Data Quality: The Key to Effective Business Decisions
Download MP3Data quality is a critical aspect of any business, as it ensures that the information used to make decisions is accurate, reliable, and relevant. In a recent podcast, Chris Detzel and Michael Burke discussed the importance of data quality and best practices for managing it effectively.
Defining Data Quality
According to Michael Burke, data quality is about ensuring that the data used in a business is accurate, reliable, and fits its intended use case. In industries such as pharmaceuticals, data quality plays a critical role in patient outcomes. Poor data quality can lead to wrong prescriptions, supply chain issues, and customer dissatisfaction.
The Importance of Governance and Ownership
Chris and Michael use the analogy of a water works system to explain the importance of governance and ownership in data management. Every point in the system needs to have governance and ownership to ensure that everything works smoothly. This means that each team and individual responsible for managing data must have clear roles and responsibilities.
Improving Data Quality
Chris and Michael discuss how data quality can be improved through cleansing, standardization, and enrichment. Enrichment involves collecting third-party data that can provide more context about customers and businesses, allowing companies to make better-informed decisions on how to market and communicate with them.
Best Practices for Managing Data Quality
Michael emphasizes the importance of identifying data quality metrics and ensuring that they are monitoring what is actually important to the organization. He suggests starting with understanding what the data is being used for and the cost of bad data, then designing controls and monitoring strategies accordingly. Chris adds that it is also important to build metrics that stakeholders understand and that are relevant to their needs.
Emerging Technologies and Data Quality
The conversation also touches on the role of emerging technologies in improving data quality. Michael notes that while machine learning can aid in data quality control, it is important to remember that it is just a tool trained with math. However, there are some use cases where machine learning can identify patterns and trends that would be difficult for humans to do on their own. Michael gives the example of using machine learning to monitor data entry at a large organization to identify anomalies.
Ethical Considerations
The speakers also discuss ethical considerations around data quality and how organizations can ensure they are using data in a responsible and ethical way. This includes ensuring that data is collected and used in compliance with relevant laws and regulations, as well as taking steps to protect the privacy and security of sensitive data.
Data quality is a critical aspect of any business, and managing it effectively requires clear roles and responsibilities, appropriate metrics and monitoring strategies, and a willingness to embrace emerging technologies. By focusing on data accuracy, consistency, completeness, and integrity, businesses can ensure that they are making informed decisions that drive success and growth.
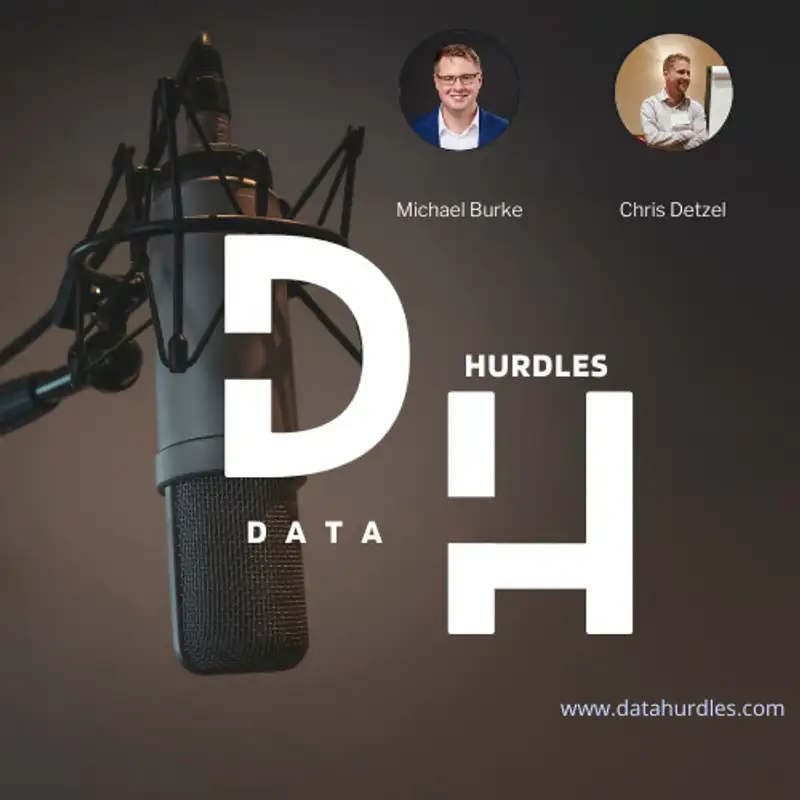