Revolutionizing Healthcare Data Sharing: Shubh Sinha, Integral's CEO, on Data Hurdles
Download MP3Revolutionizing Healthcare Data Sharing: Shubh Sinha, Integral's CEO, on Data Hurdles
===
[00:00:00]
Chris Detzel: All right. Welcome to another Data Hurdles. I'm Chris Detzel, and
Mike: I'm, michael burke. How you doing chris?
Chris Detzel: Good man here in dallas. It's hot as hell. So that's you know, typical I guess of this time as always As usual, we go out and my wife and I go out and are running Kind of things and it was tough this morning.
I got six miles in though So what have you been up to?
Mike: You know what? I have not hit the gym yet. It's on my list to do I'm gonna see if I manage to make it there today. On my goals, but yeah, doing really well. The weather here is beautiful in Massachusetts and loving the outdoors. We had a close scare.
We'll introduce Shub in a moment, but for you, for your background, I've been recently investing in chickens. This is like totally outside the data world. And we had a scare where a hawk came and we thought it took a few of our pets that was the big big scare yesterday.
Yeah, so we've been resetting from that
Shubh Sinha: Are we talking here
Mike: six six, yeah, nothing crazy so
Chris Detzel: not yet [00:01:00] until I have a little chicklings, you know All right. We have a special guest. Shubh Sinha ha is that right? Tell me I said it, right?
Shubh Sinha: Yeah
Chris Detzel: Got it. And he is the CEO and founder of Intragoal, and we would love to get to know a little bit more about you.
How about that?
Shubh Sinha: Yeah, let's do it. Let's do it.
Chris Detzel: I was gonna say,
Shubh Sinha: you let me know.
Chris Detzel: Yeah no, tell us a little bit about the company, your background, why you you know what made you decide to come up with the company and all that kind of stuff.
Shubh Sinha: For sure. For sure. Yeah. So I guess just a little bit about me.
I'm originally from Nashville, Tennessee, but I have a lot of family in Dallas and Austin. So Chris, I've been down your way many times and have gained appreciation for truly how large Texas is in many ways. But I most relevant to integral is the company I was at before this. So I spent a couple of years as a software engineer and then joined this company called live ramp, a public tech company based out of the bay.
And moved over to San [00:02:00] Francisco when I was working there and, LiveRAM's core tech, it the best way to describe it is that it solved data fragmentation where, if you're like a Kellogg cereal or like a CBG brand like that, oftentimes you have so much data stored in places that you don't own or have access to.
If you think about where you buy cereal, it's at Walmart, CVS and all these different stores. But all Kellogg really receives is sales figures and store numbers, and it's not much of a customer journey in that way. And so what LiveRamp did was Come in and partner with those retailers as well as normal data providers that exist in the network and pretty much created nodes to all of them and create a unified layer such that Kellogg could track a customer journey at a high level from point A to point Z, or, no serial to serial and, who's purchasing what and how and the idea there is to just get a greater depth of understanding for the consumer to find out, shouldn't there be more sugar free cereals? Or should you deploy inventory in this way? And so trying to get that granular understanding [00:03:00] of a consumer.
And then when I showed up in 2020, my mandate was to do that. But for. Regulate data, and especially in health care, as you might imagine, like a health care customer journey is even more fragmented than a regular customer journey where at least to get to a prescription. You go through a doctor, an insurance company, a pharmacy benefits manager, the pharmacy itself, and most health care journeys are not that straightforward.
Unfortunately. And so you have all these points in between yeah. And so anyway, I spent a lot of time building analytic solutions to be able to make sense of that customer journey for the Pfizer's and the modern as of the world. And for the farmers of the world, I realized very quickly that sensitive data or connecting sensitive data is very hard.
And so there should be a company to automate that. And make it easy. And so that's what integral is, but just at a high level kind of gives you a sense of the world from, yeah,
Mike: really interesting. So I've done some work with live ramp in the past as a data person. Obviously, they are one of the, big pillars in the kind of, I wouldn't call it [00:04:00] enrichment anymore, but that's what we used to call it, right?
What do you call it? Overarching journey, right? Of understanding a customer journey, right? The healthcare space is so interesting to me, what, When you were working in this space and digging into kind of health care data and the sensitivity, what were some of the big pain points that these organizations are having?
Is it sharing data? Is it encrypting data? Sharing it securely? Give us a little bit more detail to how does integral solve those pain points?
