Enterprise Data Observability and the Future of Agentic AI with Ramon Chen, Chief Product Officer at Acceldata
Download MP3Data Hurdles: Ramon Chen, CPO, Acceldata
===
Chris Detzel:
Welcome to another Data Hurdles. I'm Chris Detzel. And I'm
Michael Burke: Michael Burke. How you doing,
Chris Detzel: Chris? Pretty good, man. I think we'll hit 90 degrees today, so it'll be nice and warm here in Dallas. So we have a special guest today. He's been on the show before, Ramon Chen. He's the Chief Product Officer, ex Acceldata
ramon, how
Ramon Chen: are you? I'm great. So thanks Mike and Chris for having me on again. Welcome back. We're excited to have you. Yeah, it's been a while and lots have happens in this fast moving world of ai, Agentic AI, AI and so forth, so I'm delighted to chat about that today.
Chris Detzel: Yeah, and we're excited to. That's amazing.
Yeah, and we're excited to, to have you and then to talk about that. But before we get going let's do a quick introduction. Ramon, tell us who you are again, and what's been going on at Excel Data and in your world today.
Ramon Chen: Yeah, so as you mentioned, I'm the Chief Products Officer at [00:01:00] Acceldata
we have an enterprise data observability platform. The data observability is a category that, when I spoke to you about a year and a bit ago. Was starting to get a lot of play, but it's full bone tidal wave now in terms of people's interest and understanding and how it fits in the context of data quality, data governance, and so forth.
So I'm excited to explain that to you. And Chris, as my career history involves a company that you and I both worked at Reltio. I was the chief product officer there for many years, and I've been in the data and analytics space for about 30 years now. Got the gray hairs to prove it. So deci excited to talk to you today about what's happening in the world of data observability, but also this notion of agent ai.
Chris Detzel: You're building a whole conference around that view, that's interesting.
Ramon Chen: Yeah. Thanks. Thanks for prompting. I'll do a lot of advertorial real quick here at the beginning. So autonomous 25 May 20th. In San Francisco, we are hosting industry leaders to talk around ag agentic ai [00:02:00] and the impact of ag agentic data management.
It's gonna be a fascinating topic. Fortune 500 companies are coming to present and speak industry leading vendors, so it's gonna be a very good time. Go to the Excel data website, excel data dot and you can register. And right now, for a limited time, it's completely free.
Chris Detzel: Wow.
Michael Burke: But nothing better than a free conference.
I feel like conferences have gotten too expensive across the board. Anyway, so tell us a little bit more about Agentic AI ai. I'd love to hear what does it mean to excel data and where are things going within the ecosystem of observability?
Ramon Chen: Yeah, thanks Mike. And so let's talk about data observability first, if I would.
You wouldn't mind, so data observability, I was just at Gartner, Orlando. A couple of weeks ago, and the notion and the understanding of data observability has just exponentially exploded. Instead of asking observability, I thought that's what Splunk or Datadog did. People know now who the players are in data observability and enterprise data observability, but [00:03:00] more importantly, they know how it fits right in the data management ecosystem.
Very briefly, just for the listeners who don't know, data observability is. In a word, proactive data quality as one use case, right? Being able to ensure that the data is fit for purpose. Gartner calls it a data readiness, right? Whether that's for a, and then the data to be fully trusted. Now, the word trust is used a lot from.
Anything from MDM to people who manipulate and deliver data through their silo in context. But this is really about making sure that the data from the very inception, from when it enters the data supply chain, has been vetted, not just for the quality of the data, but for the accuracy and the frequency in which it's being delivered by third party data suppliers.
Whether the schema has changed or not, right? And data observability are policies and rules that are enforced throughout these steps in the data supply chain so that people can have peace of mind so that data engineers, when they are [00:04:00] alerted of issues that could have occurred, they can resolve them quickly before their business users have problems with bad data in their business reports or in their AI downstream.
So that's data observability in a nutshell.
Michael Burke: And so with data observability, and I feel like across the board we're hearing that. The traditional way of doing observability with a Splunk or kind of this log monitoring process and writing manual rules is phasing out. At least it's beginning to phase out.
