The Future of Data Teams in the AI Era: Insights from Alex Welch, dbt Labs' Head of Data and Analytics
Download MP3Data Hurdles with Alex Welch - Head of Data at dbt Labs
===
Chris Detzel: [00:00:00] Hello, data enthusiasts. This is Chris Detzel and I'm Michael Kirk. Welcome to Data Hurdles. We are your gateway into the intricate world of data or AI, machine learning, big data, and social justice intercept, expect thought provoking discussions, captivating stories, and insights from experts all across the industries, as we explore the unexpected ways data impacts our lives.
So get ready to be informed. Inspired and excited about the future of data. Let's conquer these data hurdles together. ~ ~ All right. Welcome to another data hurdles. I'm Chris Detzel and
Michael Burke: I'm Michael Burke. How you doing, Chris? Pretty
Chris Detzel: good, man. How about you?
Michael Burke: Really good. Since our last episode, which I think that we recorded two weeks ago, if I recall correctly, I had a baby girl. So we have a new member of our family. And I have my mind has been totally preoccupied since we last connected.
So
Chris Detzel: you haven't been thinking about data? [00:01:00]
Michael Burke: Not at all. Not at all. I've actually been thinking about, like, how to react to something where I get no data from it, right? So Well,
Chris Detzel: That's awesome, and congratulations. And before we forget, we have a special guest today, Alex Welch. Alex, how are you?
Alex Welch: Doing really well.
Thanks for inviting me. I'm here.
Chris Detzel: Yeah, you're from DBT Labs, and you are head of data analytics. Is that right?
Alex Welch: True. Yeah, I've been there for just under a year now. So start back December. Awesome.
Chris Detzel: Yeah, great. So Alex, let's just dive in. Tell us a little bit about yourself, how you get into the data space, what you do today, and all of those kinds of things.
And then we'll dive into our topic today.
Alex Welch: Yeah. So just a quick, I think I'll touch on really quickly, just what I've been doing the last decade. And then it is interesting. We're talking about how I actually got into it. But so for the past, yeah. Little over a decade, I've been working in the FinTech space particularly in companies that build platforms for derivative traders.[00:02:00]
And so I've been part of a couple of exits building, helping build up companies being sold never gone public, but through private acquisitions. And so I've gotten lots of. I've had lots of really interesting experiences, both like being ~ ~Create a data team within this already established org, create a data team with nothing, inherit data team in a larger org and kind of figure out the organizational structure and things like that.
And then when I, last year I did was Thinking, I wanted to buy something different and dbt lab came along and it's a completely different beast, it's enterprise SAS, not B2C, trading platforms. And so it was, it's been a incredibly exciting challenge and learning a lot.
And, but a lot of the same paradigms and concepts when you're thinking about data teams translates well and the relationships and things like that. Yeah, it's just into the new business model, learning all those new concepts and then applying what we can.
Chris Detzel: Yeah. That's great.
That's
Alex Welch: Michael.
Michael Burke: I was just going to say, I've heard so much about [00:03:00] dbt labs over the years. I was a, most of my career, I've been a big data bricks user, for the past and like the coupling for so long, they, these guys were so close to one another, I feel and that kind of collaboration and workflow Chris, go ahead.
I don't know. I didn't mean to cut you off there.
Chris Detzel: No, I'm glad you did. Because it's funny because when I told Michael that we're going to interview you and talk to you, he's what, dbt labs? I know.
Michael Burke: Yeah. Another cool company. I was like, you guys I've seen you guys for years. This is great. ~Yeah. Yeah.~
So can you tell us just break it down really quickly at dbt labs? What are you doing and how are you as the head of data kind of driving value within the ecosystem, which is now massive, right? They've grown, I don't know how many X over the past few years, but crazy.
Alex Welch: Yeah, I think it's really important to understand the stage we're in, right?
So you have like you're startup phase where you're doing product market fit and you're really trying to figure those types of things out. And at that point, a lot of the analytics that are really important are top level. It's okay, what are my revenue? Maybe some cohorting to really understand like nuances of your acquisition.
But [00:04:00] we have very solidly transitioned transitioned into this go to market phase where getting out there expanding pipeline, expanding presence is really important. And when you do that, the rest of the organization starts to scale as well. And and everything becomes much more nuanced too, right?
It's not just I want to see a cohort. It's I want to see. This group of population, how they interact with X feature. And what does that mean in terms of the retention if they do? Why? Yeah. So it is looking at the stage we're in and saying, Okay, how do we facilitate that?
How do we not become a bottleneck as a currently? We're a centralized data org. And as you if you don't be very thoughtful about that. You can very quickly become a bottleneck. You can be that, scapegoat where people are saying like, I'm not getting my data, I don't know what I'm doing. I have no visibility.
And so we're right now we're looking at, okay, like what is the next evolution of the actual composition of our data team? What does that look like in terms of how it works with different functions? So like, how are we going to work directly with product? How are we going to work with sales and operations and [00:05:00] marketing in very specific ways?
So that we can get those nuances, we can tie into the strategy and we can actually drive value there.
Michael Burke: I love that so much. In my, one of my earlier roles, I was working tightly with the marketing team as an AI team kind of data group. And one of the things that we were working on was like data as a product is a term that we use a lot now, but wasn't quite there, eight years ago or whatnot.
And actually being able to build products. Where, depending on who you are, maybe different things show up differently within the application, right? If you're a data stored versus data engineer, for example, you might have completely different needs on what you need to see in a menu or a drop down, right?
And like that prioritization of features and without confusing users between documentation and other things. But can you level up certain capabilities to make them more accessible and more relevant?
Alex Welch: Yeah, absolutely. That's very much in line. So Tristan, our CEO, released a white paper about the A.