Shubh Sinha: Yeah, certainly. And if I had to sum it up, I would say most of the problems were around sharing data and productionizing it and in a rich state. And so if you think about any regular data set that is not bound to compliance, you can slice and dice, view it however you want, even share it with whoever you want, plug it into Tableau and you get all these like pretty nice features that help you understand what is in a data set.
And it really gets you a good depth of understanding. I think to translate that to the healthcare world and just more broadly to the regulated data world. Where you do have these sensitive data sets and combining certain variables can introduce a certain amount of [00:05:00] risk. That is a big no from the regulatory authorities.
I think it gets a lot more complicated. And so when it comes to data sharing what can you share such that 1 data is. Combined or it collides with any other type of data set. It's a compliant collision or a compliant aggregation. And then on the flip side, like, how can you make sure that you get a lot of analytic value out of a data set without getting too granular because I think it's like this constant balance where historically, I think the attitude was, oh, you got to be more conservative on the compliance side and that's going to destroy a lot of the analytic value.
But I think, especially at my time at LiveRamp, I realized that's not necessarily true. You can still maintain a state of richness and a state of high compliance and high privacy. And so I think that's what we set out to do. And I think it's primarily possible through technology, just given the speed at which we operate at.
But yeah, I think that a lot of what I saw was around the data sharing and the data utilization or the data productionization there.
Mike: Really interesting. And for our viewers that, don't necessarily work in the data space day to day. What is the [00:06:00] value that a commercial entity or a hospital would get from this data?
What is the pain points it's solving for them?
Shubh Sinha: Yeah, I think just very fundamentally, if you think about a human in their day to day activities, especially in the medical world, a lot of our medical and health care outcomes are dictated not only by our medical behaviors, but also our non medical behaviors.
So if you think like the average person, the amount of beers they drink, the city they live in, The fitness regimen they have, those are all technically non healthcare behaviors that all impact what prescriptions you have, what providers and ultimately, like what surgeries you might have, what complications from the medical side that might get introduced.
And the way I think about it for myself is I want my data to be safely utilized because if there's a lot of people like me, and maybe there's not a lot of people like me, it's I want that to be a. And I want a company to have that understanding so that I can get treatments catered to me at scale, or I can get, personalized messaging about what to do what not to do.
And that's what I think about is if you're a consumer, you ideally [00:07:00] want the world tailored to you, especially when it comes to the healthcare world. And so I think that the data availability is so important, but on the flip side, also, you want your data utilized really, Yeah, absolutely.
Really securely and really safely. And so it is like maintaining that. But yeah, for those who are not in the data world, I would say that for health care companies, especially, it's so important to have that combinatorial or that comprehensive understanding of a person just because they're all the factors really impact your health care outcomes.
Mike: That's super interesting. In. Why today? And I have had some experiences recently. I went in for a test. We have a patient portal in Massachusetts. The patient portal is fragmented. It only holds like certain records. Other records are on archaic systems. I ended up having to send a fax for a pre off on something.
It was just like a hot mess. Does this data? Is it mainly geared towards commercial entities or can this actually help hospitals and other larger organizations have a more unified view of a customer or a patient?
Shubh Sinha: Yeah, and definitely can relate with [00:08:00] you on that pain it's like you got to get to that first doctor's appointment 15 minutes early just to fill out all the Same information you've gotten and then obviously that's more of the innocent or benign.
Waste of time There's clearly more more important scenarios where time waste is just not possible, right? And so by the way
Chris Detzel: When you go to the doctor you have to go fill it out And when you go to the doctor again in two two months same doctor You got to fill out the same form and i'm like don't you already have this, oh, we're just updating i'm like This is exactly what I did last time. Anyways, keep going. Sorry.
Shubh Sinha: No, I was gonna say all of this stems from this super large problem in the regulated data and healthcare space where you have data fragmentation. And I think it's You know, a variety of reasons where some tech is up to date.
So some of the newest, I would just guess some of the newest provider offices probably have better tech than some of the ones that have been around for 30 or 40 years, which is contributory to the problem. The other part here is that there's a lot of data sharing that's recently started pick up because especially during COVID and now with a lot of the AI boom, you [00:09:00] have so much data being unlocked.
And so not only are people sharing more data and aggregate, but they're People's data is also being unlocked where you can take structured data or unstructured data and utilize it more effectively. And so for us, we seek to kind of power the entire exchange where at some point in the future, as a result of integrals infrastructure, that's buried deep in the in different companies.
