's pretty much across the board. There's definitely like levels of maturity in there So I spent a ton of time in financial sector A long time back. They've done it for years and they called it something else before, just, it's data mesh. It's like a lot of the concept are similar and things like that.
I spend a ton of time, for example, on medical technology, like they're starting now, like today for them. It was in the past, just taking two examples, like having a great product sufficed, like that was just leading a lot of their growth just came from. acquisitions, like mergers and acquisitions even.
And so like having a fantastic product, which is what drove growth. That's changing now because the products for just to give you a specific example, the products that they put out there actually themselves start producing data. So that becomes something to take into account, but also like across the different product portfolios, like the customers and accounts become [00:33:00] much savvier as well to figure out negotiations.
And so these companies now need to start, their commercial organization needs to be served with the right data around what are actually our products that we have, what are the pricing strategies what is a very complicated customer landscape in terms of, negotiation groups, the hospital, the healthcare practitioners, like they now recognize having, not having invested in that in years past that they need to have these data assets and products in specific areas.
And through that are finding their way to. Data mesh being the enabler, the foundational enabler.
Chris Detzel: Something that Mike always says, when you look at engineers and architects and things like that, a lot of times they're working on their own silos and doing their own things.
And it's just not really, they don't work well together necessarily. And Mike, keep me out of if I'm wrong, but I've heard you say that several times. But how do you see that role of the data engineers, architects, product manager, [00:34:00] etc. Evolving in that data mesh environment. They don't already greatly together.
Is there some things that you see? Changing or
Willem Koenders: I think so, a couple of things that would come to mind, like you may actually have a defined role that becomes more stronger, like a chief architect of shorts, like where you have an actual role across everything. It's you're one of your job descriptions is going to be to drive the the consistency and the coherence across the different domains.
I always love the connection with like broader non data kind of enterprise architecture as well. That's a very. Often neglected, connection to make because the enterprise architecture is very strong connections with business ownership and all that. Then low, lower level, like I would say, like more granular roles that are out there.
Them starting to think about data as a product here as well. So you may have an actual, they might not actually become the. The data owner or the data asset owner that tends to be someone who leans towards or sits in or reports to the business or the function, but they still need to think about that as a product.
[00:35:00] It's no longer anymore. I do pipelines in, I don't know, a power center or something like that. There's, there needs to be, how do the things that I'm putting together contribute to this becoming the best product that I can deliver to my consumers or customers. And from that perspective, also a little bit less.
Gatekeeping a particular system or technology to enabling self service. Again, that's what it's really all about, right? So and if we take that another step further, then things like, specific automation technology, orchestration engines, I guess a certain awareness of cloud native architecture as well, just become very helpful as well.
Depends a little bit on the stack you choose, but those are not new to the engineers. But I think from a perspective of enabling the net the mesh, something that will become very helpful to them.
Mike: Yeah, I love what you said there. And Chris, I have used that term a lot, probably more than I should about data and engineers working together.
But what it really is comes down to the same thing. It's communication on goals and [00:36:00] KP eyes, right? The engineer is building features. Why should they add in tracking telemetry data? Why should they worry about, machine learning offsets and how the changes in their code base are going to affect something in the data space?
And In successful companies, what I've seen is exactly that, right? If you have really good alignment on your goals, really good communication on what you're trying to accomplish and you work together, you're not siloing your KPIs, you're not siloing, the attribution of those KPIs, and you don't have grandstanders pulling up their one highlighted project, you get to this point where you build trust and everybody wants to see you succeed.
But that. Unfortunately, it's hard to do, right? It's really hard to build that trust within an organization, especially if there's been past tensions.
Willem Koenders: It sounds like you're listening into my program right now. That is exactly where, you have a very strong initial The enterprise technology company, like they are set on bringing some of these things to the business.
The business has been frustrated with the past couple of years, have invested in some of their own [00:37:00] resources that have these types of skills. Now, it's not working. It's not been working. So we're coming in to help try to figure out, how can you find a way forward in here? And you have to, what you're saying that there's no trust.
Because the business folks, the commercial IT organizations cannot operate without the operation, the platforms and the corporate data, enterprise data that the enterprise technology company organization would look after. But also like very specifically, like there's methodologies out there. That help you work together in a cross-functional squad, or whatever you call it, right?
You create the epics, the user stories where people actually report to different bits in your organizations, but are brought together in a kind of in, in a different team, in under the, let's say, the supervision of a product owner. But like for the actual current leaders to fully buy into that is a leap of faith in a lot of different times.
Didn't agree more.
Mike: Yeah, absolutely. And it's so hard. It's so hard to get there. But when it happens, it's magical, right? It's this like incredible. Like [00:38:00] movement happens at a rate that you'd never see anywhere else. And whether you're using data mesh is a great framework. I think that, I'm agnostic on frameworks, but I think that when you have that communication and that trust and that responsibility deviated in a way that's effective, it's just, it's incredible.
And Chris, honestly, going back to community, where you are, like, look at what you're really doing. You're building trust between customers and you're providing them education so that they can do more on their own and they can feel more connected to your product. In the same way, like when you have high quality data, you're doing the same thing is internally.
You're bringing people together, you're connecting them, you're allowing them to accomplish their goals faster and on their own. And that's really like a big, I think, differentiator between how a lot of people view data, which is a tool to answer questions. And that normally becomes much more of a siloed environment, which is what, where all these frameworks break down, right?