D. L. C. The [00:06:00] analytics development life cycle. And I wrote a companion article for that. But, the core of that is, and I'm gonna tie it back to what you were talking about is this concept of personas and how you can, trade the hat. And move across personas as you need to. And we can talk more about that later.
Cause I think it fits in well. When we are developing, when you are thinking about the features, what you want to release, you want to build things in a way that allow people to change those hats, but doesn't create friction or, having them to completely change the frame of reference in their context.
So it feels natural, part of the ecosystem and part of their flow. And it really augments their flow instead of. Being a barrier, right?
Michael Burke: Yeah, totally. I always think back to Adobe Photoshop if you've used that tool ever and like their modes, right? You can select like the designer mode or the presenter mode or the curator mode.
If you're an illustrator, you need different things, right? And I think this is actually a really good segue into one of our first questions that I'd love to dive into, which is, AI has become such a household term over the last couple of years. Yeah. [00:07:00] AI driven changes in the data industry.
Really, are different from previous technological shifts. Yeah, and especially related to your work today How do you see ai driving new change within your ecosystem?
Alex Welch: Yeah, so I see Ai ~ ~Not just in my ecosystem, but like in the data industry in general two primary differences between past like shifts we've seen on this A.
I. One. And so the first is, previous shifts were primarily tool based, right? So moving from like mainframes to client servers and then eventually to cloud, right? And it really focused and changed on how we work. The other big difference is that the shifts We're largely deterministic, right?
And that's completely different than what AI is now. So like jumping back into the first one, we're talking about tool based paradigms that shift, right? AI has changed what we work on and what work is actually done. Not necessarily completely how we do it, right? Like it changes, like we prompt [00:08:00] engineer and we do those types of things.
But now AI is really representing like cognitive transformation, right? It requires a lot more judgment when you get outputs and like review and it started to blur those roles, the boundaries between roles. And I really think that in previous shifts, it was about amplifying human capacity and our skills.
And now it's really focused on augmenting our cognition, which is a huge shift between throwing a hammer up and down, and then understanding that this hammer is not great for putting something in a screw, right? Totally.
Michael Burke: I love that. I think that's such a good analogy too. The ecosystem of how we do things, it might change in the future, but certainly not now.
We still now have to think through process in a way that is, at least in my opinion, I'm sure there's people that disagree here. This is now a new territory that we're walking into, we still need to think through process when we're doing everything. And we still are. Experts in this space.
And we need to think of as additive, not a [00:09:00] replacement. And for most things that we're doing in the world, in my opinion, at least, right? Yeah. So with this kind of change, what do you consider to be the top three best practices for organizations looking to adopt AI tools in their data operations? There is a lot more coming, I think, with large language models.
And some of the things that we're seeing with multi model build outs and how those are evolving, I would say, like process of thought. How do you see that changing in dbt labs? ~ ~Data space. Really?
Alex Welch: Oh, so are you talking about just the impact they're gonna have or like the three best practices when you're like looking at the org and yeah,
Yeah, absolutely.
Yeah if you are actually seriously considering adopting AI, I think they're like. Three really core areas to focus on, right? You have your data foundation, which has to be solid, right? You need a data quality framework where you're going after all the usual suspects, right? Accuracy, completeness, consistency, et cetera, et cetera.
You need a, Yeah
Chris Detzel: [00:10:00] quick question on that because michael and I and even in our past experiences We talk a lot about data quality and how important that is and it's core it's been a 20 year long conversation that nobody really has Seemed to figure out, you know can you talk a little bit about that because I think that's just such a hard problem to solve You know, it
Alex Welch: really is and I you know I think there's never going to be a true solid answer.
I literally just said, you need a good data quality framework. And I think the key word there is a framework, right? Like you need to have something in there where, you have your automated validation rules and channels and you have some sort of error detection or anomaly detection going on.
Maybe you have some run books in place for when certain aspects of the pipeline fail. Things like that really help. And then. I think another area that people and teams. ~ ~Skip over in the early days is actually putting metrics around these things. So you can actually just track what it is, right?
If you're not tracking your data quality in terms of like your freshness metrics or, your [00:11:00] accuracy or your role volumes, basic things like that, if you're not looking at that and saying, okay, How am I responding to these? Is it something that continually happens and using that as a feedback loop?
Your data quality is going to suffer and it may not be a lot at first, but it's it's just like fact that it's accumulation of that and it will continue to get worse. And yeah, I think the key word there is just a framework that you can give to your engineers, give your teams where they go, okay, ~ ~Now, at least I know where I should be focusing my quality efforts and it it removes A certain level of ambiguity and the mental load that they have to take on to actually take action
Chris Detzel: I think that was great and I love The little bit more detail around the data quality the metrics and just what you should look at to at least You know the you know pushing out.
Hey, it's just a framework I think it's important because you know Just talking high level data quality that a lot of people do is let's dive deeper into that to make sure and understand what that really means. Thank you. That was good
Alex Welch: Yeah, and i'm a huge And you'll probably hear me use word framework tons of times over the course [00:12:00] of this I'm a huge of just okay, here's the guidelines, right?
And you've hired your individuals for their skill sets for their expertise, right? So here's the boundaries and go and do what you think is best, like how you execute and things like that. But at least you have that framework in place so that they can execute in it. Perfect. Thank you. Yeah, go ahead.
Michael Burke: I was just gonna add to it.
I feel like data quality is in so many ways like a misused term because it's almost like telling a runner they need to run faster, right? It's what are you? What is important? What do you need to optimize in your ecosystem to improve quality? Do you need if you're a runner? Do you need new shoes?
Do you need to change your biomechanics? There's 1000 things that you could think about. And the same thing with data quality is like I think when we say these words, we think about certain things like, Oh, the data needs to be accurate, or it needs to be cleaned or merged properly. But there's so much more on top of that with.