What is your perspective on where things are headed? How are we going to do this differently? I. Data is complex. The meaning of things like quality and accuracy can vary completely from one company to another. Exactly. How does Agentic AI AI help with that?
Ramon Chen: Yeah, so Agentic AI AI is applied to data observability.
So we just announced three weeks ago Agentic AI data management. So Agentic AI ai, I'm sure your viewers, you can't go anywhere without hearing about agents and agent ai this and AI that. But it's been mostly applied in the [00:05:00] context of customer support, sales and marketing, assisting using natural language and responding with a corpus of data as of a human being would to autonomously assist, right?
And help improve functions in those areas. And the productivity gains are quite well proven. The context of Egen AI in data management is very similar. So imagine if you will, a chat GBT or perplexity like. Interface where instead of you navigating a tool and looking at dashboards and trying to uncover whether issues or problems, the questions you ask will dictate the answers you are given back.
Not just the question, but who you are and why are you asking? So the system would be aware and would be intent based. They would know who you are, whether you're a business analyst or whether you are a. Data engineer and they would infer the reason of your question and give you the data in the more mode and form, [00:06:00] whether that be a chart or a graph or a table in the function and workflow that you would expect.
So as an example, if I'm a business analyst. I asked the question, what reports have poor data quality and should I trust them? It should get that list of reports, right? But then it would prompt you with, okay, what do you wanna do next? Do you wanna look at a specific report and find out why it has issues?
And here's the link to drill down. Further, and then you can ask further and further questions. If I was then a data engineer and I was asking this context, it would ask the reports, but it would immediately know as a data engineer, I'm here to solve the problem, right? I'm here to fix it or prevent the problem.
So it would immediately say, Hey, do you want to add some rules and policies that could really beef up the quality of these reports, add more checks if you will, checks and balances. So that's when the. Same sort of ideas approach from business side and technical side can converge on the same corpus of data that data observability pull together.
Michael Burke: And so [00:07:00] just so the audience gets this straight and I get this straight too when we talk about interacting with an LLM, like that is the assumption that we've got our data already indexed. And the LLM has an understanding this agent or agent AI has this understanding of all the data. All of my systems, and it also has an understanding about me.
So when I talk about quality and trust, is it making that decision based off of my definitions of quality and trust? Absolutely. 'cause my definitions and like an engineer's definitions might be completely different.
Ramon Chen: Exactly. Correct. So the first step is what data observability foundationally does, right?
Together with all the other tools, right? If you've ever heard or seen Mark Byer talk at Gartner, talk about active metadata, right? As the lifeblood, right? And in fact, Gartner says that we're in the active metadata era, right? This is now the evolution of data warehouses. Now, active metadata is the lifeblood of what's feeding ai.
AI data. Observability goes around collecting. Active [00:08:00] metadata and making it active across databases, across tools and then and catalogs and governance tools, and assembling it all together so that you can see pipelines, you can see data flows, and then using this active metadata derive rules and policies that you can then.
Put into context, but that active metadata, right? Much a thermometer tells the temperature, but a thermostat adjusts according to your desires and wants, right? Active metadata is that information that can be put into action. So this active metadata is the fuel that. The Ag agentic AI and AG agentic data management agents need as foundational, right?
And that comes from both structured and unstructured data, right? Harnessing and then extracting the metadata from that, and then the use of chunking and vector and. Databases and so forth deals with unstructured, whereas the traditional metadata is stored in system catalogs and as you well know, unity catalog Mike, right?
That has all of that great [00:09:00] information and using things like Iceberg and so forth. So once the LLM has all of this, the next step is then for it to get some metadata about the individual users that are asking the questions, right? It also needs to be able to be smart enough to say, wait, maybe I can't answer this question.
Maybe the corpus of information is in another LLM. Because it might be industry focused. It might be domain focused, right? Because you are using RAG and stuff and having smaller models rather than a monolith model will reduce hallucination and all sorts of stuff, as you well know. But armed with those two pieces of information, the system is continuously learning, right?
So taking the information, refining the answers. So there's definitely a level of training and active learning that needs to be done to start to make it. Fully customizable for you, just like you use chat GPT.
