DeepSeek's Cost-Efficient Model Training ($5M vs hundreds of millions for competitors)
Download MP3The episode features hosts Chris Detzel and Michael Burke discussing DeepSeek, a Chinese AI company making waves in the large language model (LLM) space. Here are the key discussion points:
Major Breakthrough in Cost Efficiency:
- DeepSeek claimed they trained their latest model for only $5 million, compared to hundreds of millions or billions spent by competitors like OpenAI
- This cost efficiency created market disruption, particularly affecting NVIDIA's stock as it challenged assumptions about necessary GPU resources
Mixture of Experts (MoE) Innovation:
- Instead of using one large model, DeepSeek uses multiple specialized "expert" models
- Each expert model focuses on specific areas/topics
- Uses reinforcement learning to route queries to the appropriate expert model
- This approach reduces both training and inference costs
- DeepSeek notably open-sourced their MoE architecture, unlike other major companies
Technical Infrastructure:
- Discussion of how DeepSeek achieved results without access to NVIDIA's latest GPUs
- Highlighted the dramatic price increase in NVIDIA GPUs (from $3,000 to $30,000-$50,000) due to AI demand
- Explained how inference costs (serving the model) often exceed training costs
Chain of Thought Reasoning:
- DeepSeek open-sourced their chain of thought reasoning system
- This allows models to break down complex questions into steps before answering
- Improves accuracy on complicated queries, especially math problems
- Comparable to Meta's LLAMA in terms of open-source contributions to the field
Broader Industry Impact:
- Discussion of how businesses are integrating AI into their products
- Example of ZoomInfo using AI to aggregate business intelligence and automate sales communications
- Noted how technical barriers to AI implementation are lowering through platforms like Databricks
The hosts also touched on data privacy concerns regarding Chinese tech companies entering the US market, drawing parallels to TikTok discussions. They concluded by discussing how AI tools are making technical development more accessible to non-experts and mentioned the importance of being aware of how much personal information these models collect about users.
Major Breakthrough in Cost Efficiency:
- DeepSeek claimed they trained their latest model for only $5 million, compared to hundreds of millions or billions spent by competitors like OpenAI
- This cost efficiency created market disruption, particularly affecting NVIDIA's stock as it challenged assumptions about necessary GPU resources
Mixture of Experts (MoE) Innovation:
- Instead of using one large model, DeepSeek uses multiple specialized "expert" models
- Each expert model focuses on specific areas/topics
- Uses reinforcement learning to route queries to the appropriate expert model
- This approach reduces both training and inference costs
- DeepSeek notably open-sourced their MoE architecture, unlike other major companies
Technical Infrastructure:
- Discussion of how DeepSeek achieved results without access to NVIDIA's latest GPUs
- Highlighted the dramatic price increase in NVIDIA GPUs (from $3,000 to $30,000-$50,000) due to AI demand
- Explained how inference costs (serving the model) often exceed training costs
Chain of Thought Reasoning:
- DeepSeek open-sourced their chain of thought reasoning system
- This allows models to break down complex questions into steps before answering
- Improves accuracy on complicated queries, especially math problems
- Comparable to Meta's LLAMA in terms of open-source contributions to the field
Broader Industry Impact:
- Discussion of how businesses are integrating AI into their products
- Example of ZoomInfo using AI to aggregate business intelligence and automate sales communications
- Noted how technical barriers to AI implementation are lowering through platforms like Databricks
The hosts also touched on data privacy concerns regarding Chinese tech companies entering the US market, drawing parallels to TikTok discussions. They concluded by discussing how AI tools are making technical development more accessible to non-experts and mentioned the importance of being aware of how much personal information these models collect about users.
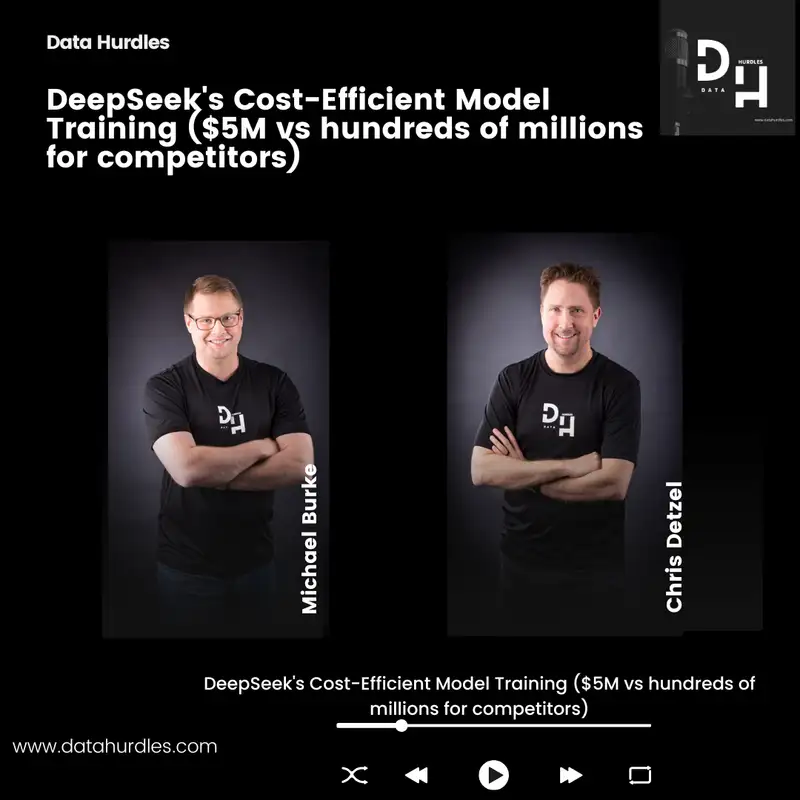