What are you steering towards right? What are you trying to get value? What is the value that you're running after right in your [00:13:00] ecosystem?
Alex Welch: Yeah, I have a couple thoughts on that one I think your running analogy is excellent there, right? Because everybody that goes out and runs is a little bit different, right?
You always hear these new training paradigms or Whatever it is, but a lot of that is framework put around okay, within the context of slow runs and mixing it with speed runs and running efficient metrics, right? All of that, you have your framework and you fit it in where you can excel in that.
And then the other thing I was going to say is, I think a common trap that data teams fall into is looking at their Entire stack and saying like I need accuracy across the board. I need 100 or 99 and in doing so you're treating every individual component of your ecosystem With the same level of criticality and scrutiny when it probably shouldn't be you probably have a bunch of data in there That's you know, not as business critical, right?
And if that goes down, maybe it can wait Two days or three days, but if you're like revenue [00:14:00] numbers or your real time to your Contact center goes down. You need to be able to execute and make sure that data is accurate So if I had to give like a big piece of advice, it's look at your ecosystem and say what are the critical elements?
Let's start there and get that accuracy in those quality metrics and then like triage down from there You're spending the time on the right things Especially when you're trying to implement something like an ai initiative
Chris Detzel: one You don't want to some of this programs or projects could seem like boiling the ocean.
Oh, yeah. So if you have all these data systems with all this data in there, maybe you start with one or two rather than, oh, everything needs to be done, within six months, right? You're just gonna. Go and piece it together. Because to me, it's sometimes you get in these programs like I want this and this and this and I can't do all of that.
Yeah, exactly. It's gonna take time. And like you said, what's the priority? What? What needs to be done first and then go from there? I love that.
Alex Welch: Yeah. Going back. I think that feeds well [00:15:00] because you have a data quality, but it also goes into like setting up a data governance framework. ~ ~I think that's really important for your org when you're getting ready for that.
And I think you need data owners who are accountable and they also have the ability to make decisions about the system. I think traditionally we've separated those two. Where you have analysts who are accountable for the data that's coming out of it, but then they aren't, they don't have the ability to actually go in and make decisions, changes about what is happening underneath the hood.
And I think that's really cool thing about dbt is we've started to bridge that and allow that to happen. But then you need other things like perhaps like a governance committee to help set policy and settle, discrepancies and alignment issues or and what I think is going to be particularly important are this concept of data stewards that live within different verticals.
And the reason I believe that are going to be really important is because there's a domain expertise. And as you give out fine, more finally tuned AI systems that are specific to marketing or sales or whatever it is, you need somebody who has that really [00:16:00] nuanced information and mindset to be able to go in and like help, help Guide that and roll that out in addition to your data and engineers and stuff like that.
Michael Burke: Totally. It's like I think as practitioners, we always, we want to centralize, right? We want to standardize. It's in our nature to want organization and structure. But at the same time, Experts are going to be collecting information and having insights that we will never have. And I think like a, such a good example of this was early in my career, I used to think that we should be able to do everything with data.
Like we should be able to make informed decisions on everything. And then I remember much later, like I was at a sales meeting with the sales guy who is 10 year, he's been in the industry 40 years. We're talking to this customer. And I walk away thinking, all right, this is closed. Like we know enough about this customer's insights.
Like we're going to get this. And he looks at me and goes, no, he's not. Did you hear his tone change at X, Y, and Z points? And I was like, no, I totally missed that. And it was like, that was the quality of data that somebody who's been in [00:17:00] the industry for 40 years is going to capture. ~ ~We'll never be able to centralize.
That's an expert operating in an independent silo that needs to happen that way. But like somehow I think in this ideal system, we are able to pull that kind of information back faster and more efficiently so that others can learn from it. But we're so far away still, I think with everything that we have in this ecosystem, there's still a lot more insights that like we.
We, I think we don't give ourselves credit to say that we have a lot more to learn, to be able to really make these informed decisions.
Alex Welch: Yeah. And I think the other component of that is, Oh, sorry. Go ahead. No. Go ahead. Oh yeah. No, I think the other component is your point like, yeah, we will never have that context of being able to read the tone and whatever, because we're not in the room, but nor should we, right?
That's not our core competency. That's why that individual exists in the organization. That's their specialty. That's the value that they bring. It is understanding that we aren't like just the central node of intelligence and everything that goes on, but everyone's there for a reason and for their expertise.[00:18:00]
Chris Detzel: Have you ever thought about going back to the data governance specific Thing is i've been at companies where I mean you do whatever you want Yeah, you know what? I mean go kind of thing and to some degree, And maybe to michael's point some people need that and some people don't but I find it very difficult to Go into a company that has very rigorous data governance and other governance kind of Things that have been around for a long time, you know If you want to build something from ground up or do something that needs, you know a ticket here a ticket there a ticket, like How am I going to do this, and yeah, how do you say?
Okay, this person's coming in let them have access to certain things, like contacts or I don't know whatever, you know It's a character Think about some of that. And I guess nothing's perfect. It's just hard to make it good. Really good.
Alex Welch: You
Chris Detzel: know?
Alex Welch: Yeah, I think there's a few things to unpack there.
I think, yeah, one of them is ~ ~governance committees [00:19:00] and ~ ~that those organizations within broader enterprises, I think they oftentimes lack that review cycle where they say, why do we have this policy in place? Why is this existing? And if that is no longer the case, like for example going through the the whole migration into cloud, right?
There's a huge pushback from what I call legacy, industries like finance healthcare logistics saying our data is too valuable We can't give it to aws. We can't do it this way and therefore we're going to lock everything down and you also saw that similar thing happen with ai right? Like we're going to lock out everyone's access to chat gpt to claude And it's just, part of that is not quite understanding the technology and really understanding, the value and the security measures around it.