Michael Burke: So interesting. And the question that I keep running around with, and that's all I know you've asked this one before, is if we have LLMs that now understand our data and understand us, what is really the gap [00:10:00] between some of these roles going away?
And these agents really just starting to do these jobs. If they have that, a real understanding of us and our needs and what is quality, can they start to take action? Can they be more proactive? Is that something that you're starting to see emerge in Excel data or is that kind of like futuristic stuff at this point?
Ramon Chen: No, I think, all of the major leaders at Amazon and Salesforce have all talked about, this notion of. Retooling and re-skilling people, because some of the rote tasks are going away, interestingly enough. And I think this is still valid. A few a few months ago there was a statement made by the CEO of AWS that said that there'll be no coders anymore.
'cause code is being written by these sort of Agentic AI generative AI functions. So that's a scary thing because coders were always the safest in terms of jobs. Throughout my career at least. But it's really. And then, there's this whole thing where, oh, they're all gonna have to become prompt engineers and all of this stuff.
I think it's a little bit over, could be
Chris Detzel: engineer.
Ramon Chen: What's that?
Chris Detzel: [00:11:00] I said I could be a prompt engineer, 'cause I could Yeah. Even
Ramon Chen: you can be a prompt engineer, Chris. That's what we're saying. That's how easy it is. No, it's
Chris Detzel: not really easy. But
Ramon Chen: yeah.
Chris Detzel: The point is, and I've talked to Mike and other people about this as.
I can now, at the very least, without, I'm what you call a Vibe coder. Yeah. Vibe Coder Mike? Is that the, what they call it? And so I can build an entire website and just clean, crisp, I can even build a generator of, running kinda plans and everything else that tells you Monday through Sunday what you should run, what shouldn't run, and people just put in their information that they wanna run a three 20 marathon and then gives you a plan.
Boom, right there. I've already done it. And I'm like that, I know that's simple stuff, but you know what, it's, I know it can go deeper than that. Quad three seven is phenomenal, so I don't mean to off, but the point is that it will change coding forever. If it hasn't now, it will,
Ramon Chen: yeah, for sure. I think though, that there's still, we're in this sort of, I don't wanna call it Goldilocks period, but it but there [00:12:00] is a period right now where it's not fully autonomous, so our conferences call autonomous 25. And that's a little aspirational, right? I think that human in the loop is still very important, right?
Yes. We're not at the Terminator stage where we're all gonna get eliminated. Exactly. That, that's and then you guys do, you guys know p Doom. The quotient P Doom, right? So if you look it up on Wikipedia, p Doom is what are the odds? Everybody has their p doom number, right?
What are the odds of a agentic or a GI taking place and, destroying the human population? Terminator style. So everybody's running around. I wrote a blog post about p Doom about a year ago. George Matthew and Slack Partners had a panel. Scale up ai, which talked about it and super interesting.
Anyway, that's the doom and gloom stuff. But the reality is humans are still required, right? There's a lot of hallucination going on, but you don't want some agent AI system to make a decision without somebody first checking on it, right? But humans can make mistakes as well. So we're in this process where people won't be completely and entirely replaced, but they will [00:13:00] have to be better at different things.
The stuff that is rote tasks that involve sort of desk checking things that can be automated. Will be automated. And things that previously required two hours to pull together a presentation will take 15 minutes. So I see it as a hyper. It already does. Efficiency does exactly.
It really does, the hyper efficiency acceleration. And apply to data management. That is huge because there's so much siloed data as you well know, Chris, right? MDM deals with the siloed data that is the actual customer data and the product data, but there's siloed metadata that's not being put to good use for data management and not being used to be understanding the needs and wants, and simplifying and democratizing access to critical information about the quality of data and the reliability and the trust of data.
And so I think that's gonna change. I think, Mike, your point about, is are people gonna, yeah. Certain roles and responsibilities will be redirected [00:14:00] to other tasks. Yeah.
Michael Burke: Yeah. And it's it, the thing that's just wild to me is if I even told you two years ago, somebody who's in the. iSpace oh, LLMs are gonna be, able to label their own data and like some of these new things.