And why I think they get a bad rep and a good rep.
Chris Detzel: Since we look towards the future, both WIllem and Michael, whatever what does the future hold [00:39:00] for data mesh? Do we expect it to become like this dominant data architecture for big organizations or something else?
Willem Koenders: Michael, you first or me?
Mike: Look to me, these problems are never going to go away, right? Because inherently they're driven by culture. They're not driven by technology or code or languages. Yeah, so like anything else, if your company has the right culture and the right trust, you will find whether it's data mesh or another framework, a successful way to operate.
But if you don't have that, even AI, like nothing is going to fix these problems. Yeah,
Willem Koenders: I think for me, some of it is about the definition maybe of exactly what is or is not a data mesh. I sometimes I hear examples of alternatives, but I hear all these components like domain driven ownership, interoperability, it's data mesh to me.
It might not just not be, but like those, if we talk about data mesh and it's slightly looser interpretation, again, about main driven [00:40:00] software engineering, domain driven Governance, like having the most strategic data be discoverable across your organization and accessible, subject to a set of, standards and enablers, then, yeah, I think that is the dominant way that especially larger and more complex organizations, you will have to adopt something like that.
Doesn't mean that your entire organization and every team and every sub domain will need it. I think it's probably. More often than not, like less than a third of the domains that really need it, there's a couple of others that you're probably perfectly fine maybe with a centralized team or even a platform based, team, but I think the larger and complex organizations will naturally kind of evolve towards this this type of technology and pattern.
My hope is that what will change is how some of the tooling that we have is going to make that a little bit easier still, because some of the bottlenecks for data mesh, again, it's not the pipelines, right? It's not the, being able to store the data, like some of [00:41:00] the bottlenecks are still like, do I know what data I have?
Have I quality controlled it? Do it. It's things like that where I'm it's almost I don't want to bring the buzzword back in, but like where something like Gen AI can really help, right? Sometimes they're getting you the definitions, some of the workflows automate them. I just hope that will make this easier and more attainable also for companies that don't have, 10, 20, 30 million budgets to try out how it could work.
Chris Detzel: Data mesh isn't a buzzword. It is, yeah, I guess too. Yeah,
Willem Koenders: yeah.
Chris Detzel: Did we miss anything? Did you want to touch on something that we just missed? Or do you feel like we covered what we needed to cover today?
Willem Koenders: I think so. Yeah, I'm curious what people's responses would be and if they feel that it was helpful.
If there's like particular, practical Michael, you raised a couple of them from your examples, but I'm curious if there's any others. But no, I think from my perspective, this is the kind of the some of the key trends and some, at least the things that that keep me passionate about this.
Mike: No, that's great. Go ahead mike. I [00:42:00] was just gonna say if you're a data fabric fan We want to hear about it in the comments. Let's
Chris Detzel: In the comments we'll post on linkedin and you can comment and tell us what you think so Thank you, for coming to another data hurdles. Please rate and review us.
I'm chris detzel and
Mike: I'm, michael burke. Thanks for tuning in. Thanks william. All right. Thank you guys
​
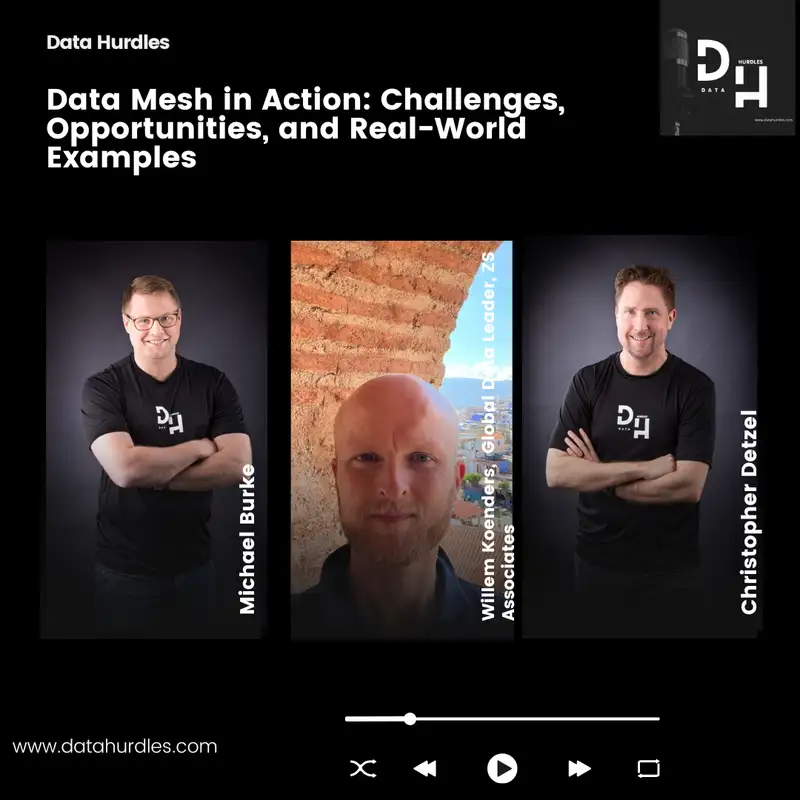