They need, you need to have that feedback cycle so that you are removing policies that are prohibitive. And I'm also a big believer You know at the stage in the company one of the things you need to do is put in policy You need put in governance, right? And so I was literally just talking to one of [00:20:00] our data engineers today And he's I feel like i'm always going process But it's a good thing as long as it meets two different criteria, right one is that it's not Introducing more friction than it needs.
Sometimes it needs friction. Sometimes it doesn't. The other is that it is being successful in offloading the mental load that people have, and it informs of about what and how they should be doing something. So I think those two components in conjunction with continually reevaluating your policies and why are really critical together that so that you don't over policy something, but you need it.
But you can't go over overboard.
Chris Detzel: I like that. That's good.
Michael Burke: And I totally agree with that. There's so many things. And Chris, I feel like in the example you bring up, you are in some ways the victim of somebody who's caught up in a, in bureaucratic policy. And that can be challenging at times, but at the same time, like to play devil's advocate, what if that policy didn't exist and you could just [00:21:00] send, whatever emails to millions of people or, delete some data on some table that, you weren't supposed to have access to.
I've been there, I've created systems where. That's happened. And, there might be 10 other departments that are running their business on that centralized data system that you built. And some, engineer came in, any of
Chris Detzel: the information I just need. Totally. But
Michael Burke: we live in such a complicated ecosystem nowadays where there's so many codependencies that even something as simple as reading or taking action on a piece of data.
Could have huge downstream effects that we're not aware of right? Yeah, because we are in silos inherently in larger ecosystems Whether we like to believe it or not I
Alex Welch: mean even older ones, right? You have that simple like data team which is a silo and then you have these other groups that are springing up So I mean exist in orgs of all sizes really
Michael Burke: so jumping back here, companies that are You know moving into this ai space and [00:22:00] trying to make sense of You know, A.
I. Catalyze shifts. I know a lot of C. I. O. S. And C. D. O. S. Now have huge budgets to invest into do A. I. What do you think the biggest hurdles are today in starting some of these projects?
Alex Welch: Yeah, I think I identified two hurdles. One and I'll rank them. One is data debt, tech debt.
The other is skills and culture gap. So going back to the debt perspective, right? It is one of those things that we Everyone knows exists. It impacts a ton of things, right? It can ultimately impact your model performance, your reliability. It can impact your implementation timelines and all of that kind of coalesces around, like impacting the ROI of your success, right?
And the thing about tech debt is it doesn't go away. It just can purely compounds over time. And it, it can break your lineage, create data silos. And if. You aren't conscientious about resolving that, or at least paying it down or restructuring it in some way. And your [00:23:00] AI depends on data assets that come out of that ecosystem.
You're going to find yourself, spending more time diving in, trying to understand why certain things are happening. Especially in an AI system. If you don't have the right outputs that explain why it made the decisions it made, right. You're just going to be like, why does all this exist?
Why is this two different versions of the same metric? So I think that's the number one thing that, we really have to take a look at when you're implementing a I. The other is, skills and culture, right? There's going to be resistance to change, and that's just a constant for everything, right?
And that can be fear of the new technology, not understanding it, not wanting your job to change. All these types of things are going to create this friction and resistance in the org. And then also just getting the right people with the right skills to do the things you want to do. So I think those are the, those would be the two big ones, I think.
Michael Burke: Yeah. I, and I think that the skills piece is so interesting because we've now moved to this world where everyone's no, [00:24:00] like we, you don't need as much skill. ~ ~And I think that is such a loaded assumption to make right walking into is Oh, no, we can give it to a large language model and you get results and you can use those results.
And it's Okay how do you interpret those results? Yes. Why do you think that a machine made those decisions? And how are you going to take that data? And what are you going to do with it? Those are all like pieces that If you haven't worked and analyzed and understood a lot of these technical components, it's really hard to get the right kind of value out of this.
And you could be driving things in a completely wrong direction, right?
Chris Detzel: Absolutely.
Michael Burke: Yeah. I can't even imagine right now what cohorting, how cohorting has changed, right? It's been a while since I've been in that space, but I'm sure it can be. The explainability gets a lot more complicated, right? Are you can you use a large language model to explain a cohort or do you need to do your traditional A B testing that you've had to do like for decades before, right?
~ ~Especially for companies getting into this, and I know that there's just like such a push. From every company that I've talked to about, like doing more [00:25:00] with AI. And I think that's great, but don't replace a highway in your ecosystem, right? Start with something really small because you are just going to get run over thinking that you're working with an expert when you're taking the wrong action still.
And then to me, like data quality. Is partly about having the right engineers and resources to be able to analyze information to understand what to do with it and we're yeah we're not we've missed that piece over the last year and a half and we think that we've replaced it but there's still so much more there that we're Assumptions that we're making right?
And you're starting to see these things backfire more and more which is funny as AI professionals and data professionals being like it's probably shouldn't have done that right there. Yeah, it can those three steps
Alex Welch: Yeah, I mean it goes back to your comments earlier about boiling the ocean, right?
If you're trying to bring in ai to solve all the big problem of give my users Unfettered access to insights off my data. It's probably not the right initiative that you want to start out with. It may be You know the real focus at least [00:26:00] my belief is and how i'm approaching it is to look at look for use cases where I can Augment my team you know help them identify data quality help them troubleshoot when problems happen You know, what other automations can I do to?
Hurry up their time so they can use it on most valuable aspect of their job, right? And eliminate that kind of tedious and waste in the system as it gets generated
Michael Burke: I love this and I think this is a really good segue into how do you see humans and A. I. Interacting with data management and analysis moving forward?