We just released this thing called tau test time. Adaptive optimizations. Yeah. Really? I saw your post about
Ramon Chen: that. That was really
Michael Burke: good. Yeah. And they're it's all using similar strategies with the same models, but the way that we're applying them to build on top of each other is transforming it into something new.
And I think that for those of you who know about reinforcement learning and that's learning like a baby learns how to walk is the easiest example to describe if a model is armed with these capabilities and they're armed with the right inputs to ingest new data. The opportunity is unlimited as to what it could do and how it could use its own context to make decisions.
And I think that's really where some of these agentic AI models we're gonna see just grow and skyrocket is because they're gonna start to learn about you based off of the feedback that you provided. [00:15:00] They're gonna start to learn about you also based off of the thousand attributes that they have on you on where you work and what role you're in and your experience level and.
Maybe every other search you've done on your intranet and on your internal portals. And that information is gonna give it the context to take all of the relevant information it has across the internet or its domain of knowledge and provide back really relevant data. Almost something that is a psychic in some ways that can understand who you are and what you're really looking for, versus just the words that you're typing into the search bar, right?
Or the way that you're communicating with it. And we're already starting to see this, right? We're already starting to see. Desal and I were talking about this a couple weeks ago, is that like my version of an LLM that I use regularly on a day-to-day basis communicates differently with me than his does with him.
And when you think about the context of a internal system like data observability, there's even more context. There's context that wasn't available online that now is just available to the business. And imagine how you can accelerate these systems [00:16:00] internally. Yeah. By using something like that.
Chris Detzel: Ramon, quickly, out of curiosity, do you guys.
Build your own LLMs or do you use like a, Claude or ChatGPT or whatever and build it from there?
Ramon Chen: Yeah so we use a whole host, obviously Gemini, and we use open ai. Our goal is to be agnostic, but we will start with a foundational LLM because that's a great question, Chris because we at Excel data, our clientele, ours, global 2000, fortune 500, right?
We only work with the very largest companies in the world because. They have the most challenging data problems, a variety and data environments and scale. And that's where we think, our differentiation stands. There are a lot of other products out there that do data observability that are very departmental scale, single snowflake instance.
And that's not where we offer are huge value. And when you're dealing with such squirrely situations at enterprise scale, the security checks that we have to go through to support. [00:17:00] Banks and telcos and life sciences companies is extreme. Everybody is scared and have their AI radar up around compliance and responsible use of ai.
So inevitably LLMs that will be used will be the choice of the customer, right? They'll have their favorite LLM, they'll want it to be within their vir VPC. So that's what we've architected for and that's what we're planning for.
Chris Detzel: It's interesting because I see this as the new cloud type stuff.
You have three different clouds, but you can go to all three or yeah. A company like yours or RLT or whatever. Can do all three or, and so it's you're gonna have to be experts. Your company has to be experts on each LLM. Is it Gemini? Is it open ai? Is it Claude, is it, whatever else is out there?
It's so interesting. It's just the things that you guys have to go through to, to teach, whether it's engineers and things like that. You gotta be specialized in all of that. I think,
Ramon Chen: yeah. That's the job, right? As a platform, that's what you sign up [00:18:00] for, right? And Mike knows full well, like Databricks is a fantastic platform.
You have to be all things to everybody. It's expected, right? Yeah, I, I have no fear on that. My engineering team doesn't. And the company, and then road, our CEO, if you want to be the platform for the enterprise, for data observability, you've gotta support all modes, all clouds on-prem. Hybrid, 400 petabytes, one terabyte.
It doesn't matter. Scale up, scale down, multi, multi-tenants environment, so on and so forth. So it all comes with the territory. We wouldn't have it any other way.
Michael Burke: Yeah. And the truth is Detzel, like these large enterprises, they have so many engineers that somewhere in there is an expert that if we don't build it or Acceldata doesn't build it, they're gonna build it.
And so you are competing sure against your other competitors, but you're also really competing about the with the engineers that can build some of this stuff and making sure that you are helping guide them on a way that's gonna reduce that total cost of ownership. That's just, and I've been through this, I've [00:19:00] built my own applications when there were third party tools out there.