There should be a world where you don't have to fill out doctor's forms every single time, or at the very least for some of the bigger hospital systems, there is an interoperability layer in the back that understands Shub at Hospital A in San Francisco versus Shub at Hospital B in New York is the same Shub going to these places.
I think the way to tackle that, however, is Call it top down in a way where we're working with the entities that have the most commercial incentives to be sharing this data and creating treatments and promoting messaging, but also in the data supply chain, so to speak, working top down, like we're starting with the data purchasers and eventually we'll work our way down to the data creators.
So the hospital system, [00:10:00] the care delivery areas, but definitely a lofty mission. So we're starting top down
Mike: and just so everyone else is aware, and these are leading questions a bit, but is this being, is this kind of lack of. Technology in the industry driven by regulation. Is it just driven by archaic infrastructure?
What's caused health care to just be so behind everyone else in the industry?
Shubh Sinha: Yeah. And I think with most health care explanations, it's definitely multi pronged. And in one way, there is this, Kind of overall risk that is presented with any kind of tech migration. Like you, you've seen in many other industries, right?
Where you migrate tech, and then you lose some data or whatnot or maybe the tech goes wrong and you have some critical systems go down. I think in healthcare, the critical systems are truly critical and that they deliver care and whatnot. And there is this better safe than innovative posture at times.
I think that being said, on the other side of the tech readiness, there's a lot of tech that's geared towards non legacy [00:11:00] institutions like health care, and I think only in the past 10, 15 years, have you seen at least from a BC and like tech perspective, have you seen healthcare unicorns and whatnot up here?
And in some ways the software wave or the technological wave and healthcare is younger than other industries. And so I think that's also contributory, but I would definitely say that now, especially with what we're seeing, there is a, where there used to be a desire. Now there is a necessity to keep up with the speed of the world because there's so much tech.
Encapsulating the world where health care is no longer immune. And you definitely still have areas that are a bit more on the better safe than sorry side of things. But I also think tech has become a lot more deliberate and methodical. And so there's more health care adoption happening because you see the equal care by which a company is engaging in with you and making sure your system doesn't go down.
Or if it does there's a quick backup in place because you can't have. Important machines go down or like important data infrastructure go down.
Mike: Yeah, absolutely. So these companies, you said you're starting [00:12:00] really at the top of the funnel, right? Mostly people that are willing to pay for information.
They're building these kind of unique 3 60 customer profiles right on their customers. How do they create value with that data? And, since it's a boston based company, I'll pick Moderna as an example, but we can obfuscate it to anything you want, right? A company that from what I know of them primarily is manufacturing vaccines, but I'm sure that they're doing tons of other research, right?
How would they use my customer 360 data to create more value?
Shubh Sinha: Yeah, and the most clear journey I saw over and over while I was at LiveRamp, but I think we intersect the most with at Integral is I would say targeted messaging or awareness of medications. And if you're Moderna and you have a vaccine out in the market, You want to get that vaccine to people in a way that's catered to them.
Not everybody has a CVS near them. Not everybody has a clinic near them. And so how do you simultaneously take into account me in New York where I pretty much have access to most clinics. And [00:13:00] then, back before I started LiveRamp I was living in a suburb of Tennessee where there just wasn't as much in terms of clinics and whatnot.
So it's like those two consumers have to be accommodated simultaneously. 'cause it's not it's like a Cheetos ad where you can ignore one. And so in that way, you have to understand both of those people at a certain 360 degree level that helps you inform decisions like ad deployment. So if you know that somebody has a particular an inclination to be on their on their cell phone, like you make sure to insert an apps, you make sure to have ads and certain apps that your audience is more likely to go to say, Hey, New vaccine available, go get in line or go register on.
Then, that's a very specific example, but it's this idea of how do you tailor the journey to individual consumers? You have to start with a 360 degree understanding of each of them at scale, and then you have to cohort them and then you have to understand what are the unique and scalable paths to spreading awareness or to letting them know that there is this new thing out and vaccine, especially when COVID was surging was so important for so many people, [00:14:00] how do you let the older population know simultaneously while letting the immunocompromised population know?
And it's all these like unique tailorings that you have to do at scale that are now possible because there's so much data to capture every part of a consumer's life.