Let's scope it a little bit, right? Let's say in the next year, right? What are some of the changes and ways that people should really be adopting or leveraging these technologies, especially when we say I think we're talking about large language models. But correct me if I'm wrong here.
How do you see people using it?
Alex Welch: Yeah, I ~ ~think it's going to change things. Pretty dramatically. So if I had to draw a parallel, it might be This is a very, might be a terrible parallel, but like [00:27:00] to spreadsheets and how they didn't actually replace financial teams or accountants, but it completely transformed the role of the, what they do, the skill sets they need to actually do their job.
And it, Augmented their job. So like they didn't have to type in stuff manually in the calculator and write it down and reduced errors in the system. And maybe introduced a bunch more because we all know spreadsheet hell and how that works out. But if I had to draw that parallel, right? And so if you take that in and keep that in mind, there are like some core areas I think that will change.
And that's one of them is just this evolution of roles and responsibilities, right? And how that changes over time. For example, an analyst, right? If they're moving from having to troubleshoot a model or no longer having to do manual data processing there's a world where they spend 60 percent more time on strategic work.
So there's stuff like that. I, there's this division of labor from a cognition [00:28:00] standpoint. A. I. Is really great at pattern recognition and repetitive tasks like you can hand over your pattern detection or your basic analyses or report generation to A. I. Right? But then for humans, you reserve that brain power for strategic thinking, right?
What is the problem definition? How does this align to the broader strategy? And they all have their own weaknesses. But I think that's going to be another thing that, ~ ~really highlights the interplay between humans and AI. And then you start talking about like interaction models off of that, once you understand the strengths and weaknesses of both of them, and then you start defining how you want them to interact with each other and you can have.
I think you can bucket into two different areas. You have like decision support where it's like AI generates an opinion and then a human comes in and makes some choices, has a conversation and refines that and then you can have like knowledge enhancement where there's context that's given by the AI and then the human turns around and gives their expertise and that's more like continuous learning down the path.
Michael Burke: I love that. [00:29:00] Example of kind of a I being more proactive and providing a question, right? Or a solution and saying, What do you think? I totally agree that I think that's going to be a huge piece of this. It's Hey, you've got a thousand pieces of data. You need to review. Here's my guess on what it looks like.
Does this look roughly right to you? And I think that as we think of like, ~ ~how do you work between a junior or senior and a principled data professional, it's similar, right? Like the senior or principled data professional doesn't have time to actually review everything that a junior person does, maybe an intern they have to.
But, the. Say, Hey, what do you think about this? And you look at it and you eyeball what they're doing. You look at maybe a first a hundred records or something and say, yeah, this is the output we're thinking about here. Yeah.
Alex Welch: That's on the design. Yeah. I like that
Chris Detzel: thought.
So a couple things that you mentioned was, look, I think AI changes everything, but I, somebody asked me one day, do you think what advice would you give to a young person using AI in, in all this I mean that they're just going to be they're just going to have it ~ ~There's no advice I can give to them because they're just going to [00:30:00] be using it on a consistent basis It's more advice to 30 plus year olds that are worried about their jobs, You know before the internet we actually had jobs and had to do everything by paper But then we had to change the way we did things then it took years and years to do it all in a digital world moving, file cabinets into you know You Google drives and whatever, that's how it's going to change the world. Our jobs will change everybody's job will change. It's like just a year ago I needed a blog writer to write all of my blogs now I can take the transcript write a blog in three minutes Which used to take me a week or two and then I had to find an expert to clean up the blog You know what?
I don't Media something that took two weeks or so now it takes five minutes. Oh,
Alex Welch: yeah. Yeah, so Remarkable. Yeah,
Chris Detzel: so those jobs do go away ish, right? I'm, not saying that people can't have an eye and just clean it up make things better and I just think that it will ai changes everything [00:31:00] in a big way We don't know everything we know some ways with the clods and the chat gpts and things like that but Even more so it's going to be embedded into every single product.
It's going to just be you know for every single product company You know talks about they were doing ai everything, and that's just the thing But
Alex Welch: yeah,
Chris Detzel: Three years from now. It's I mean you're not doing it. I don't really get that
Alex Welch: Yeah, I don't see that. I think if 30 something year old, right?
Yeah, I think it would be two products. One would be You and they play off of each other, but the big one is just looking for ways to infuse AI in your life now and in small ways and in doing so you learn how you prefer to interact with it. If you, for example, I used to spend forever meal planning for my family, right?
My wife, two kids for the week, and it would take forever. And then you have to place the order. And now I have a little system with a prompt that I've been playing with locally that goes through. It takes all of our preferences and it [00:32:00] says, okay, here's four meals. Here's the recipes. And I'm like, it needs to be under 30 minutes to cook and needs to use common ingredients, and they say meet these like nutritional restraint, like constraints boom. And I'm like, great. It asks for my confirmation. If I want to switch something out or if I want to do anything else with it, once I say, okay, it creates that shopping list and then it ships it into an Instacart for me to review the order.
And then done right now.
Michael Burke: Are you starting a company? This is awesome.
Alex Welch: This is me getting sick of doing things ~ ~but really like it's stuff like that where you're like What why am I spending so much time doing something or like? I saw on LinkedIn, Allie Miller, if you guys are familiar with her, she did one where she set it up so she could take a picture of her fridge and it will tell her what snacks are available, right?
It can be little stuff like that, but as you do that more, it becomes more of a muscle that you build and then you start learning, okay, where are the borders where I'm going to bump up against? How do I prefer to interact with AI systems across different modes and whatnot.
And then as [00:33:00] AI continues to evolve and be implemented in organizations, you're going to already going to have that muscle to one, identify where it can be strategically helpful. You're going to have familiarity with the tools, so you're gonna have better adoption of those. And your skills will be there.