Yep. Because they weren't moving fast enough. And it's just like that's, and then you get to this operational overhead that's not scalable. I think that's where all these applications in this space really play and provide value is. There's so much to be done, especially on the governance and management side, where there's just a thousand things that you didn't think about when you started that project that these commercial projects have already covered.
Ramon Chen: Yeah. The history. Mike if you're as old as I am you'll have seen this story before because. Doesn't matter whether it's gen AI or whether it was four GL back in the day. There's acceleration in productivity on building applications, managing data every single step of the way. There's something better and it never fails that, internal, it will say, I.
Why do I need to buy an MDM solution? I can build my own. What do I need? A data observability solution? Why do I build my own? It's not building the first situation. That's hard. You can get that up and running, but then you've gotta maintain it. Then you've gotta, if that person leaves the company, then you've gotta hand over and what the heck did this person do?
And who's [00:20:00] supporting it now? And so on and so forth. So as with anything, build versus buy the, and we'll see the same mistake, just like the stock market will crash and it'll come back up again. And people have bad memories. Same thing with build and buy. People will be like, yeah, I'm gonna build all of this using gen AI because I can just do it myself.
And GitHub and co-pilot will just code it all for me. And then. The Uhoh moment will come. Like, why do we do that again? Now I have this legacy thing that nobody wants to maintain. It's not my core competency, it's not my business. And some companies will do it themselves and then they'll go through the cycle and then they'll come back and they'll go we'll have to replace what we have.
Legacy doesn't matter if it's Gen, ai, doesn't matter if it was. OGL. Doesn't matter if it was built in NoSQL or Hadoop. Same thing's gonna happen.
Michael Burke: Ramon, where do you think. The future is headed. I know this is a hard question. We ask it to everybody these days because I think we're all floundering a bit in catching up with the day after day innovations of large language models.
But where do you think we're gonna be in six months?
Ramon Chen: I'll be back on this show and we'll be talking about something completely different. [00:21:00] That's the, that's my prediction, but, in six months. That, that is also an eternity, quite frankly, at the pace of things are moving at Right? Every single day.
You can pull up LinkedIn and there's gonna be a new announcement, and it's not one announcement. It's like later in the afternoon, somebody else is gonna make an announcement because somebody's gonna feel like X, y, Z made an announcement. When we made it the ent data management announcement just before Gartner.
I was chuckling to myself with my CMO because all of a sudden everybody had one and they were announcing it that week, and you could see the test releases that were pulled together in haste, right? Because yeah. It's if you are, if you're not announcing something and you're not having that vision in that direction you're gonna be left behind.
So I think the pace is gonna continue to change The economics of this with deep seek and all of that, it's already turned itself on its ear. I think that. I can't predict, and I won't predict, but I feel the path that Excel data is on and other companies that are [00:22:00] adopting Agen ai is the way to go.
I. How that gets received. I think there will be a little bit of a slowdown. Not everybody is comfortable letting AI do the work, right? Some people will still say, too dangerous. Let's, stay with the traditional for now. Keep an eye on it, and then there'll be like the late adopters that are coming in.
But it's a fascinating time. I'm not really answering your question directly, but I think, death taxes and the rate of technology change, those are three certainties right in the world. And I'm here for it. I think it's super exciting. This is what. Is gonna keep your podcast going here.
There's always something good to talk about.
Michael Burke: And when you look back on, where we've come from in the next year do you see any major risks with large language models? Governance? We're talking mainly about observability. I know, but really around the governance of a lot of these technologies.
As they start to scale up and be more embedded and proactive, are there any risks with that or has awaited those to some degree? Yeah.
Ramon Chen: Yeah. We know that there are risks that not just, so there's two risks, right? The first risk is the [00:23:00] use of ai. In data management without human in the loop, fully automatizing and taking actions without somebody governing it, that's gonna be a risk.
And we encourage all companies who are adopting this not to just flip the switch and walk away and go, go do something else. So that's number one. The second one I would say is, how is. And is AI in the other areas being responsible? Are they hallucinating and taking data and delivering really bad outcomes?
And so that's where AI observability is coming into play, right? So now I. You've gotta observe the data to make sure it's trusted to feed these large language models. But then once the models start to do stuff, you've gotta keep an eye on them so that they don't misbehave and basically hallucinate and do different things.
