Data in Machine Learning and AI, Understanding the role, Hosted by Michael Burke and Chris Detzel
Download MP3In this episode, Michael Burke and Chris Detzel discuss the role of data in machine learning and AI. They define machine learning as the process of identifying patterns in data to create value and AI as a computer's ability to make decisions on its own. They also explain that data science is the larger sphere that encompasses both of these fields. They then go on to discuss the role of data in machine learning and how it helps organizations make better decisions. Finally, they use the analogy of driving a car to explain the importance of data in machine learning.
Michael Burke, a speaker, discusses how to evaluate the quality of a dataset and assess its outliers, as well as how to featureize the data in a way that makes the information fit the needs of what you're trying to solve. The example he uses is a dataset of passengers on the Titanic, in which the goal is to predict whether they will survive or not. The speaker talks about the importance of assessing the quality of the data and whether it is consistently and accurately logged for each individual passenger. The speaker then discusses featurization and outliers, and how to shape the data to create more meaningful predictions, such as classifying or sub-classifying groups of people. Finally, the speaker mentions that featureizing data can be an art and can involve working backward from the problem that you're trying to solve.
Michael Burke talks about how data science works, using the example of predicting house prices based on square footage. He explains that the data is plotted on an X-Y chart, and a linear line is adjusted to fit the points as closely as possible. The model then uses this line to predict the price of a house based on its square footage. When asked about best practices for data preparation, Michael emphasizes the importance of selecting the right data and collecting meaningful data that can help answer the question at hand.
The potential issues that could arise with machine learning models such as Chat Gpt. They talked about how human bias could be introduced in the training of these models, and how inaccurate or misleading data on the internet can negatively impact the results. They also mentioned the importance of vetting the information that goes into these models to prevent misuse, misappropriation, and misinformation. Furthermore, they talked about the integration of machine learning into big companies' existing data infrastructure and the challenges that come with it.
Some potential dangers of machine learning and AI technology. They talk about the need for caution in organizations that are implementing machine learning, particularly in highly regulated industries like banking and government. Burke highlights the importance of auditability and understanding how decisions are made by machine learning models. They discuss the risk of bias and exclusion in these models, especially in areas like healthcare and financial services, where the consequences of decisions can have serious impacts on people's lives. The conversation emphasizes the need for responsibility and ethics in the use of machine learning and AI technology.
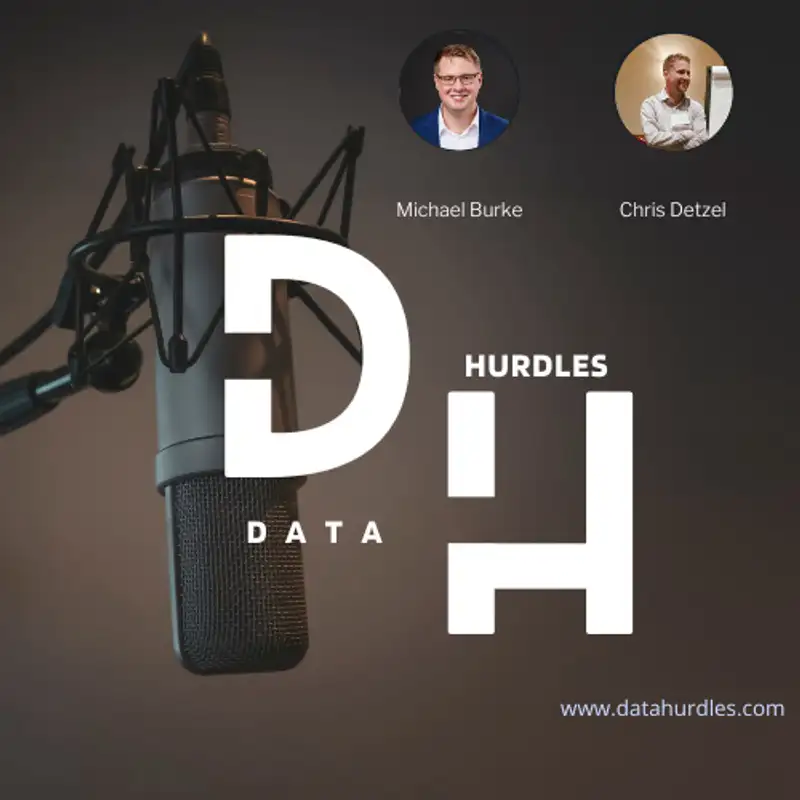