You don't need to know linear algebra and how all this works To get the more value out of AI, you do need to know how to use it. And so by just challenging yourself now for all the little stuff during the day of what you can use AI for, it's going to build that muscle so that when it times comes, you're going to be in a great position to take advantage of it.
Michael Burke: Totally, that's all I would also add to this and I love your examples. That was amazing, by the way. And I think we might need to do another episode on this fridge app. You've built. That sounds incredible.
Chris Detzel: I knew Mike would be excited about that when you were saying that. Yeah, a couple episodes. With
Michael Burke: a
Alex Welch: newborn, right?
Yeah,
Michael Burke: I won't. Yeah, I won't deviate too much, but I've definitely done some fun little projects there. ~ ~I think the other big thing that we do not give [00:34:00] ourselves credit about is the originality of us as individuals. And one thing that I've recommended to everybody who I'm saying, use chat GPT, right? Use cloud, try these models out, play with them, ask questions, don't ask for solutions.
And the reason being is like, You as a decision maker need to continue to work that muscle. And if you're writing an email and you need it, you want it like feedback on how to write it better. I wouldn't take what chat GPT gives you. I'd say, Hey, can you give me some suggestions on what I could do better?
Yeah. Take that into your ecosystem and learn from it. Because personally believe that we are starting to develop the early signs of reliance on AI. And I think that's. Going to happen. I think the more that we teach ourselves to be stronger and smarter and better human beings will make us better at using AI and it will improve our own quality.
Like we shouldn't need to use AI to write an email after we've learned how to write that email better, and we can, sure there's a nuance there, but like [00:35:00] even with what you're eating every day, give me a list of three options. Don't tell me what i'm eating for the day. Oh, keep the choice within the person because I think that will inherently make you stronger and more unique as an individual Like you have a voice you have a you have amazing thoughts Like use those to your advantage within these systems don't rely on the standard output that it's going to give you
Alex Welch: yes, and I think you can even take it. You've got to look
Michael Burke: there.
Alex Welch: No ~ ~Further than that because I definitely do that i'll be like, okay give me Five examples of this or five ideas here and i'll pick from it The other thing that I do at the start of my prompts is like ask questions along the way, right?
And so I love doing that and so that it oftentimes gives me a different venue to get a different perspective on what I'm asking, right? I'll be like, Oh, what about this? Or what about that? And then sometimes like in the, if I'm writing like a document or a proposal for something, I'll throw it in and I'll be like, okay, go through here and challenge some of these assumptions.
Why are you challenging [00:36:00] them or find places in here where it's, Vague or whatever and help me craft a better narrative and give me a couple options, right? And Doing that, again, it goes back to augmenting, I still have my expertise that I'm imparting on the system But i'm using that to speed up.
The kind of creation process and the other way I look at it too is you know, ~a~ lot of Ideas like if you go back to college and you're sitting in your dorm room at 3 a. m With like your roommate and you guys are solving all the problems in the world, right? Yeah, we're gonna be awesome but and the world's gonna be awesome those ideas generated because you had that back and forth.
You had that conversation going and you were able to ask questions about clarifying questions. Oh, that's cool idea and pull these and you can treat like these LLM is the same way for like challenge assumptions. And I think that's the important part. You gotta be willing to challenge your own assumptions and your own learnings.
Because if you're not you're just going to get that same output every time but you can use it as such a powerful like Context and decision making tool
Michael Burke: [00:37:00] totally and the reason it got me I'm going off on a tangent here as I was watching this video the other day. I have some A woman at school in college and a pop quiz came up on the screen She took a picture of it flipped it to her laptop and solved every problem with photomath or some other tool like that in 15 seconds and i'm like We are missing the point of leveraging AI to make us better at that point.
And we're starting to become dependent on its solutions. And this, I know this goes on now with you, if you've been interviewing junior engineers, you see it all the time. Like reasoning skills are depleting, like we're losing those skills. And it's it is so important that we keep them. And I think for us as a generation that didn't have this, we have a lot of that, and we're having these conversations, but the younger generations, they're, you They're just dependent on this technology, like nothing we've ever seen before.
And it's worrisome for me that we, a great example of something that's doing an amazing job is like a con Mego. I don't know if Khan Academy, they haven't, they have an AI that's, we'll only respond in Socratic methods. So you are trying to solve a math [00:38:00] problem and say, okay what steps do you know?
And it'll walk you through it as a teacher would like, we need to be, I'm leveraging
Alex Welch: now. That's awesome.
Michael Burke: It's an amazing tool Like I think it's one of the best use cases of a large language model out there today but we need to be we need to be asking questions And brainstorming not just relying on solutions, right?
Alex Welch: There's also a book out there that I you know, i'm not affiliated with in any way, but it's let's hear despite matt being what's called the skill code ~ ~And it's a book about how exactly what you're talking about, right? Is, these younger generation coming through and not getting the touch of a master apprentice type transference of knowledge and expertise there.
And, when you bring up the, the girl taking the picture, you're like, you're not learning anything from there. You're expediting the solution, but you're not actually, Doing what the purpose of that quiz was for, right? You're circumventing it. And I think, in the book, it really hammers the point of the more we abstract and make it easier for [00:39:00] experts to do things, the wider that gap of, mentorship becomes, and it's going to continue to have adverse effects on the skills that come through it.
And I think going back to advice for young people, it is really going out of your way to. Find somebody and work with somebody to mentor you on the expertise so that you can then take those expertise and then in addition to your knowledge about the systems, enhance it. And I think that will put your career on a trajectory, right?
Michael Burke: Couldn't agree more. Yeah, absolutely.
Chris Detzel: So there's a question we haven't asked yet, and it goes well with what we're just talking about, like new skills and roles. What do you think about when you think of the future for data organizations? What's going to be crucial in this gen AI world?