So the natural extension of data observability is AI observability. I think the two are synergistic. I think, just as [00:24:00] Databricks is the data and AI conference data and AI observability. Is the same one in continuum, in terms of an umbrella.
Michael Burke: Really interesting. Council, do you have any other questions to follow up with?
Yeah, go for it.
Chris Detzel: Just a quick thought in, in the snu. Relating to everything that we were talking about, but it's mostly around hallucinations. And one of the things that, I start thinking about is, these AI models are supposed to learn from other things. And we give it that. I think they give it, some flexibility to, to hallucinate, right?
And so as these things become smarter, what we think might be hallucinations might be actually what it learned and maybe things that we should take into account. Not saying right now, because it is. It's better actually than it has been over a year, but it still does hallucinate, but I think we really have to think about that.
Is it really hallucinating in the future or is it that we just didn't know because it's way more smarter than we are at some point. Yeah. It's an interesting thought. It was just a philosophical thought that I had about that, and it was like we wanted to. Yeah, [00:25:00]
Ramon Chen: for sure.
You must have been watching the Matrix movie or inception over the weekend, right? What's reality is the I that completely
Chris Detzel: changes this entire conversation, but. Sorry.
Ramon Chen: Yeah. It is what l lm say real. And you are just stuck in the past, right? Yeah, exactly. You just you, your, you have your way of thinking of things 'cause that's traditional and the LLM is coming with an alternate view and you deny or do not believe that's what it is.
There are certain things that are a little bit black and white in terms of, hey, this field is now, not now. There's some things that are just clear where, based on facts and data, it comes to a conclusion. Typically hallucinations that what, at least where I've seen it, is not, 'cause there's no ego in the LLM, right?
People always say MLMs are like humans. They don't want to not be able to give you an answer, right? So they're gonna spew out some garbage just because they're expected to give you an answer. And there's some truth to that, right? But you can tune it. You can say, look, if you're not sure, don't gimme an answer.
You can. Yeah. Or fact check it, right? That's why [00:26:00] all of these complexity, ChatGPT, they all have attribution now, right? And links, this is where I got this piece of information from. So you have attribution from source, and that I think is highly valuable because that engenders trust in the responses.
And the same thing will happen right in the Gentech data management. When somebody says. Which are the, and go by my original example, which are the reports that are the least data, poorest data quality. It'll spit out maybe a table of 10, and then it'll say, I figured this out through this. If you want to know, check my work.
Just like when you were in fifth grade or sixth grade math and you had to do an equation, you had to do a QED at the end to prove your theorem, so more and more that's what's going to happen. So hallucinations are essentially a combination of facts that were either misordered or incorrectly applied together.
But if you can see the origination of the sources and you can see how the conclusion was drawn, you can gradually train and correct that. And the arbiter of what is a [00:27:00] hallucination is, yeah, it's gonna be different for different people and different contexts. That's a great
Chris Detzel: answer, by the way, because that helps me a lot.
What I just learned too is once it's all, the thing that you're building, it's gonna save a ton of time. Just because it's gonna show you where I got the data. If I'm sitting there having to go find it myself and do all these, it's forever to do that. Now it's just boom.
Within like 20 seconds, it's there. Here's the link. Read it if you'd like, or just let it give you a summary. Anyways, go ahead, Mike. I
Michael Burke: was just gonna say though, to add to your point, to not, there are really interesting things that have happened in the past, like especially around neural networks and how information is communicated between one system and another.
So a really good example of this is Google Translate and Google Translate when you were translating between languages, and I forget the neural network's name that they used, but the neural machine translation. The layers of that translation between let's say Spanish and English actually be turned into its own language that [00:28:00] was more optimized Yep.
Than text, just to translate between two systems. Yeah. Yeah. And I think that as we start to really evolve in the large language model space. You'll still have your standard text outputs, but when you start communicating from model to model, there will be new systems that emerge that are gonna be much more optimized than just like a Latin character base.