Mike: That's amazing. That's amazing. I have so many thoughts about it, like competing in my head. I'm going to try not to get too far off on a tangent, as a company, right?
Unlike many other markets and the dirty secrets, if you don't work in the data spaces, we can target anything right at this point about you, who you are, what you do what you need. But with health care data, there are more restrictions, right? There's a lot less granularity.
Can you walk us through kind of some of the ins and outs of What is what? What can and can't you do with health care data? And also maybe just talk a little bit more about how that varies from state to state, right? We have all these regulations. C. P. A. GDPR. If you're working in Europe, how do those impact how you handle data?
Because for me, and even working with ramp [00:15:00] a few years ago, the complexities of that ecosystem just became overwhelming. And to navigate even the laws themselves are confusing as to what they mean and what you, how you can act by them.
Shubh Sinha: Yeah. And I think you're hitting on the key point, which is, there's a certain amount of reactive compliance.
So HIPAA has been around for 20 years or 15 years, however long it's been around. But it also got an update last year for tracking technologies, which weren't around 20 years ago. And so you have this, what I'll call like a reactive compliance solution. So You know, most companies know what it means to be HIPAA compliant with your data and what you can target on and what you cannot.
But then you have the state data privacy regulations that you just mentioned, where in the last 4 years alone, you have 23 states that have released their data privacy regs. And. I think what's likely going to happen is more of those come up, because I think we look more like a fragmented GDPR in the future than a one sweep all kind of policy, in which case federal typically stacks on state and then state [00:16:00] stacks on federal, depending on where you're sourcing your data and how you're using your data.
And if you think about just our previous example, like Moderna. They're not going to not use California data, just because CCPA has more restrictive covenants than HIPAA, and they're going to find the union in between HIPAA, or they're going to union HIPAA and CCPA and take into account both of them at the same time.
In which case, that becomes a little bit of a proactive stance, because. There is no like unique combination rule, but you have to take that into account for being compliant, which I think is important, not only because, you want to avoid fines as a company, but at the same time, customers want to build trust.
And the only reason they share data is because they know it's used somewhat safely. And so I think there's a real risk there to not doing that. And so to the point you were making about navigating all that, I think that is why we developed the software. We did where it's a pretty robust automation that powers data connectivity, but it's not that we went policy by policy and automate each of them.
It wasn't like, CCPA is Monday, Virginia is Tuesday. It was more like, we spent a [00:17:00] long time developing a product that could learn, interpret and apply regulation to find embedded risk relative to that data set. And as new policies come out to the point of being proactive. You're not going to be a multi billion dollar company and launch a data set and then just, say, Oh, there's no compliance around here.
You're going to be on the proactive side and say, I'm going to be compliant ahead of new laws, or I'm going to be compliant as new laws are being passed or as laws are already passed. And so that's where we help navigate the trenches a little bit there, as well as just also making sure to avoid the known complications.
Mike: Really interesting. Now, I know a lot of companies and I've got a few more big questions to come, but a lot of companies right in this space, they build out an ecosystem and then eventually the level two is some sort of cross connectivity. Or even data store or place where you can sell and commerce data in a way that's meaningful and safe and reliable are things like that in your foresight.
Are you looking towards the [00:18:00] ecosystem like going further upstream? Or where is where's integral heading in the next 3 to 5 years?
Shubh Sinha: Yeah, certainly. And I think for us, there is a network effects that appear. So what we notice is that if company A is an integral partner or customer, and they want to share data with company B, and they're not our partner and customer.
There's a, there's usually a setup integration process that still happens more quickly than if you were to use the incumbent solutions of consultants. And you can allow to share data. But if you have two integral companies sharing data with each other, because we have direct integrations, it happens relatively seamlessly.
Where there is that network effect of, okay, you're already on the network, you're already on the compliant rails, and don't think we have like a data store per se in our future there, but you can easily see how it acts as like a pseudo data store in a way. And so my goal is not to play matchmaker with data.
Typically, we come in when people already know what data sets they want, or we help them data set purchases that they want. And so usually there's an idea of here's what It's there, as opposed to them coming to integral and saying, give me what you got [00:19:00] thing. And so we're typically coming in when there's already an idea for exploring, and you either need a magnifying glass into the data set to find out is this worth buying or you need a magnifying glass to find the embedded risk and then have integral proposed solutions and execute those solutions and deliver the data in a timely manner and then maintain that real time regulated data delivery throughout quarters or months or days, just because that's how quickly certain data sets change.