Alex Welch: Oh, man.
So there are roles, I think that will exist, right? And I'll go into those a little bit, but then there's also these changes to just the organizational alignment. And I think it's going to have really interesting impacts over there, but like from a roles perspective we're going to [00:40:00] start continuously prompt engineering specialists that are in there and like fine tuning the prompts that are used for these systems people solely dedicated to.
I'm calling them data quality orchestrators, not just like analysts, right? That are specialists in designing validation frameworks. They are specialists in optimizing these platforms for AI specifically, right? You're gonna have governance roles that pop up, right? And these are all core foundational roles.
But then you get into more strategic roles like AI ethics officers innovation strategists that are focused on AI. And then the last piece is operational roles. So things like, I think I find this one. Somebody mentioned this to me a while ago. I found it really interesting is a human AI interaction designer, right?
Somebody who is actually designing UX UI systems has experience in human psychology. And it's actually building all this into optimize a human experience there. And, Really pull that out. And I thought that one was really interesting as well.
Michael Burke: Yeah, totally. It's there's so [00:41:00] many roles that I think are going to be, you're going to specialize in, right?
Like embeddings, I think there's going to be a whole, there already is, people that focus on this, but it was very niche. Prior, embedding specialists are going to be huge. I know people with PhDs in data science that this is what they're doing now. And I'm like, really? And he's yeah, it's a pretty easy job.
I just manage vector encodings and embeddings and ship them. That's my role. ~ ~And also I think that you'll see a big ~ ~change in the amount of true AI specialist versus these like sub roles within AI. Where it's more of like a certificate program or like an associate's degree of going through and learning how to work as a operator of AI, right?
The same way in manufacturing, you've got people that design systems and then you have people that operate systems.
Alex Welch: Yeah very true. I you saw that a little bit in data science, right? Yeah, and that whole way that came through. ~ ~Absolutely. But I do think it's gonna be really interesting.
There's one. ~ ~I do think that we're gonna start moving away from this hierarchical structure a little bit into more than like [00:42:00] traditional teams where you have analytics teams with very fixed roles, isolated tax for engineering teams, and then you move into more of a dynamic pod structure where you have scientists and engineers and domain experts all together.
And I think that's gonna start happening because it's You have these when you infuse AI into that, you can speed up decision. You can speed up response time. You can iterate much faster and you can solve these problems and then hand them off to the, what you were describing, right? The operators, and then you can disband and move and kind of position.
Spotify has a model right now where they like tribes and groups and things like that. But, I do think having. We'll start seeing these specialist pods that help solve problems. With the verticals with the business domains and then you have a simpler transition effort that happens to the operators and then And maintainers and then you disband and create different ones across the org as you devise value
Chris Detzel: So quickly [00:43:00] and this would be our last question.
This has been very good so far. Thank you Again, this has been great. So when you think of emerging trends technologies You know in the ai and data space, what should organizations prepare for and think about?
Alex Welch: What should they? You should be thinking about human AI like collaborative teams between human and I and what those interactions look like Right and it like how you treat a eyes a team member and like a junior, right?
You could you should be thinking about what is your Decision intelligence network look like across, what are you, what is the impact it's having on learnings on the velocity of your decisions on the capacity, right? That's another thing. How you can use AI to, automate your cognition process of your workflow, right?
Taking knowledge work and automating that taking some complex decision automation and creative task assignment for that perspective. And ~ ~yeah, I think. Operations is probably going to be an area where ai [00:44:00] really ticks off as well so prepare what your ops teams and Those types of functions are going to look like in addition when you have ai to augment it and what you're going to do with extra capacity the answer is probably not just cut work first because we get all this whatever that we get all this extra capacity and efficiency.
It should be okay. We have this extra capacity to do it ~ ~Train mentor to bring up new skill sets to get that next generation and play to succession plan. We have this to actually take the experts and put them in those invited pods as domain experts to help craft and fine tune these models in trying to figure out what you're going to do with the extra.
Efficiency and capacity that you get from actually implementing these things.
Michael Burke: Alex, what do you think about the human side? I think that large language models and AI right now we focus. They're very focused in silos, right? There's specific tasks. But as we get to multi models and more even centralized models, I would say within an ecosystem, ~ ~do you think that they're going to be working [00:45:00] more?
With things like, and this is, again, this is much more in the future. I would say not in the next year, but probably in the next five or 10 years, even, but being able to see, inefficiencies or synergies between business units, or maybe between even people and personalities, like we are going to be putting so much data and so many feelings and our communication styles into these systems.
There might be ways that it could even see across these different borders differently than. And I just I'm thinking back to the water coolers when I used to go into an office of Oh, you know i'm having trouble with this employee or like i'm not getting what I need from this manager Yeah, and like the only time that got escalated was like if I had to report something to hr, right?
Or there was some big to do but otherwise like there might come a time where we're actually giving a lot of this information to an AI, right? And if it's centralized, can it see across those systems? Do you think
Alex Welch: That's up to your org, right? Are you gonna allow it across the systems?
Is that the first one? Yeah, I think one One, you know at dbt we have an AI [00:46:00] function where we're really focusing and like rolling out a lot of functionality for like Auto document generation, code generation conversions to our semantic layer. Also it's cool stuff like that. But as we talk about, what does the future look like with AI?
There are worlds where, I'm a, I could be an analytics engineer, I could get on in the morning. Oh, there was an error. I pull up the AI system. It helps me diagnose exactly what's wrong with the error. I confirm that's. Is in fact, not a false positive. And then it takes all that gets the business contact and sends it off to the business owner, like the marketing.