Yeah. And you're gonna start to see that for sure. You were
Chris Detzel: talking about that like a year ago, Mike. Anyways, go ahead. When these models start talking to each other, have you seen
Ramon Chen: that? Have you seen that video that's going around YouTube and the internet where somebody is calling up to get travel agents advice and the model is talking to another model and they figure out that they're both models and that they go into jibber mode?
Have you seen that? Yeah. Yeah. That's pretty
Michael Burke: amazing.
Ramon Chen: Yeah. So then all of a sudden it's just, blah, blah, blah, communicating. So it's then they're not speaking anymore, but you can see the printed text and Yeah, for sure. It's a communication protocol like anything else, that's. Gonna occur, it's gonna happen.
These things are gonna evolve very quickly. I think that this is a [00:29:00] very fun time or if you're not happy or interested in this era, I seriously think you need to be examined because this is as much fun. It's even the road hallucination. This is as much fun as you can have without actually getting hallucination with LSD and smoking marijuana, quite frankly.
This is all technical fun and it's one big party.
Chris Detzel: I think it's amazing. I think. The time we're in is just unprecedented. It's nothing that I thought it could be, and I do so much more, with that technology than I could before and a lot quicker, yeah.
Even at work, Hey, you need this PowerPoint and you need some big long buzzwords about some things. Yeah. Boom. Okay. That sounds good. Yeah, mean just it's a pretty cool time and the things that it can do. So Ramon, this has been really great. Really appreciate you coming on. Oh,
Ramon Chen: thank you.
Thank you for having me on.
Chris Detzel: We'll definitely do it again. But Mike, did you have anything else before? I
Michael Burke: thank you Chris, and thank you Ramon. This is amazing. For our listeners on the call, don't forget to rate and review us. I'm Michael [00:30:00] Burke.
Ramon Chen: And I'm Chris Tetzel. And I'm Ramon Chen. Autonomous 25 May 20th.
Be there. There. It's gonna be. It's gonna be a rollicking. Good time.
Chris Detzel: You always put on a good event, Ramon, so
Ramon Chen: yeah. Thank you. Thank y'all. That was good. Thank you so much.
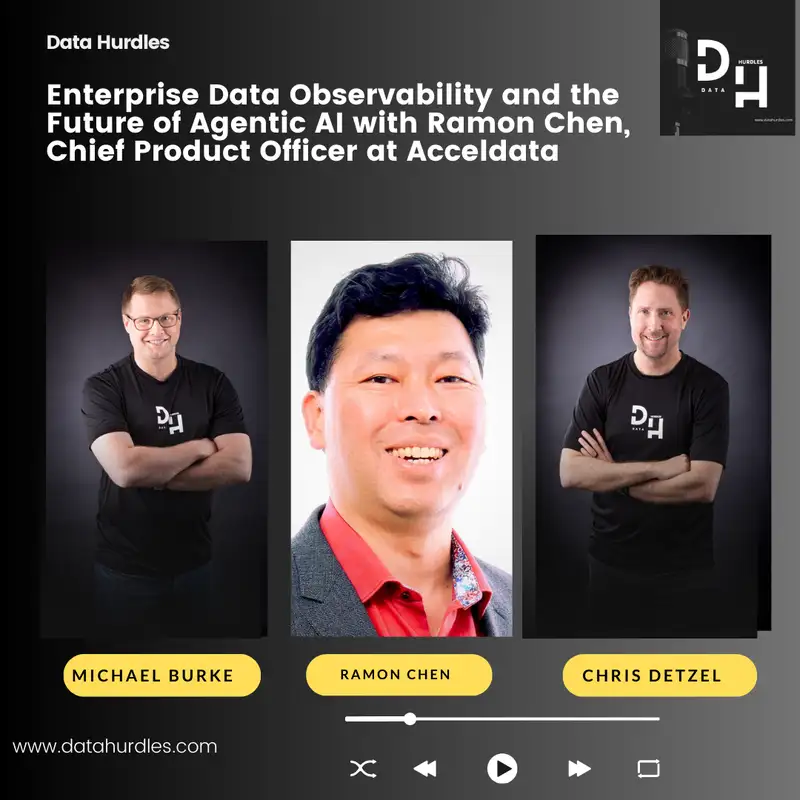