Mike: Really interesting with all of this, right? In the last year, we've slapped on a I and large language models. And now it's like the rage. Everybody wants a large language. Are you guys working with large language models? If so, how does that change the landscape?
Shubh Sinha: Yeah, certainly. And from a customer perspective, we have a few use cases.
And I definitely noticed for us, it's the same implementation, just edited in a way that we're looking for. All right. Maybe different types of risk because you have to look for bias and data sets, and you have to look for certain things that a model can learn from.
Whereas, if you're just putting a data set in a data lake for it to be [00:20:00] analyzed, there's a model can hallucinate a query cannot for the most part. And so you have to worry. You have to worry about that. If you're the company. Ideally, if you use us, you don't worry about it. But if you are the company, and you're thinking about concerns and compliance risks for things to mitigate those are things that you have to think about.
And so for us. In the use cases, we definitely make sure to have maybe like a more comprehensive understanding of the data with regards to bias and whatnot, because you have certain executive orders that are coming out. And I think, regardless of whatever happens throughout the course of the year, I think user privacy is something that people are typically aligned on.
And so we see getting tighter and tighter, especially with how models are picking up in pace and innovation, which is a fantastic thing. But The model is also only as good as the data that goes within it. And so it's like this equal parts handshake. And then internally, yeah, we're definitely leveraging a lot of these innovations where, for us to be able to understand more greatly in our specifically in our R and D where, where can we improve?
How can we always make sure we're moving faster with regards to internal deployments and whatnot? Definitely. I think. [00:21:00] AI has catalyzed the work of what would be like an additional five or six people, but making it cost of 20 a month or whatever it is to do or, some of our home homegrown models that are on prem siloed off from the world.
Mike: It's so amazing. And we've talked to a number of data leaders over the past year on large language models and the effect on data quality. It's already hard enough if you've got a complex ecosystem inside to maintain and manage quality. How do you help ensure customers as they're integrating in all of these third party and nth party sources that consistency and quality is maintained?
Shubh Sinha: Yeah, and that's where our software, if I had to oversimplify it, it can interrogate a data set very quickly and very comprehensively. And in that world, say, you have a ton of 1st party data and 3rd party data. We'll start with interrogating each data set separately and even each column, each value in the data set separately.
Luckily, it's a computer doing all this. So it's relatively quick. But combinatorial [00:22:00] analysis of what does it look like to combine each column? And what is the risk profile there? So you have this almost like this independent and combinatorial analysis. And that is what showcases the risk in the entire data set and where the risk might be hiding in the corners that you have to analyze.
And then on our end, as we're coming up with problems and servicing them to the data buyers, because it's. They want to know where the compliance concerns are to get ahead of them. We're also generating compliant copies or compliant options such that they can have something off the shelf that's ready to go or they're able to customize it.
But, a big thing that we do in all of our implementations is we make sure to gather data preferences ahead of time because I think compliance and data utility have to be treated equally. And so what we typically do is that one of our off the shelf copies typically works out just because we have that preference ahead of time.
And then, we do our delivery and that happens on some sort of cadence. And so for us, it is really managing that process end to end and making sure things are weighted relatively equally from a data buyer, data purchaser perspective. [00:23:00]
Mike: Really interesting. And so Do you have your own, I would say templates or predefined schemas that you recommend people fit to so that those transactions are better?
Or do you do all of the connectivity behind the scenes?
Shubh Sinha: Yeah, it's a function of both where if a company wants just like the quickest solution possible we've done so many of these now we can say, Hey, if you want something that's cookie cutter, we can get that for you. But I would say 95 percent of our use cases fall under the custom umbrella, a lot of where.
Oftentimes, the incumbent solution is a consultant. And because it takes a lot longer, like 6 to 12 weeks compared to hours to a few days for us custom, you see a lot of custom requests. In which case, while we do offer predefined schemas, we find that the real part where integral shines is balancing customization and speed.
Mike: So looking ahead, we've talked a lot about today and thank you for getting in the weeds of me. This is where I shine. I love to learn about new technologies [00:24:00] and new companies, as do a lot of our listeners. Where do you see integral moving in the next three to five years? And how do you see the market landscape changing?
Do you think there'll be less regulation or more standards? Where are things headed?