And says, Hey, there's an issue. This is what's happened. Here's how we're running is, or, Hey, we saw a step change in the, the revenue numbers and it popped up. ~ ~Let's package that. Let's send it off to somebody to actually put their own expertise on it. And route those things. So then all of a sudden you're getting an interact a proactive interaction Just the watercolor was like, oh, I saw this cool thing What do you think and then it can [00:47:00] facilitate that knowledge dissemination as well?
Michael Burke: I love that alignment right like instant alignment and in detzel's case instantly filing tickets right with 12 different organizations at the same time Exactly. I think that it you know It's interesting, but like that idea of even a ticketing system may change completely in the future.
And that kind of internal approval process, it governance. I think we're a long way away from it, but that's something that excites me. It's instantaneous water cooler conversations like and resolutions. Yeah in one swoop I
Chris Detzel: mean, it feels like it could you could build such a system that tracks, When you do trades and all these other things that says, you know What you're making a bad decision go with this and then everybody gets rich because it knows everything No,
Alex Welch: I you know, I think there's can you imagine a system that You know, under takes the request and understands the questions the business is asking and then actually creates and breaks down those into tasks and then auto prioritizes them based [00:48:00] on the business strategy and just assigns those out or gives more context to the analyst or coordinates certain meetings coming together because it needs this level of expertise from here and here.
Just like streamlining that whole automation. That's. It is a, it could be a reality in, five years or something, or tomorrow based on our trajectory, wild,
Chris Detzel: what about like code that breaks all the time because it was just bad code, right? Just implementing this AI system that looks at all your code and says, clean this up, push the button to do it.
It's clean, because you have a lot of. Coders that leave and say, bring in other coders. And I feel like that's a huge opportunity.
Alex Welch: That's, yeah, that's the, when I'm talking about, when I was talking about, how I'm thinking about it and finding like those use cases that augment the team.
That's a, that is a use case where we're like, okay can you look at the DAG and say, okay, this is where your bottleneck inefficiency, or you, this is a critical point in your DAG and you need to [00:49:00] do something special with it. And. Not only this is it, but explain why it is, and then write the code for me.
Yeah, give me, write the code and give me a recommendation and like on potential other paths forward, right? And then it can open a PR request for you and actually say boom. And then you can actually work with it there and speed up that whole development process.
Chris Detzel: Yeah, so many opportunities, I think, with AI and how we embed it into these new products and, the skills needed.
And Alex, this was awesome, yeah, we nerded out just like you promised. So
Alex Welch: always looking forward to it. Any chance I get really, did
Chris Detzel: we miss anything that you think we should have covered? I think
Alex Welch: the only other thing I would call out is, we talked about frameworks, governance, and it touched a little bit on security frameworks and whatnot, but it is, being aware of, this whole world of bad actors is emerging now in the AI space. And so not only is your engineering and [00:50:00] your data team, not only are they going to have to evolve, but your security teams will as well.
And they're going to have to, we're going to have to put in place Checks and balances to prevent against some of these bad actors that, can figure out, you've already seen it. They can circumvent some of the, like the controls that I think it was Claude had where they circumvented it and actually got the stuff behind the pay wall, in this, at the more powerful AI becomes, the more that people are going to try and exploit it and push it.
And this is a whole org change right top to bottom Everyone needs to change the way they think and they adopt it if you're going to be extremely successful in five years And so just don't neglect the areas that are necessarily like super obvious at first
Chris Detzel: ~ ~Thank you everyone for tuning in to another data hurdles Please rate and review us or make alex right and review us as well. But i'm chris detzel and
Michael Burke: i'm michael burke Thanks for tuning in
Chris Detzel: Thanks everyone.
Michael Burke: Thank you guys ~ ~
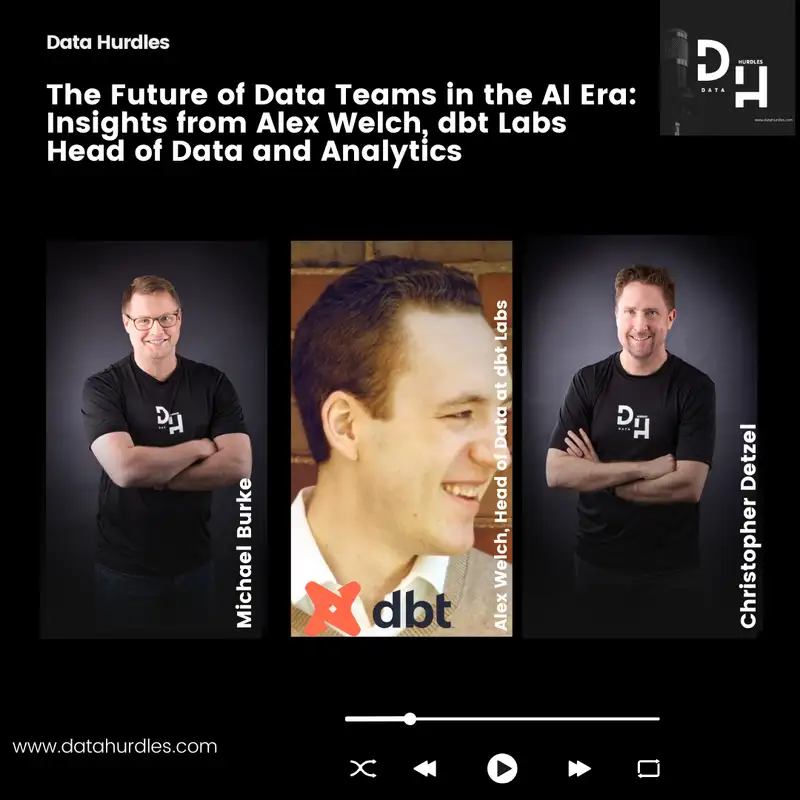