Shubh Sinha: Yeah. Yeah. And I like to call this a founding vet in a way. So like, where do we see the company going in five to 10 years? Yeah. My feeling is that healthcare financial data, they led the charge where you see more and more data being regulated now.
And I think that's due to a couple of reasons where, you know, across the pond, you have GDPR. That's really showcasing to the world that user privacy is. A lot of people mixed opinions about it, but I think the emphasis is that user privacy is important, which is probably the thing that people align most on.
And I think that's shining a light to the rest of the world where, whether it's state data, privacy laws, whether it's the bills in the house that have to do with validating user privacy at a federal level. I think there's more regulation coming. And From that perspective, look, if all data is going to be regulated, I think Integral is relatively well positioned to create a [00:25:00] regulated first data stack in a way where if you are working with sensitive health care data and you're looking to productionize it as soon as possible, you can use us.
If you're looking to, work with consumer data and combine it with other types of data and making sure you're compliant in 15 different states, as well as with HIPAA, like that, I think Integral is just well suited for. And so where I see the world going is that more and more data is regulated.
However, I don't think that it's going to prohibit usage. It's just going to incentivize a higher posture, higher compliance posture, privacy posture to deal with that data, which I think is going to be in the betterment of consumers as well as companies, because I think they're going to use it to build trust.
And so that's how I think about the world, in which case, I might be biased, but I think we're well positioned for it.
Mike: No, absolutely. I couldn't agree more. I think that in addition to. regulation, you're going to see a lot more auditability, right? The need to be able to go back and say, how did you make this decision, right?
With your model, or, how did you market to these people with this cohort? How was it formed? That [00:26:00] explainability is just coming through in every asset of this kind of new modern data stack. Yeah, thank you so much. This is super helpful. Sorry, go ahead.
Shubh Sinha: Oh, no, I was just going to say, I think that if you think about, All data being regulated.
You almost have your compliance stack and your data stack merging. And there needs to be a link between those two. And I think integral is the ideal link.
Mike: That's excellent. Thank you so much for your time today. This was incredibly I would say educational as well as really tremendously helpful. So thank you so much for jumping on the show today.
We really appreciate you joining. Don't forget to rate and review us. I'm Michael Burke.
Chris Detzel: And I'm Chris Dutzel.
Mike: Thanks for tuning in.
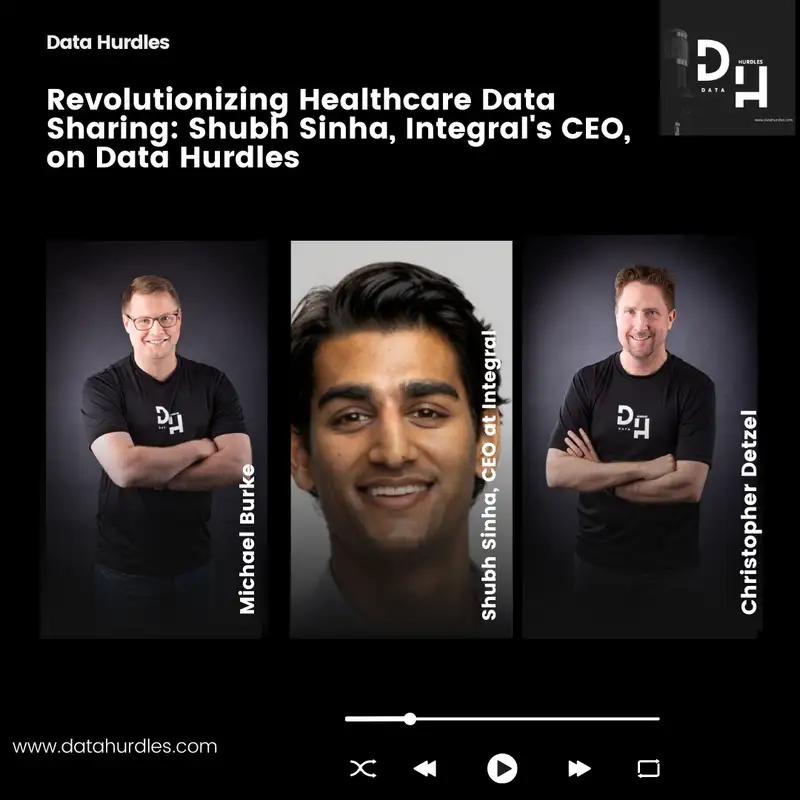